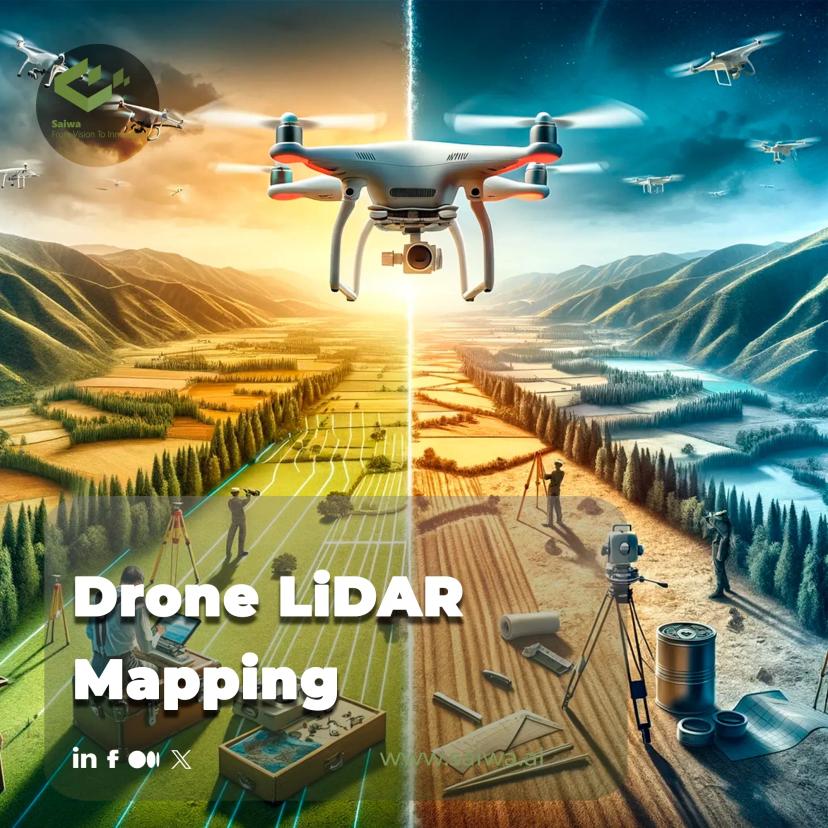
Drone LiDAR Mapping | The Future of Data Acquisition
The integration of LiDAR (Light Detection and Ranging) technology with unmanned aerial vehicles (UAVs), commonly known as drones, has revolutionized the field of spatial data acquisition. Leading the charge in this technological revolution is Sairone, a subsidiary of Saiwa, specializing in advanced UAV-based solutions.
Sairone combines cutting-edge image processing and machine learning techniques with UAV technology to offer precise and efficient monitoring solutions across various domains. Drone LiDAR mapping offers a rapid, efficient, and highly accurate method for creating detailed three-dimensional (3D) representations of the Earth's surface.
This article explores the fundamental principles of LiDAR technology, suitable drone platforms, LiDAR sensor considerations, and the data processing workflows involved in drone LiDAR mapping. Furthermore, we will delve into the diverse applications of this technology across various industries and explore the benefits and advanced data processing techniques that enhance the capabilities of drone LiDAR mapping.
What is LiDAR Technology?
Light Detection and Ranging (LiDAR) is a remote sensing technology that employs pulsed laser light to measure distances. In a LiDAR system, laser pulses are emitted towards a target, and the reflected light is captured by a sensor. By measuring the time required for the light pulse to travel to the target and return, the system can ascertain the distance to that point. By rapidly scanning the environment with these laser pulses, LiDAR generates a highly detailed point cloud representation of the target area.
Sairone's expertise in integrating LiDAR with UAVs has resulted in notable advancements in data accuracy and efficiency, facilitating the development of tailored solutions for a wide range of industrial applications. Furthermore, their sophisticated data processing methodologies facilitate the extraction of valuable insights from LiDAR data, rendering it suitable for a multitude of applications.
