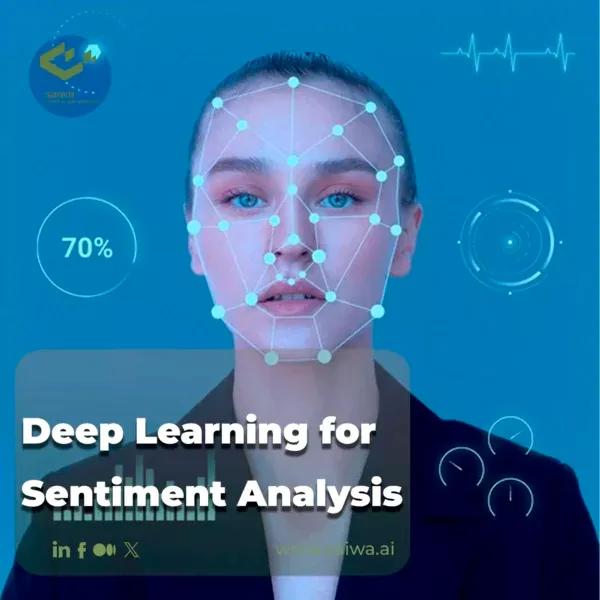
Deep Learning for Sentiment Analysis | Decoding Emotions
Sentiment analysis is a text analytics tool that automatically extracts opinion and sentiment from unstructured data using machine learning and deep learning algorithms.
Deep learning is considered an evolution of machine learning. Deep learning combines algorithms that aim to simulate the way the human brain works, also known as an artificial network, with many practical applications of machine learning, including customer support automation and self-driving cars.
In this article, we will explore and discuss deep learning in sentiment analysis.
Read Also: AI for Sentiment Analysis | From Data to Actionable Insights
What is Sentiment analysis?
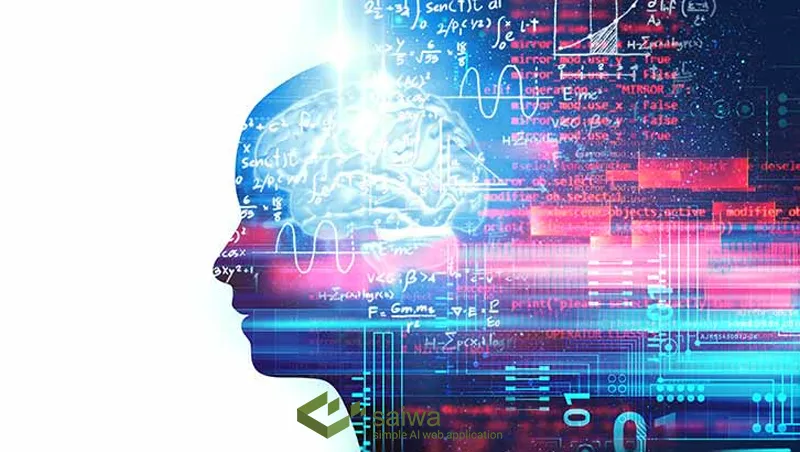
Sentiment analysis is an analytical technique that uses statistics, natural language processing, and machine learning to determine the emotional meaning of communications. Companies use sentiment analysis to evaluate customer messages, contact center interactions, online reviews, social media posts, and other content. Sentiment analysis can track changes in attitudes toward companies, products or services, or individual characteristics of those products or services.
Sentiment analysis tools
The basic level of sentiment analysis involves statistics or machine learning based on supervised or semi-supervised learning algorithms. Supervised learning involves humans to score datasets. With semi-supervised learning, there is a combination of automatic learning and periodic checks to make sure the algorithms are working correctly.
Deep learning is a tool by which sentiment analysis is done. Deep learning uses a network of layered neural hubs that are modeled after the way the human brain works. This level, more complex than sentiment analysis, can consider all sentences and complete conversations to determine sentiment, and can also be used for audio and video analysis.
All cloud providers offer sentiment analysis tools, as do major customer support platforms and marketing vendors. Vendors of conversational AI also provide sentiment analysis tools.
Companies interested in conducting sentiment analysis should first consider the tools and technologies they currently use. Do they have a survey tool that has sentiment analysis? Customer feedback management platforms also have basic sentiment analysis.
Deep learning in sentiment analysis, NLP, and ML
Few companies build their sentiment analysis platforms. This requires in-house expertise and large training datasets but may be appropriate for companies that have very specific needs that cannot be met by existing platforms. In these cases, companies typically build their tools using open-source libraries.
For deep learning, sentiment analysis can be performed using BERT, XLNet, and GPT3 models. GPT3 can perform sentiment analysis without any training data.
It should be noted that the creation of sentiments by companies themselves can give them a greater advantage over their competitors. This is a trend that can be seen in the market and large companies. Not buying the competitor's tool from the same place as Korea's choice is considered a competitive advantage.
Sentiment analysis APIs
A common approach for companies building their platforms is to include sentiment analysis functionality via an API. All major cloud providers offer this service. The question that arises at this stage is how suitable these APIs are if you have a specific product, they should work hard to be suitable.
The next issue here is the discussion of cost. Each API call has a cost. You must ensure that calls to these specific APIs are economically viable. But it can be a good solution for small and medium businesses.
