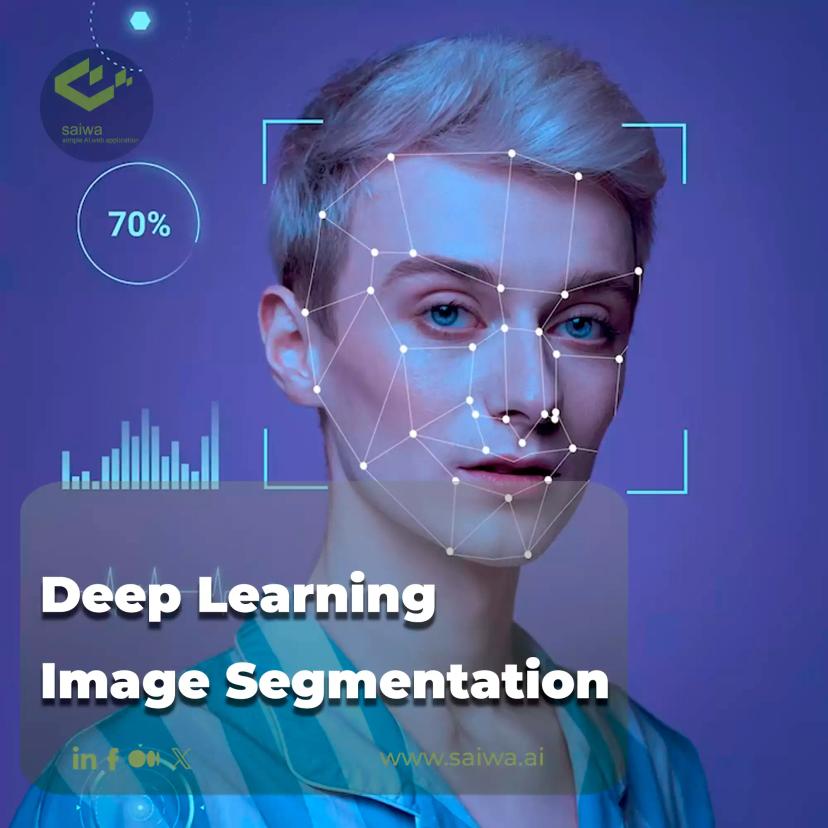
Deep Learning Image Segmentation | Precision Unleashed
Image segmentation is a crucial computer vision task with applications in medical imaging, robotics, autonomous vehicles, and other fields. Traditional image processing techniques such as thresholding and edge detection are inadequate at accurately segmenting complex, real-world imagery. However, deep learning image segmentation has facilitated significant advancements in this area. In this paper, we present an overview of the image segmentation problem, including advances in convolutional neural network technology that are pushing the boundaries of state-of-the-art approaches. We also discuss various methods for evaluating these approaches and explore potential areas for future research.
Traditional Image Processing Methods
Before deep learning, image segmentation depended on hand-designed pipelines. Grayscale thresholding separates light and dark areas based on pixel intensity. Edge detection extracts image contours that can then be linked to cluster regions. Normalized cuts partition graphs founded on pixel similarity. Active contours continuously refine boundaries. However, these techniques do not adequately capture semantics and have difficulty with cluttered images. Deep learning has since resolved these restrictions through data-driven feature learning.
Fundamentals of Image Segmentation
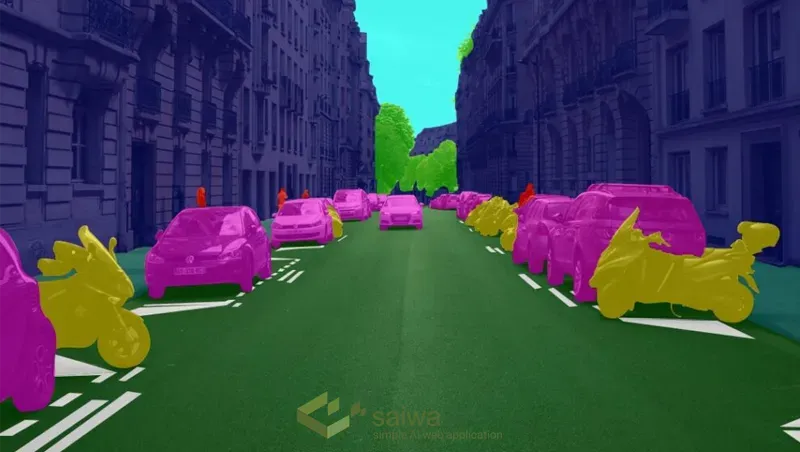
Image segmentation has been an enduring focus in computer vision. Classical approaches relied on hand-engineered pipelines involving edge detection, thresholding, region growing, and clustering. However, these methods struggled with complex images and lacked flexibility. Deep learning has since revolutionized segmentation through data-driven feature learning.
Read Also : Deep Learning Image Segmentation | Precision Unleashed
The Role of Convolutional Neural Networks (CNNs)
Convolutional neural networks are optimal for image segmentation. Architectures such as fully convolutional networks (FCNs) and U-Net utilize encoder-decoder designs. The encoder extracts features through convolutions, while the decoder upsamples using transposed convolutions to restore spatial resolution. Pre-trained models provide generalized features to bootstrap learning. Loss functions that consider pixel interdependence enhance coherence and accuracy.
Deep learning image segmentation poses challenges. Complex scenes with occlusion and small objects strain model accuracy, and high-resolution imagery requires efficient processing. Weakly supervised and interactive segmentation paradigms aim to reduce annotation requirements while incorporating human guidance. As research addresses these challenges, deep networks will continue to extend the horizons of computer vision and image understanding.