Here's a breakdown of the core operating principles of LiDAR technology:
LiDAR operating principles
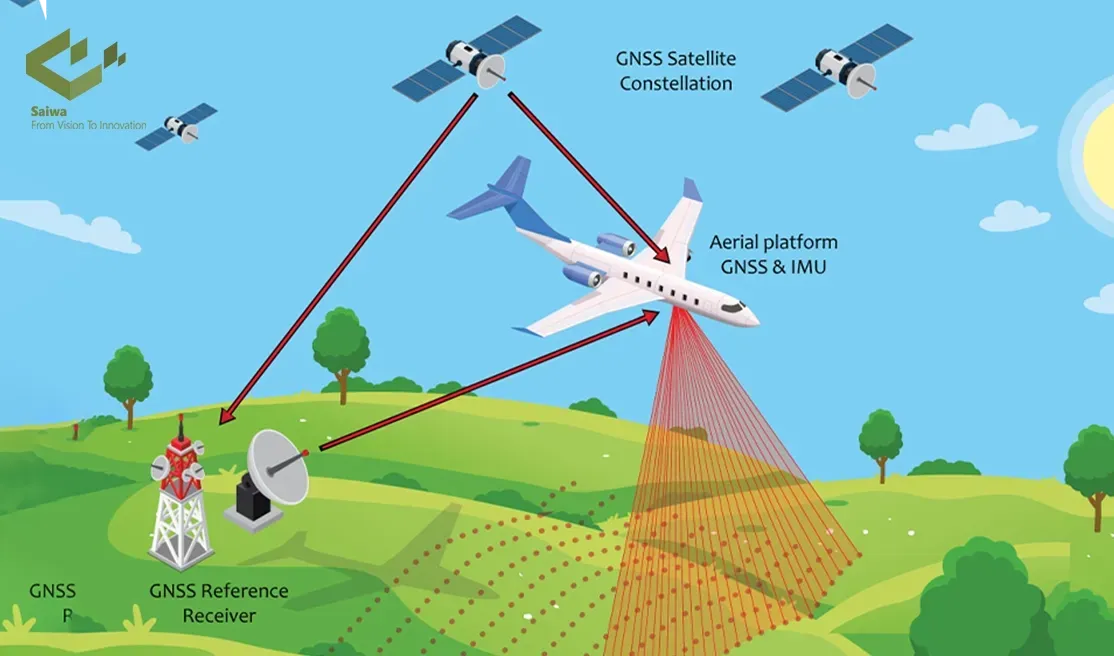
A LiDAR system is comprised of three primary components: a laser source, a scanner that directs the laser beam, and a receiver that detects the reflected light. The laser emits short, intense pulses of light at a specific wavelength. The scanner oscillates the laser beam in a rotary motion, directing it toward disparate points within the environment. Upon impact with a target surface, a portion of the laser pulse is reflected back towards the LiDAR sensor. The receiver detects the reflected light and measures the time elapsed between the emission and reception of the laser pulse. By employing the principle of the speed of light, the system is capable of calculating the distance to the target based on the time-of-flight measurement.
Types of LiDAR systems
There are various types of LiDAR systems, each with its own operating principles and applications. Common types include:
Time-of-flight (TOF) LiDAR: This is the most widely used type, as described above, where distance is measured based on the time it takes for the light pulse to travel to the target and back.
Phase-shift LiDAR: This type utilizes a continuous wave laser and measures the phase shift of the reflected wave to determine the distance.
Waveform LiDAR: This advanced type analyzes the entire waveform of the reflected laser pulse to extract additional information, such as target characteristics and material properties.
Read Also : AI-Based Drone Operation| AI in Drones Use Cases
Key components of a LiDAR system
A LiDAR system consists of several crucial components:
Laser source: This generates the pulsed laser light used for ranging measurements. Common laser sources in LiDAR systems include solid-state (diode) lasers and fiber lasers.
Scanner: This mechanical or optical device directs the laser beam toward different points within the environment. Scanners can be rotating mirrors, oscillating prisms, or electronically steered beams.
Receiver: This detects the reflected laser light and measures its properties, such as intensity and arrival time. Receivers typically consist of photodetectors sensitive to the specific laser wavelength used.
Global Navigation Satellite System (GNSS) receiver: Provides the LiDAR system with its position and orientation data, allowing for georeferencing of the collected LiDAR points.
Inertial Measurement Unit (IMU): Measures the drone's attitude (pitch, roll, yaw) and accelerations, aiding in correcting for platform motion during data acquisition.
Drone Platforms for LiDAR Mapping
The choice of drone platform significantly impacts the capabilities and efficiency of drone LiDAR mapping missions. Here are key considerations for selecting suitable drones:
Types of drones suitable for LiDAR integration
Several types of drones can be used for LiDAR mapping, each with its own advantages and limitations:
Multicopters: These are the most common type of drone used for LiDAR mapping due to their stability, maneuverability, and ability to take off and land vertically.
Fixed-wing drones: These offer longer flight times and higher coverage areas compared to multicopters, but require runway takeoff and landing.
VTOL (Vertical Take-Off and Landing) fixed-wing drones: Combine the advantages of fixed-wing drones (long range) with the vertical takeoff and landing capabilities of multicopters, offering greater operational flexibility.
