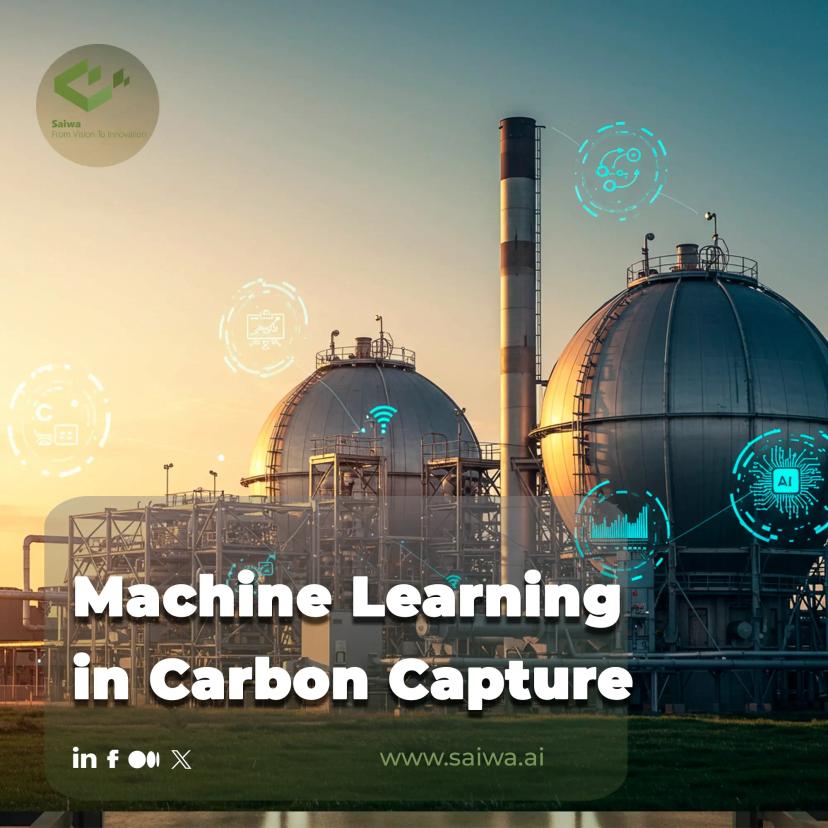
Machine Learning in Carbon Capture | Advancing Climate Solutions
The escalating concentration of atmospheric carbon dioxide (CO2), primarily attributed to anthropogenic activities, has spurred significant global concern regarding climate change and its associated environmental repercussions. Mitigating the adverse effects of climate change necessitates a multifaceted approach, encompassing both reducing CO2 emissions and actively removing existing CO2 from the atmosphere.
Carbon capture, utilization, and storage (CCUS) technologies have emerged as a crucial component of this strategy, offering a promising pathway towards achieving net-zero emissions. Machine learning (ML), a subfield of artificial intelligence, has demonstrated remarkable potential in enhancing various aspects of CCUS, from optimizing capture processes to accelerating materials discovery.
Saiwa is an AI-driven company that provides a service-oriented platform for advanced artificial intelligence (AI) and machine learning (ML) solutions. Through its innovative technologies, Saiwa helps companies improve efficiency, solve complex challenges, and achieve sustainable growth with customized AI and ML services.
This article explores the intersection of machine learning and carbon capture, examining the diverse applications, key techniques, associated benefits and challenges, and future trends in this rapidly evolving field.
Understanding Carbon Capture
Carbon capture encompasses a suite of technologies designed to capture CO2 emissions from industrial sources, such as power plants and cement factories, before they enter the atmosphere. These captured emissions can then be either utilized for various industrial processes or safely stored underground, effectively preventing their contribution to global warming.
Several carbon capture methods exist, including absorption, adsorption, membrane separation, and cryogenic distillation, each with its own set of advantages and disadvantages.
