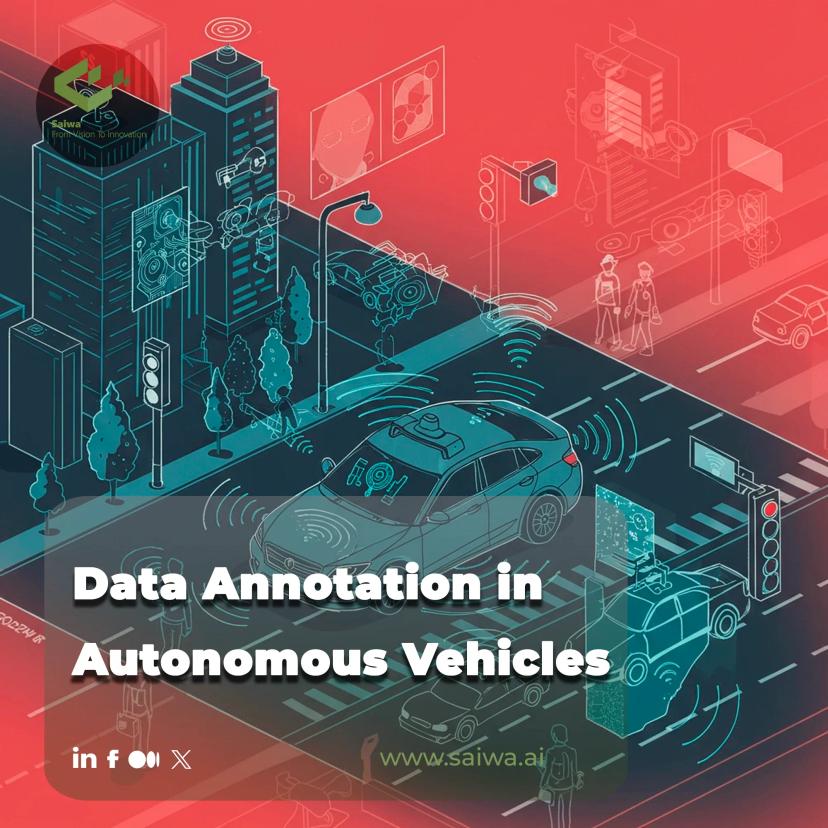
The Role of Data Annotation in Autonomous Vehicles
The development of autonomous vehicles (AVs), also known as self-driving cars, represents a significant leap forward in transportation technology. These vehicles, capable of navigating and operating without human intervention, rely heavily on sophisticated algorithms and vast amounts of data. Central to the success of AVs is the process of data annotation, which involves labeling and categorizing data to enable machine learning models to understand and interpret the environment.
Fraime, offered by Saiwa, provides a comprehensive AI-as-a-Service (AIaaS) platform that includes specialized services such as detection, annotation, and image processing. In the context of autonomous vehicles, Fraime’s annotation tool plays a crucial role in efficiently labeling and categorizing data to train machine learning models, whether for image recognition or more complex sensor data interpretation.
Its flexibility also allows for easy customization to suit video tagging applications, making it an ideal choice for tasks that require accurate and scalable data annotation.
This article examines the critical role of data annotation in the development and deployment of autonomous vehicles, exploring its various applications, benefits, challenges, and future prospects. From image recognition to sensor fusion, data annotation is the foundation upon which AVs perceive and interact with the world.
The Need for Data Annotation in Autonomous Vehicles
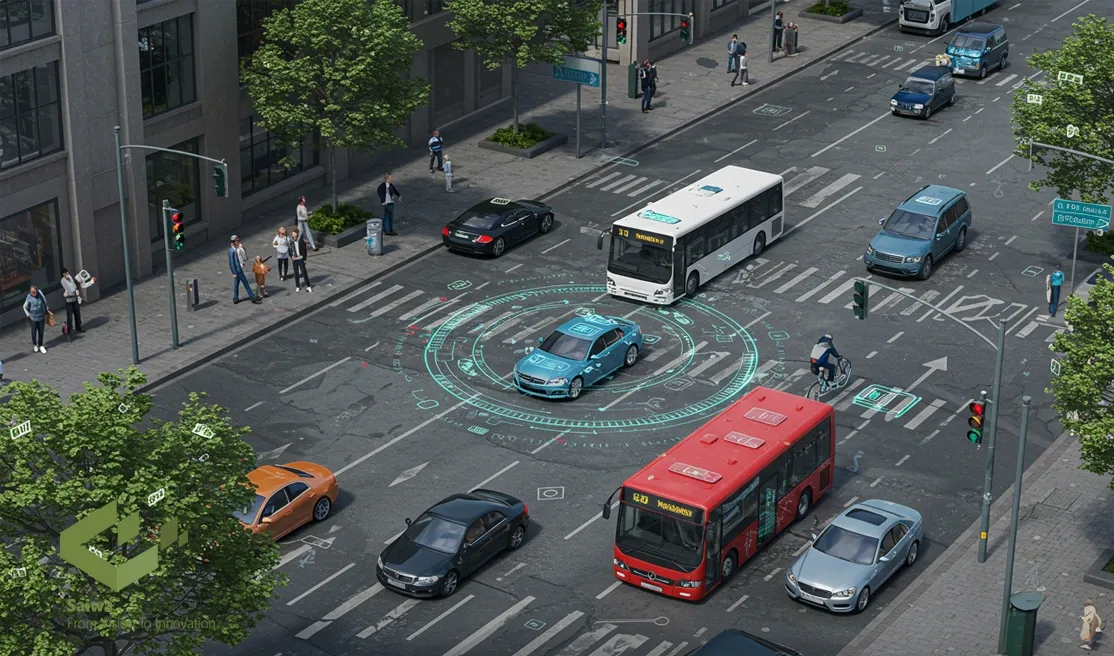
Autonomous vehicles operate in complex and dynamic environments, requiring them to perceive and interpret a constant stream of information. This information comes from various sources, including cameras, LiDAR, radar, and GPS, and must be processed in real time to enable safe and efficient navigation.
