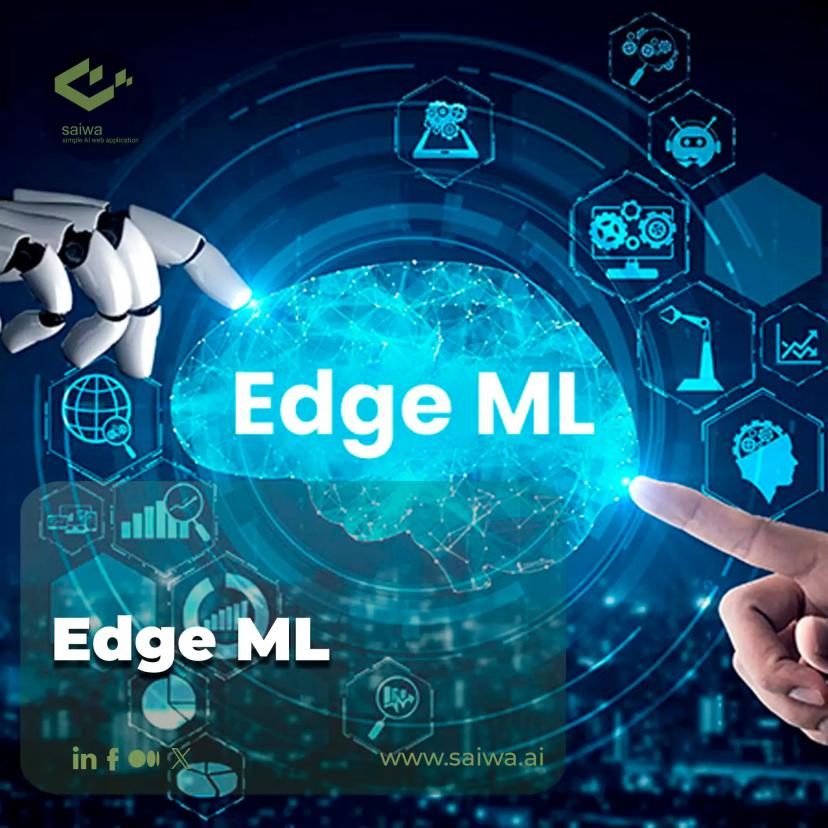
Revolutionize Your AI | Unleash the Power of Edge ML!
The rapid rise of smart devices and the Internet of Things (IoT) is paving the way for new computing technologies. Edge ML, also known as edge machine learning, is a revolutionary technology that allows devices to analyze data locally, minimizing dependence on cloud networks. Edge ML enables activities such as image recognition, natural language processing, and anomaly detection to be performed autonomously at the device or local level by installing machine learning algorithms directly on edge devices. This article explores the concept of edge machine learning, how it works, and its broad implications across industries.
What is Edge ML?
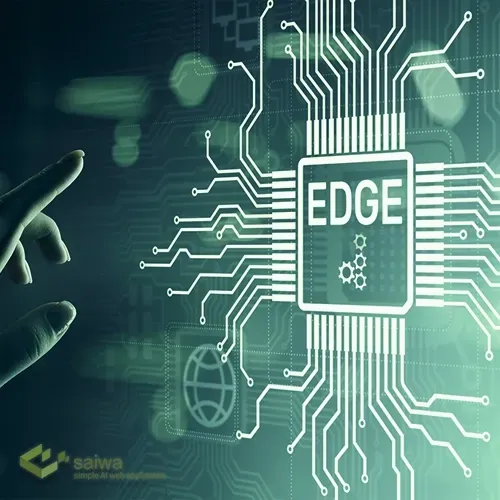
Edge ML, also known as edge machine learning, is an innovative approach that enables smart devices to process data locally, reducing reliance on cloud networks. Instead of sending data to centralized servers, edge machine learning uses machine and deep learning algorithms to perform processing at the device or local level, closer to where the data is collected. This advancement has recently gained significant attention, paralleling the growing Internet of Things (IoT).