The Role of Machine Learning in Carbon Capture
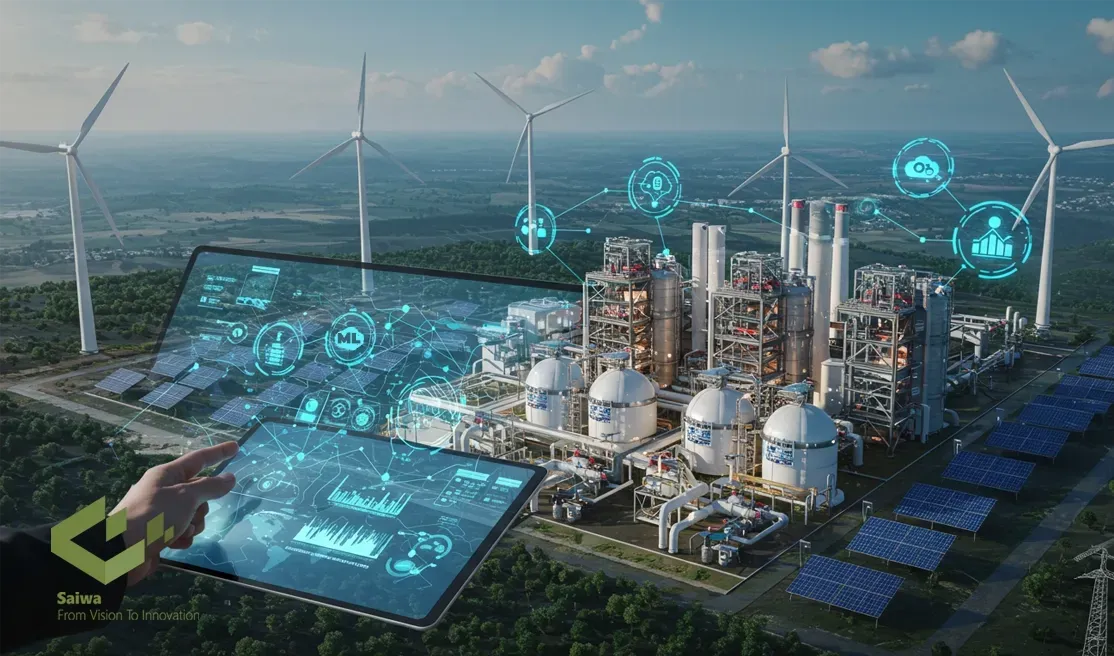
Machine learning plays a pivotal role in optimizing and enhancing various stages of the carbon capture process. By leveraging its ability to learn complex patterns and relationships from data, ML algorithms can improve the efficiency, cost-effectiveness, and overall performance of carbon capture technologies. ML can be applied to optimize process parameters, predict capture performance, accelerate materials discovery, and enhance monitoring and control systems.
Applications of Machine Learning in Carbon Capture
Machine learning finds applications across diverse carbon capture methods, tailoring its approach to the specific characteristics and challenges of each technology. Its ability to analyze complex data and identify patterns makes it a valuable tool for optimizing and enhancing various aspects of carbon capture processes.
Absorption-Based Carbon Capture
In absorption-based carbon capture, CO2 is absorbed by a liquid solvent, typically an amine solution. ML can be used to optimize solvent selection, predict absorption rates, and control process parameters such as temperature and pressure, leading to enhanced capture efficiency and reduced energy consumption. ML models can predict solvent performance under different operating conditions, allowing for the selection of the most efficient solvent for a given application. Furthermore, ML can optimize the design and operation of absorption columns, minimizing solvent usage and energy requirements.
Adsorption-Based Carbon Capture
Adsorption-based carbon capture utilizes solid adsorbents to capture CO2 from flue gas streams. ML can assist in designing novel adsorbent materials with improved CO2 selectivity and capacity, as well as optimizing adsorption process parameters for enhanced performance.
ML models can predict the adsorption behavior of different materials, guiding the development of high-performance adsorbents. Moreover, ML can optimize the regeneration process of adsorbents, minimizing energy consumption and improving the overall efficiency of the capture process.
Membrane and Hybrid Technologies
Membrane separation technologies utilize selective membranes to separate CO2 from other gases. ML can be employed to predict membrane performance, optimize membrane design, and control operating conditions for enhanced CO2 separation efficiency.
