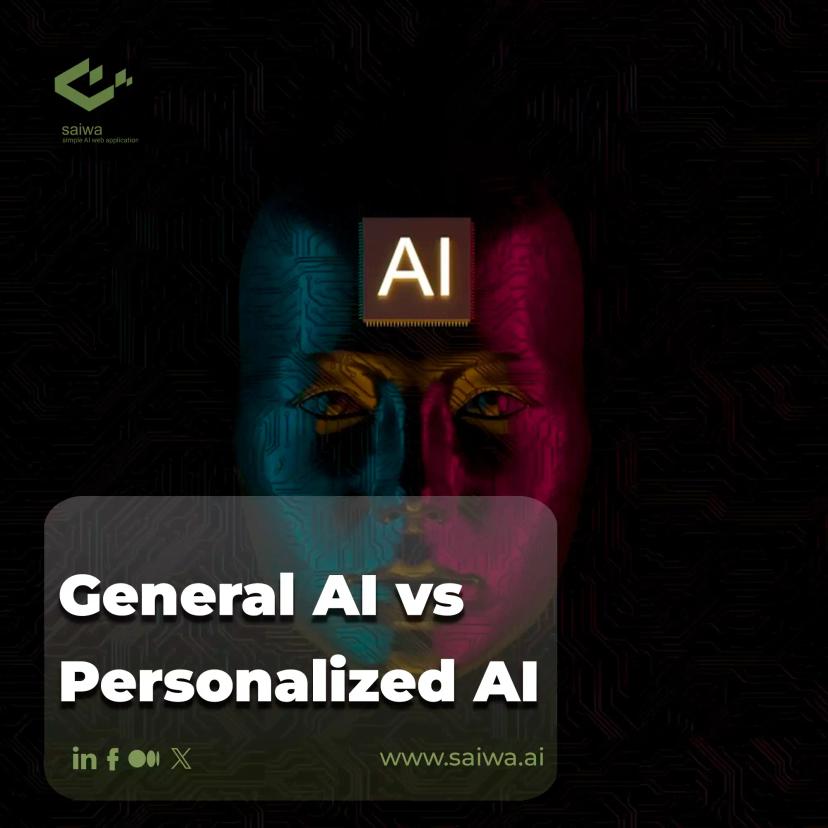
General AI vs Personalized AI
The rapid evolution of artificial intelligence (AI) capabilities in recent years has sparked a growing interest in not just developing generally intelligent systems but specifically personalizing them to individual users. While general AI aims for human-level mastery across cognitive tasks, personalized AI adapts models to users' unique needs and behaviors. This article compares the capabilities, challenges, use cases, and overall potential of general AI Vs Personalized AI to guide the responsible development of next-generation AI.
Understanding General Artificial Intelligence
General AI, also referred to as artificial general intelligence or AGI is a hypothetical form of artificial intelligence that aims to mimic the broad cognitive abilities associated with the human mind. This differs from current narrow AIs that focus on excelling at specialized tasks like playing chess or transcribing speech. The goal of general AI is to handle the ambiguity of open-ended, real-world situations by applying generalizable intelligence comparable to humans.
This requires a combination of versatile learning algorithms, extensive training data representing the complexity of the world, reasoning abilities to make connections and deductions, creativity to solve novel problems, and natural language skills to explain conclusions and interact. While general AI does not yet exist, researchers are exploring how to best simulate these interconnected aspects of human intelligence within a computerized system.
Significant challenges include encoding sufficient knowledge about how the world works, transferring learning between different domains, adapting to unfamiliar situations beyond the system's training, and balancing all capabilities to interact smoothly with how biological intelligences operate intuitively.
Read Also: What is Artificial Intelligence as a Service?
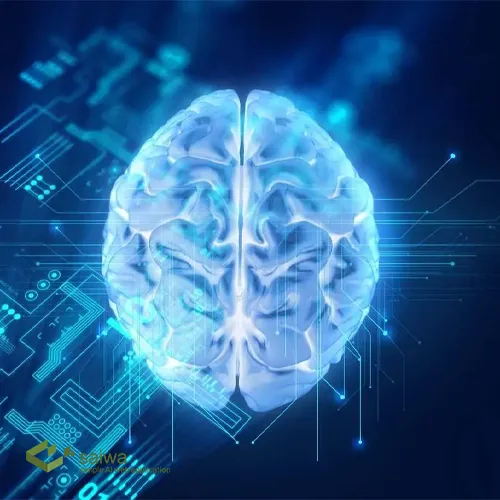
Features of Current General AI Systems
Contemporary general AI systems possess some signature features:
They aim to develop intelligence with a broad capability spanning different cognitive domains and task categories, from perception to reasoning and planning. This is in contrast to narrow AI focused on specialized tasks.
They do not require much customization or personalization for individual users. The focus is on establishing domain-independent intelligence.
They utilize principles of reasoning like search, logic, and knowledge representation to make deductions across contexts. Machine learning also aids statistical learning from data patterns.
They are often trained on large, aggregated datasets encompassing diverse scenarios beyond individual users' contexts. This aids generalization.
Evaluation involves assessing performance on standardized benchmarks and contests spanning different challenges. Real-world applicability is also important.