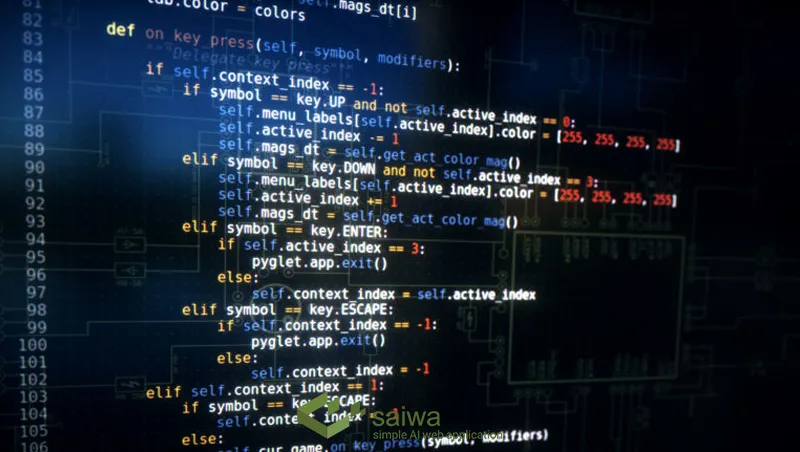
Sentiment analysis dataset
Machine language and deep learning approaches to sentiment analysis require large training data sets. Commercial and publicly available tools typically have large databases, but they are usually very general and not limited to specific industries.
For many companies, it can be difficult to get the information they need from their customers to build a model. However, NLP models are now being calibrated using technologies such as transfer learning on extremely large corpora with billions and billions of records and then applied to different use cases.
This means that a company with a small set of domain-specific training data can start with a business tool and customize it to their needs.
Face Sentiment Analysis
Face sentiment analysis refers to using automated techniques to infer emotions and attitudes from facial expressions. This emerging technology has important applications in fields like marketing, education, healthcare, and human-computer interaction. Systems can categorize basic emotions like happiness, sadness, anger, surprise, and more complex affective states to understand sentiment. However, accurately detecting and interpreting subtle facial cues poses significant challenges.
Applications of Face Sentiment Analysis
Some of the key domains leveraging face sentiment analysis include:
Marketing Research: By analyzing facial reactions to advertisements, commercials, or product experiences, brands can better understand consumer attitudes and emotional resonance. Real-time dial testing captures biometric data along with video facial coding to gauge sentiment.
Education: Detecting student engagement, confusion, or frustration through facial cues can inform improved teaching methods and adaptive learning. Sentiment analysis has been used for e-learning, intelligent tutoring systems, and evaluating student mindset.
Healthcare: Monitoring facial expressions enables more objective assessment of patient pain levels, depression, cognitive decline, and response to treatments. Particularly useful when patients have limited communication.
Automotive: In-cabin cameras with sentiment analysis can detect driver drowsiness, distraction, anger, and other dangerous states based on facial expressions, even if the driver tries to hide these reactions.
Human-Computer Interaction: User interfaces that respond supportively to human emotion and are capable of convincingly expressing empathy rely on accurate face sentiment interpretation.
These applications demonstrate the broad value of inferring inner emotional states through externally observable facial expressions and micro expressions.
Facial Action Coding Approach
Early face sentiment analysis techniques relied on facial action coding systems. This approach manually encodes the activation of specific facial muscles into coded action units based on the Facial Action Coding System (FACS) developed by psychologist Paul Ekman. For example, action unit 12 represents a lip corner pull, while action unit 27 involves mouth stretching. By identifying the combination of activated action units, human coders can classify emotions.
FACS provides an objective anatomical basis for coding subtle changes in facial structure. However, even trained experts require extensive time to manually score facial action units in videos or image sequences. Reliably interpreting compound emotions and implicit sentiments from coded action units also poses challenges. This labor-intensive process limits adoption outside research contexts.
Top sentiment analysis use cases
The biggest use of sentiment analysis in the industry today is in call centers, where customer communications and call logs are analyzed. For example, a spike in negative sentiment following the release of a new product can be an early warning sign of a problem, and the company can conduct an in-depth investigation to find out what features are causing the problems.
With customer support now involving more Web-based video calls, there is also a large amount of video training data. The same type of technology used to perform sentiment analysis for the customer experience can be applied to the employee experience.
Instead of having HR call everyone to see if everything is going well and if they feel supported, consider using a conversational chatbot. This can help companies find areas where employees are struggling or don't feel supported.
Sentiment analysis can be used for brand management to help companies understand how segments of their customers feel about their products and help them tailor marketing messages to those customers. Target more appropriately.
This is especially useful for public relations. You can catch negative sentiment early and do crisis management, or if you're running a promotion, you can find out if people like it or not.
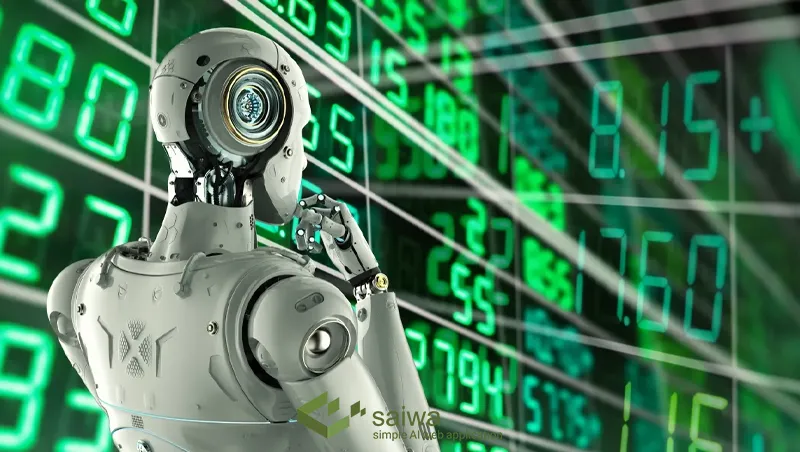
Conclusion
Deep learning in sentiment analysis provides undeniable analysis results, no matter whether it's ordinary documents or business reports, social media monitoring, customer support tickets, or anything else. Deep learning allows you to put more powerful patterns and more tools to work on your data.