Read Also: Leveraging Drones in Agriculture for a New Era of Data-Driven Farming
Payload capacity and flight endurance considerations
The drone platform must be able to carry the LiDAR sensor, any additional sensors, and batteries for the desired flight duration. Flight endurance is important because it allows data to be collected over larger areas. The best balance between payload capacity and flight endurance depends on the project requirements and the size/weight of the LiDAR sensor.
Drone stability and vibration mitigation
Stability is critical for accurate LiDAR data collection. Drones with robust flight controllers and good vibration isolation are preferred to minimize any platform movement that could affect the quality of the LiDAR point cloud. Gimbal stabilization platforms can be used to isolate the LiDAR sensor from drone vibration, further improving data quality.
LiDAR Sensors for Drone Integration
The selection of a suitable LiDAR sensor is critical for drone LiDAR mapping missions. Here are key factors to consider:
Compact LiDAR sensors for UAV applications
LiDAR sensors for drone integration must be compact, lightweight, and low-power to minimize payload weight and maximize flight time. These sensors are often optimized for UAV applications and may have compromises in range or point density compared to larger terrestrial LiDAR systems.
Performance metrics (range, accuracy, point density)
Several performance metrics define the capabilities of a LiDAR sensor:
Range: This refers to the maximum distance the LiDAR system can accurately measure. The range is influenced by factors like laser power, receiver sensitivity, and atmospheric conditions.
Accuracy: This indicates the precision of the distance measurements. Higher accuracy LiDAR systems provide more detailed and reliable point clouds.
Point density: This refers to the number of data points collected per unit area. Higher point density results in a more detailed and information-rich point cloud representation of the environment.
Scanning mechanisms and field of view
LiDAR sensors employ different scanning mechanisms to direct the laser beam:
Rotating scanners: These mechanically rotate a mirror or prism to deflect the laser beam, creating a scan line across the environment.
MEMS scanners: These utilize micro electro mechanical systems (MEMS) technology to electronically steer the laser beam, offering faster scan rates and potentially higher accuracy compared to rotating scanners.
LiDAR cameras: These are emerging technologies that combine a LiDAR system with a camera sensor, capturing color information along with the LiDAR point cloud data.
The field of view (FOV) of the LiDAR sensor determines the area covered in a single scan line. A wider FOV allows for faster data acquisition over larger areas but may come at the expense of point density.
Read Also: Fields from Above | Innovations in Agricultural Drone Mapping
Data Processing and Analysis
Raw LiDAR data collected during a drone LiDAR mapping mission requires processing and analysis to generate usable geospatial information. Here's an overview of the typical workflow:
Raw data preprocessing and filtering
Raw LiDAR data may contain noise from various sources, such as sensor noise, atmospheric effects, and ground clutter. Preprocessing steps involve filtering the data to remove noise and outliers, ensuring the accuracy and reliability of the point cloud.
Point cloud classification and segmentation
The point cloud data needs to be classified to differentiate between ground points, vegetation points, and other objects within the scene. Classification algorithms can utilize various features, such as point intensity, elevation, and spatial relationships, to categorize the points. Segmentation further groups points belonging to the same object or feature, enabling the extraction of specific features from the LiDAR data.
Feature extraction and object recognition
Once the point cloud is classified and segmented, features of interest can be extracted. These features could include digital elevation models (DEMs), building footprints, vegetation characteristics, or other relevant information specific to the project requirements. Object recognition algorithms can be employed to identify specific objects within the scene, such as trees, power lines, or buildings.
Applications of Drone LiDAR Mapping
Drone LiDAR mapping offers a versatile tool for various applications across diverse industries:
Topographic surveying and mapping
LiDAR data is ideal for creating highly accurate and detailed topographic maps, essential for infrastructure planning, construction projects, and environmental monitoring.
Forestry and vegetation analysis
LiDAR can be used to assess forest health, measure tree height and canopy cover, and estimate timber volume. This information is crucial for sustainable forest management practices.
Urban planning and smart city applications
Detailed 3D cityscapes generated from LiDAR data can be used for urban planning, infrastructure management, and developing smart city initiatives.
Infrastructure inspection and asset management
LiDAR is valuable for inspecting bridges, buildings, and other infrastructure assets, allowing for rapid damage assessment and facilitating maintenance planning.
Archaeology and cultural heritage documentation
LiDAR can be used to create detailed 3D models of archaeological sites and historical structures, aiding in documentation, and preservation efforts.
Mining and quarry surveying
Drone LiDAR enables efficient volume estimation of stockpiles and accurate mapping of mining and quarry sites, optimizing operations.
Disaster management and emergency response
Rapid and detailed 3D mapping of disaster zones using drone LiDAR can aid in search and rescue efforts, damage assessment, and post-disaster reconstruction planning.