Hybrid technologies, which combine different capture methods, can also benefit from ML-driven optimization and control. ML can predict the permeability and selectivity of different membrane materials, aiding in the design of optimal membrane configurations. Furthermore, ML can optimize the integration of different capture methods in hybrid systems, maximizing overall capture efficiency and minimizing energy consumption.
CO2 Storage and Utilization
Once captured, CO2 can be either stored underground in geological formations or utilized for various industrial processes, such as enhanced oil recovery or the production of chemicals and fuels. ML can contribute to site selection for CO2 storage, predict storage capacity and integrity, and optimize CO2 utilization pathways.
ML models can analyze geological data to identify suitable storage sites and predict the long-term stability and safety of CO2 storage. Furthermore, ML can optimize the conversion of CO2 into valuable products, maximizing resource utilization and minimizing waste.
Key Machine Learning Techniques for Carbon Capture
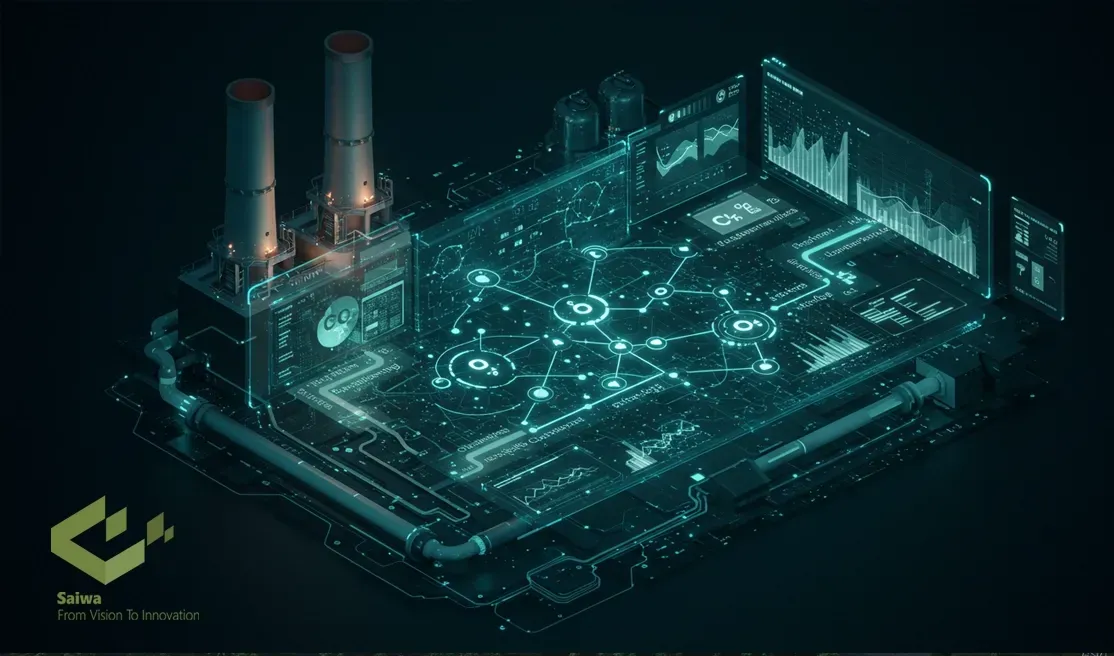
Several machine learning techniques are employed in carbon capture applications, each offering unique capabilities for addressing specific challenges. These techniques range from supervised learning for prediction and optimization to unsupervised learning for pattern discovery and anomaly detection.
Supervised Learning
Supervised learning algorithms, such as support vector machines and random forests, are used to predict CO2 capture performance based on historical data, optimize process parameters, and classify different CO2 sources.
By learning from labeled datasets, supervised learning models can establish relationships between input features and target variables, enabling accurate predictions and informed decision-making in carbon capture operations.
Unsupervised Learning
Unsupervised learning algorithms, such as k-means clustering and principal component analysis, are used to identify patterns and relationships within carbon capture data, discover optimal operating regimes, and detect anomalies in process behavior. Without relying on labeled data, unsupervised learning algorithms can uncover hidden structures and insights within complex datasets, providing valuable information for process optimization and monitoring.