IoT devices are becoming more prevalent, resulting in a massive influx of smart devices connected to cloud networks. However, the existing infrastructure struggled to keep up with the demand, leading to network congestion and overlooking the security concerns associated with cloud computing. Edge machine learning has emerged as a solution to these challenges, enabling devices to analyze and interpret data autonomously without a constant connection to the cloud.
Read more: What Is Machine Learning as a Service?
How does Edge ML Work?
Edge machine learning works by embedding machine learning algorithms and models directly on edge devices or edge computing systems, enabling local data processing and analysis. Here's an overview of how edge machine learning works:
Data Collection
Edge devices collect data from their respective sources, such as IoT sensors, smartphones, or embedded systems. This data can include sensor readings, images, sounds, and other input types.
Data Preprocessing
Locally at the edge device, the collected data is processed, standardized, or transformed into a format suitable for machine learning algorithms. Data cleaning, feature extraction, and data standardization are examples of preprocessing activities.
Model Deployment
A pre-trained machine learning model or algorithm is deployed on the edge device. This model is often tuned for execution on resource-constrained systems, considering model size, computational complexity, and memory requirements.
Local Inference
The installed machine learning models on edge devices execute local inference or prediction directly on the collected data.
Decision-Making
Edge devices can make real-time decisions or trigger particular actions based on the results of local inference.
Cloud Integration
While edge machine learning primarily works locally, there may be cases where certain tasks require additional computational resources or access to larger data sets. In these situations, edge devices can selectively send relevant data or partial results to the cloud for further processing, model training, or collaboration with other devices.
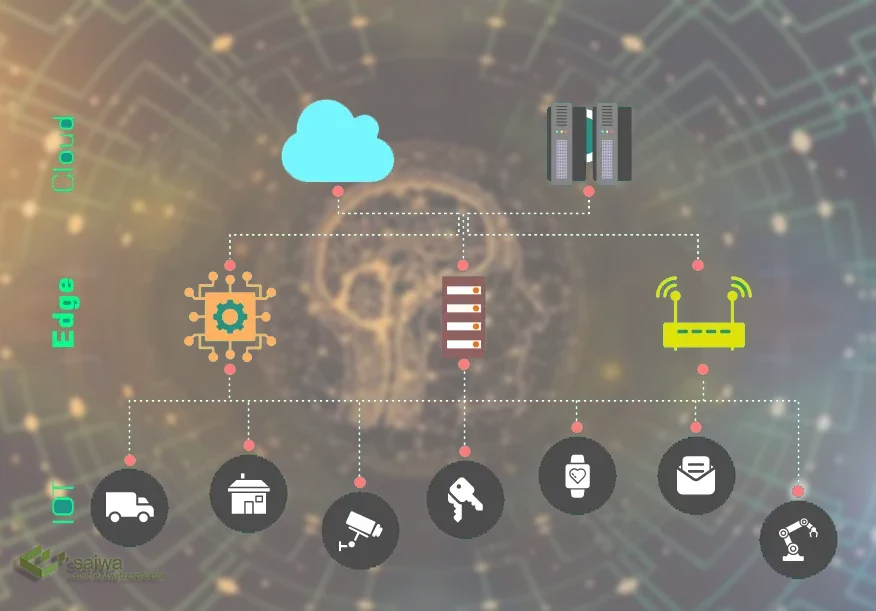
Why Should We Use Edge ML?
Edge machine learning is at the forefront of technological advances. It addresses security concerns by enabling local processing, eliminating the risks associated with storing user data in the cloud, and offloading to cloud networks. It also unlocks real-time data processing capabilities, a critical requirement for cutting-edge technologies such as autonomous vehicles and medical devices that are currently unattainable with traditional cloud-based devices.
As customer expectations continue to skyrocket, businesses face a growing need for fast and secure processing power. Today, every business-to-customer interaction involves a wide range of hybrid technologies and touchpoints. These interactions require rapid access to tools, information, and software that drive new experiences and deliver a seamless end-to-end user journey.