What is Personalized AI?
In contrast to pursuing artificial general intelligence, personalized AI systems aim to tailor responses and behaviors specifically to individual users by modeling their unique patterns, preferences, context, and feedback. Techniques like machine learning analyze data points collected about a person over time to build a customized profile reflecting their interests, habits, voice, vocabulary, and goals.
The AI can then continuously update its linguistic styles, recommendations, visual interfaces, and functionality to align optimally with each user. Rather than a one-size-fits-all experience, personalized AI provides tailored interactions optimized for the needs of specific customer segments or individuals. As people's needs evolve, the system adapts through ongoing use. Personalized AI is popular for applications like customized content curation, digital assistants, education technology, and marketing. These contained domains with well-defined user goals differ from general AI's objective of open-ended cognitive versatility resembling human intelligence.
Features of Personalized AI
Here are some features of Personalized AI:
Developing computational models reflecting each user through an analysis of individual data - Personalized AI systems collate extensive data about each user, including their demographics, preferences, usage history, behaviors, and feedback. This allows for constructing personalized profiles to tailor experiences predictively.
Contextual adaptation at runtime based on personalized preferences models - The system identifies the context like location, time, activity, and social setting to adapt its interactions accordingly in real-time based on models of individual users. This context awareness enables more relevant responses tailored to the exact situation.
Responding predictively to users based on learned individual habits and dispositions - Based on patterns recognized in user data, the system can proactively provide recommendations and information aligned to inferred preferences before the user even asks. This creates more intuitive interactions.
Improving through ongoing user interactions and preference feedback loops - The more people use a personalized AI system, the more data it gathers to refine tailored responses incrementally. User feedback also improves the models. The system essentially trains itself to align better with expectations.
Focusing exclusively on a specialized user-centric utility function rather than general cognitive tasks - Personalized AI focuses on maximizing a narrow utility related to serving individual user goals rather than pursuing general intelligence. The utility function optimizes for metrics like engagement, satisfaction, or sales.
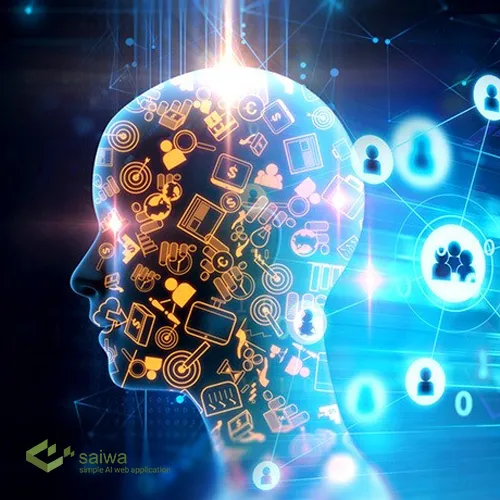
Key Differences Between General AI vs Personalized AI
Some central distinctions between the paradigms of general AI Vs personalized AI are:
Objective:
General AI aims to develop systems with human-level capability across cognitive domains like vision, language, and reasoning. The goal is task versatility.
Personalized AI focuses on adaptation to individual users. The goal is customization to users' needs, preferences, and contexts.
Scope:
General AI seeks to build AI with broad, domain-general capabilities spanning different tasks and environments.
Personalized AI provides focused, domain-specific customization tailored to individual user journeys.
Flexibility:
General AI systems exhibit pre-defined capabilities based on available training data. They display limited autonomy.
Personalized AI adapts its behavior over time based on user interactions using techniques like reinforcement learning.
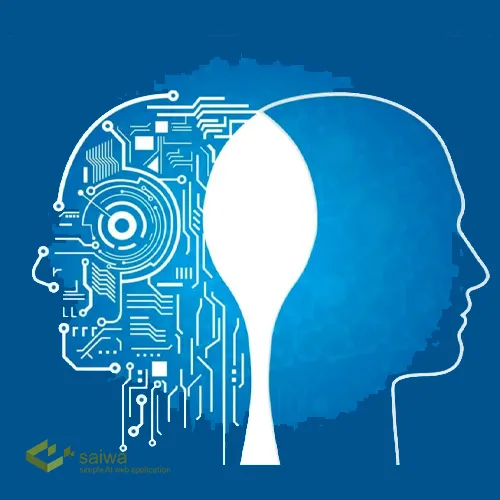
Implementation:
General AI systems utilize shared, reusable models trained on aggregated population datasets.
Personalized AI may leverage more individual user data to tailor models to their needs subject to ethical data handling.
Applications:
General AI enables applications like household robots, self-driving vehicles, and game-playing algorithms where flexibility is needed.
Personalized AI powers applications like recommendation systems, customized healthcare, and adaptive tutoring where individual tailoring is essential.
Conclusion
While general AI vs personalized AI have different objectives, strengths, and applications, their complementary capabilities can positively shape the future of human-centered AI. Blending generalization with personalization, balancing autonomy with oversight, and embedding transparency with ethics could enable AI to enhance human potential, equity, and well-being across communities. The path forward involves collaborative research toward responsible, trustworthy AI development that respects diversity and centers on human values.