Neural Networks
Neural networks, including deep learning models, are used to model complex relationships between process parameters and CO2 capture performance, predict capture efficiency, and design novel adsorbent materials.
Inspired by the structure of the human brain, neural networks can learn complex non-linear relationships from data, enabling accurate predictions and sophisticated modeling of carbon capture processes.
Deep Learning
Deep learning, a specialized form of neural networks, is particularly effective for analyzing large and complex datasets, enabling more accurate predictions and enhanced performance optimization in carbon capture applications.
With multiple layers of interconnected nodes, deep learning models can extract hierarchical features from data, capturing intricate patterns and dependencies that are difficult for traditional machine learning models to discern.
Reinforcement Learning
Reinforcement learning algorithms are used to optimize control strategies for carbon capture processes, learning optimal policies through trial and error interactions with simulated or real-world environments.
By learning from feedback and rewards, reinforcement learning agents can discover optimal control strategies that maximize capture efficiency and minimize energy consumption.
Benefits and Challenges
Integrating machine learning into carbon capture offers numerous advantages while presenting certain challenges. Successfully leveraging ML for carbon capture requires careful consideration of both its potential benefits and the limitations that need to be addressed.
Advantages of ML in carbon capture
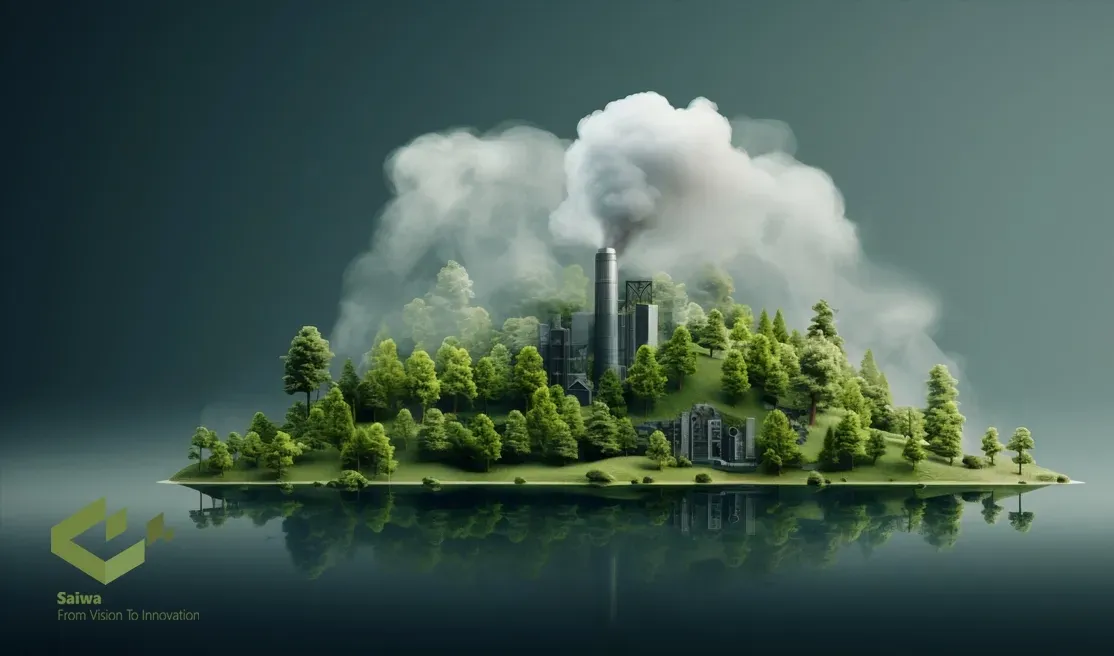
Cost reduction
ML can optimize process parameters and improve efficiency, leading to significant cost reductions in carbon capture operations. By optimizing energy consumption, solvent usage, and material requirements, ML can contribute to making carbon capture more economically viable. This cost reduction is crucial for the wider adoption and deployment of carbon capture technologies.