In these scenarios, the benefits of deploying edge machine learning are numerous, with the following being the most significant:
Real-time decision making
Cost Efficiency
Reduced latency
Offline functionality
Scalability and Reliability
Bandwidth efficiency
Privacy and security
Limitations of Edge Machine Learning
While edge machine learning (ML) offers significant benefits, it also has certain limitations that should be considered. Here are some of the key limitations of edge ML:
Limited computing resources
Storage limitations
Scalability challenges
Lack of continuous connectivity
Limited data diversity
Privacy and security risks
Edge Machine Learning use cases
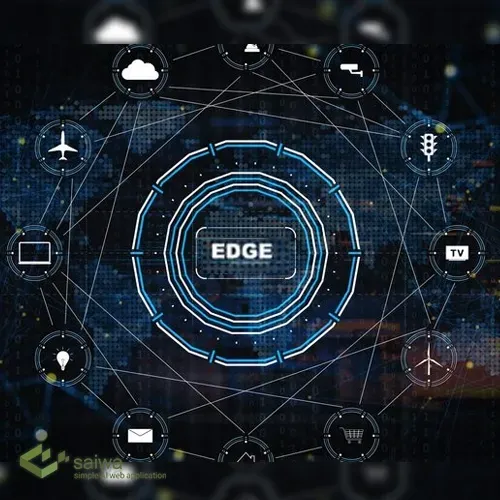
Edge ML has revolutionized several industries by permitting machine learning algorithms to run directly on edge devices, eliminating the need for constant connectivity to more powerful computers. This advancement has paved the way for innovative automation tools and smarter Internet of Things (IoT) systems. Here are some compelling examples of how edge machine learning is driving progress across industries:
Agriculture
Automatic identification of irrigation requirements to optimize water usage
Employment of ML-powered robots to capture and analyze data from crop trials, aiding in crop management and yield improvement.
Smart buildings
Smart HVAC systems intelligently adjust temperature and ventilation based on room occupancy, enhancing energy efficiency.
Security sensors equipped with ML algorithms that recognize the unique sound signature of breaking glass enable a faster response to potential intrusions.
Environmental conservation
Smart grid monitoring utilizes edge machine learning to detect early faults in power lines, helping prevent power outages and enhancing grid reliability.
Wildlife tracking systems leverage cutting-edge machine learning techniques to gather and analyze animal behavior, migration patterns, and habitat conservation data.
Health and Fitness
Portable medical devices integrated with edge machine learning capabilities can analyze medical images, aiding in identifying diseases or abnormalities.
Compact wearables utilize edge machine learning algorithms to track sleep patterns and workout activities, providing personalized health and fitness optimization insights.
Human-computer interaction (HCI)
Keyword spotting and wake word detection using edge ML allow users to control household appliances or virtual assistants through voice commands.
Gesture control as assistive technology enables intuitive interactions with devices and applications, which is particularly beneficial for individuals with mobility impairments.
Industry
Safety systems equipped with edge machine learning algorithms that automatically detect the presence of hard hats or personal protective equipment (PPE) enhance workplace safety.
Predictive maintenance solutions utilize edge machine learning to detect and analyze patterns in machinery data, identifying potential faults and enabling proactive maintenance, thereby reducing downtime and optimizing productivity.
Through the power of edge ML, these industries are experiencing significant advancements in efficiency, automation, and decision-making, leading to improved outcomes, reduced costs, and enhanced user experiences.
The Future of Edge Machine Learning
Edge ML has a promising future. More advanced machine learning models will be implemented at the edge as edge devices grow more powerful and capable of conducting complicated calculations. This implies that operations like picture recognition, natural language processing, and anomaly detection may be done directly on the device without needing an internet connection or cloud infrastructure.
Edge machine learning also addresses privacy and security concerns. By keeping data and computation local, sensitive information can be processed on the device, minimizing the risk of data breaches or unauthorized access. This approach is especially important in healthcare and finance, where data privacy is critical.
Edge machine learning also enables real-time decision-making by reducing latency. Instead of sending data to the cloud for analysis, edge devices can process data locally and provide immediate responses. This is especially beneficial for time-sensitive applications like autonomous vehicles, industrial automation, and augmented reality.