Thorough evaluation requires using metrics such as intersection over union to measure the overlap between predicted and ground truth segmentation. Confusion matrices help identify errors between classes, while benchmark datasets track progress by showcasing new techniques that surpass 80% mean IoU on challenging content. Ongoing research aims to improve the accuracy, efficiency, and generalization of models.
The Evolution of Deep Learning Image Segmentation
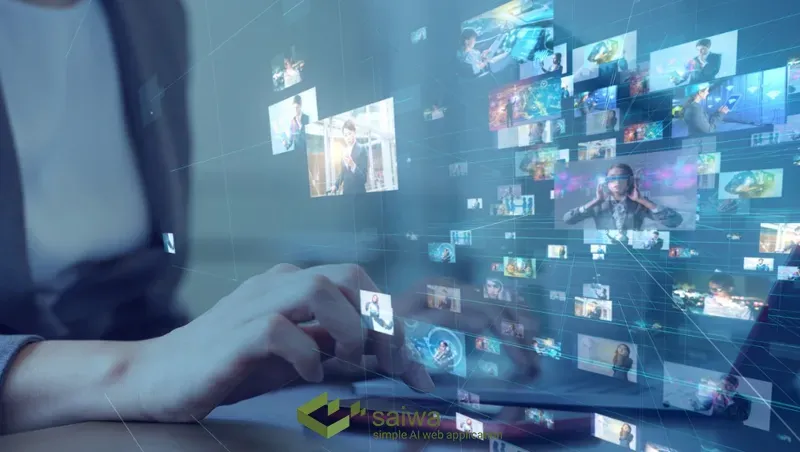
Early methods for image segmentation relied on manually crafted rules. Thresholding separates pixels based on their intensity, and edge detection extracts contours to delineate objects. However, these traditional methods fail to capture semantic context. Deep learning has since surpassed these limitations through data-driven representation learning.
Convolutional neural networks (CNNs) now enable end-to-end learning of semantic relationships directly from pixels. Architectures like fully convolutional networks (FCNs) and U-Net achieve remarkable segmentation results by combining hierarchical feature extraction with upsampling and skip connections to recover spatial detail.
Utilizing pre-trained CNNs as feature extractors is essential to this progress. ResNet models that are pre-trained on ImageNet provide ample representations to initiate segmentation networks. Employing this transfer learning technique is far more effective than starting the training from square one. Fine-tuning the decoder and upsampling components customizes these characteristics for the segmentation job.
Performance is commonly measured using intersection over union (IoU) between predicted and true segmentation masks. Widely used datasets like PASCAL VOC and Cityscapes reflect real-world complexity. Detailed evaluation guides research and tracks progress on benchmark leaderboards over time as new techniques improve the state of the art.
With accurate and efficient deep learning image segmentation, applications like medical imaging, robotics, and image editing have been transformed. Ongoing research aims to improve generalization, leverage weakly labeled data, and incorporate human guidance. Deep learning will continue advancing computer vision toward human-level scene understanding.
Benefits of Deep Learning Image Segmentation
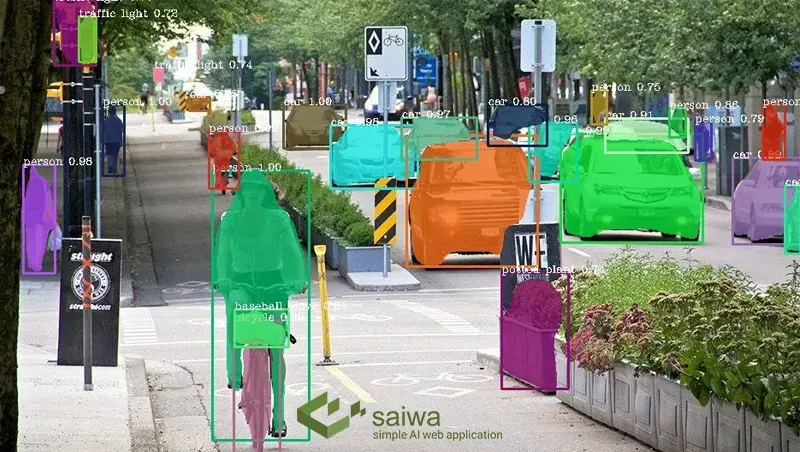
Deep learning delivers unprecedented segmentation capabilities that enable transformative computer vision applications.
- Pixel-level precision is not possible with whole-image classification
- Automated feature learning without hand-coded rules
- State-of-the-art accuracy surpassing human levels
- Real-time performance with optimized deep networks
- Generalizable to diverse use cases like medical, driving, etc.
- Scalable training leveraging large annotated datasets
- Flexible implementations using deep learning frameworks
- Powerful representation learning for downstream tasks
Ongoing Challenges and Opportunities
Despite recent progress, segmenting images can still be challenging when they lack clarity or contain complex occlusion patterns. There is room for improvement in both the efficiency of our models and the speed of our inferences, especially for applications such as autonomous driving. Exploring techniques that reduce the time-consuming task of annotation, such as weakly supervised and semi-supervised methods, is crucial. Additionally, interactive segmentation methods that incorporate human guidance also warrant further investigation. As datasets become more diverse, the importance of continual learning to segment new classes over time will increase. In general, deep learning will continue to advance segmentation capabilities in the years to come.
Conclusion
Deep learning has propelled image segmentation to new heights, overcoming the limitations of earlier approaches. CNNs now deliver real-time semantic segmentation on par with human perception. This milestone in computer vision unlocks transformative applications across domains. However, work remains to improve robustness, model efficiency, and accessibility. Overall, deep learning image segmentation offers immense value for intelligent systems as well as new possibilities for humans to interact with the visual world.