Benefits of LiDAR Drones
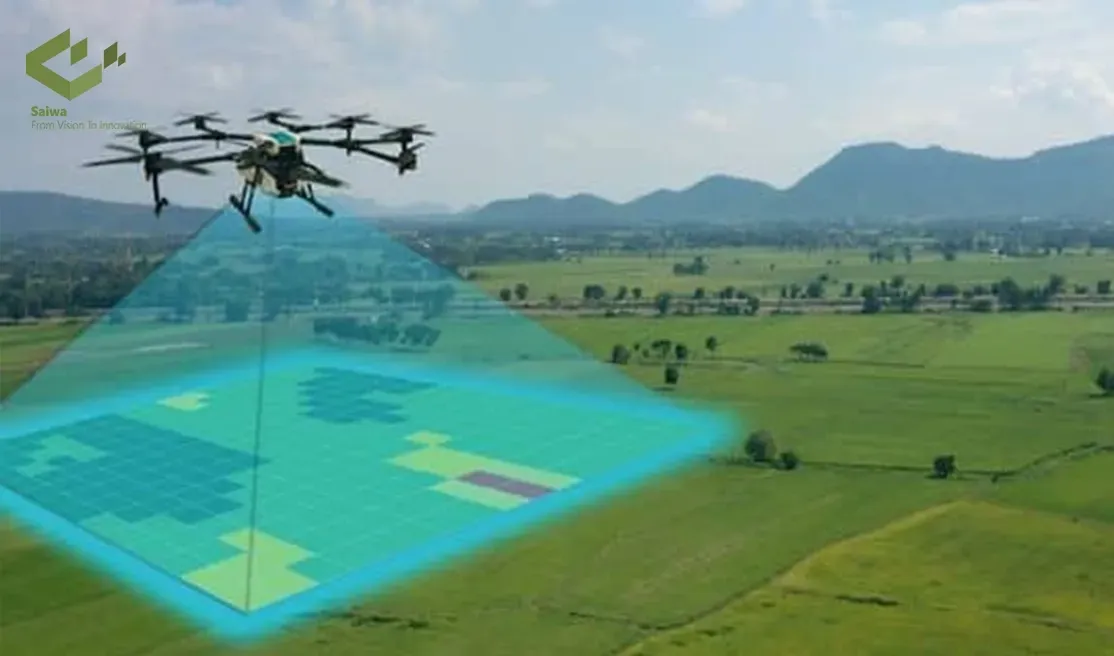
Drone LiDAR mapping offers several advantages compared to traditional surveying methods:
Speed and efficiency: Drone LiDAR allows for rapid data collection over large areas, significantly faster than traditional ground-based surveying methods.
Cost-effectiveness: The combination of drones and LiDAR technology can be more cost-effective than traditional methods, especially for large-scale projects.
Accessibility: Drones can access areas that are difficult or dangerous to reach using ground-based methods, such as steep slopes or hazardous environments.
High accuracy and detail: LiDAR data provides highly accurate and detailed 3D point clouds, enabling the creation of precise maps and models.
Safety: Drone LiDAR eliminates the need for personnel to be present in potentially hazardous environments, improving safety during data collection.
Advanced Data Processing Techniques
The field of drone LiDAR data processing is constantly evolving, with new techniques emerging to enhance the quality and usability of the data:
Machine learning for point cloud classification and segmentation: Machine learning algorithms are increasingly being used to automate point cloud classification and segmentation tasks. These algorithms can learn from labeled datasets to identify and classify features within the LiDAR data with high accuracy.
Deep learning for object recognition and feature extraction: Deep learning techniques are showing promise in extracting complex features and recognizing objects within drone LiDAR data. Object recognition with deep learning allows for more advanced applications, such as automatic building extraction or power line inspection.
3D modeling and visualization: Advanced software tools can convert LiDAR point clouds into realistic 3D models of the surveyed environment. These models can be used for visualization, simulation, and further analysis.
Integration with other geospatial data: LiDAR data can be integrated with other geospatial datasets, such as high-resolution imagery, for even more comprehensive and informative spatial analysis.
Conclusion
Drone LiDAR mapping has become a powerful tool for various industries requiring high-resolution, 3D spatial data. The combination of compact and efficient LiDAR sensors with versatile drone platforms allows for rapid and cost-effective data collection over large areas. Advanced data processing techniques further enhance the usability of LiDAR data, enabling the extraction of valuable information for diverse applications. As drone technology and LiDAR sensor capabilities continue to evolve, we can expect even broader adoption of drone LiDAR mapping across various sectors, revolutionizing the way we capture, analyze, and utilize spatial data.