Improved prediction accuracy
ML algorithms can accurately predict CO2 capture performance, enabling better decision-making and resource allocation. Accurate predictions of capture rates, energy requirements, and potential equipment failures allow for proactive maintenance and optimization of the capture process, leading to improved efficiency, reduced downtime, and ultimately, lower operational costs.
Accelerated R&D processes
ML can accelerate the discovery and development of novel materials and processes for carbon capture, shortening the time to market for new technologies. By automating the screening and evaluation of potential materials and process configurations, ML can significantly reduce the time and resources required for research and development, enabling faster deployment of advanced carbon capture technologies and accelerating the transition to a low-carbon future.
Challenges and limitations
Model generalizability across different scenarios
Ensuring that ML models trained on one dataset can effectively generalize to other carbon capture scenarios and operating conditions remains a critical challenge. Variations in flue gas composition, temperature, pressure, and other factors can significantly impact model performance.
Careful consideration of data representation, model validation, and continuous model retraining are essential to ensure robust and reliable performance across different operating conditions and maintain the effectiveness of ML models in real-world applications.
Need for interdisciplinary expertise
Developing and deploying ML solutions for carbon capture requires expertise in both machine learning and carbon capture technologies, necessitating interdisciplinary collaboration between data scientists, engineers, and domain experts. Effective communication and knowledge sharing between these disciplines are crucial for successful implementation of ML solutions in real-world carbon capture applications.
Balancing computational efficiency with accuracy
Complex ML models, particularly deep learning models, can be computationally intensive, requiring significant computational resources and energy. Deploying ML models in real-time carbon capture operations requires careful consideration of the trade-off between model accuracy and computational efficiency.
Efficient algorithms, optimized hardware, and edge computing techniques are essential to ensure timely predictions and control actions while minimizing the computational overhead and energy consumption associated with ML operations.
Future Trends and Opportunities
The future of machine learning in carbon capture holds immense potential for further advancements and innovation.
The potential of generative ML for material discovery
Generative ML algorithms, such as variational autoencoders and generative adversarial networks, can revolutionize materials science within the context of carbon capture. These powerful tools can be employed to design novel materials with tailored properties for enhanced CO2 capture, such as high surface area, strong affinity for CO2, and high selectivity.
By generating and evaluating vast libraries of potential materials in silico, generative ML can accelerate the discovery of new materials with superior performance, significantly reducing the time and cost associated with traditional experimental approaches.
Integration of ML with Industry 5.0 for smart carbon capture systems
The integration of ML with the principles of Industry 5.0, which emphasizes human-centricity, sustainability, and resilience, will pave the way for the development of truly "smart" carbon capture systems.
These advanced systems will leverage ML to optimize performance in real-time, adapting to changing operating conditions, such as fluctuations in flue gas composition or energy prices, and maximizing capture efficiency while minimizing environmental impact. This dynamic and adaptable approach will be crucial for ensuring the long-term sustainability and economic viability of carbon capture technologies.
Opportunities in hybrid and modular carbon capture systems
ML can play a vital role in optimizing the design and operation of hybrid and modular carbon capture systems, enabling more flexible and adaptable solutions for diverse industrial applications. By integrating different capture methods, such as adsorption, absorption, and membrane separation, into a single, modular system, hybrid approaches can achieve higher capture rates and lower energy consumption.
ML can facilitate the design and control of these complex systems, optimizing the integration of different components, maximizing overall system performance, and ensuring seamless operation in various industrial settings.
Conclusion
Machine learning is poised to play a pivotal role in advancing carbon capture technologies, enabling more efficient, cost-effective, and sustainable solutions to mitigate climate change. By leveraging the power of AI to optimize processes, accelerate materials discovery, and enhance operational efficiency, we can unlock the full potential of carbon capture and pave the way for a more sustainable future.
As research and development in both machine learning and carbon capture continue to progress, we can expect to see even more sophisticated and integrated solutions. The future of carbon capture lies in harnessing the power of data and AI to develop innovative technologies that can effectively and efficiently remove CO2 from the atmosphere, contributing to a more sustainable and climate-resilient future.