Data annotation plays a crucial role in this process by providing the labeled data necessary to train the machine learning models that power AVs. These models rely on annotated data to learn to identify objects, understand road conditions, predict the behavior of other road users, and make informed driving decisions. Without high-quality annotated data, AVs would be unable to function reliably in the real world.
Localization
Localization is the process of determining the precise location of the autonomous vehicle within its environment. Accurate localization is essential for safe navigation, enabling the vehicle to plan its route, avoid obstacles, and maintain its position on the road.
Data annotation plays a critical role in localization by providing labeled data that helps the vehicle understand its surroundings. This data may include landmarks, road markings, and other features that can be used to pinpoint the vehicle's location.
Detection
Detection involves identifying and classifying objects within the vehicle's environment, such as other vehicles, pedestrians, cyclists, and traffic signs. Accurate detection is crucial for safe and efficient driving, enabling the vehicle to anticipate potential hazards and react accordingly.
Data annotation is essential for training the object detection models used by AVs. This involves labeling objects within images and videos, providing the models with the ground truth information needed to learn to identify and classify objects accurately.
Voice Assistance
Voice assistance systems are becoming increasingly integrated into autonomous vehicles, allowing passengers to interact with the vehicle using natural language commands. Data annotation is crucial for training these systems to understand and respond to voice commands.
This involves transcribing and labeling audio data, enabling the system to recognize different commands and perform the requested actions.
Data Annotation for Image Recognition in Autonomous Vehicles
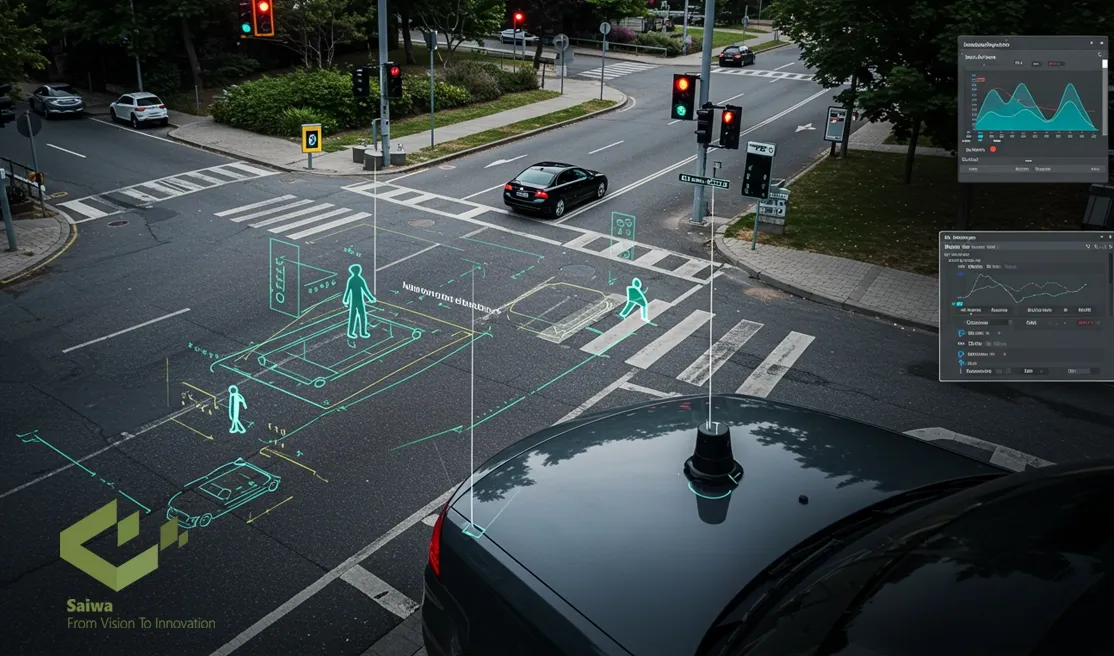
Image recognition forms the cornerstone of an autonomous vehicle's perception system, allowing it to interpret visual data from cameras and understand its surroundings. Data annotation plays a crucial role in training the sophisticated image recognition models that enable AVs to identify and classify objects, interpret road conditions, understand traffic signals, and ultimately navigate safely.
The process involves meticulously image labeling and videos to provide the ground truth data necessary for training these models. This annotated data teaches the AV to distinguish between various objects, understand their spatial relationships, and interpret the complex visual landscape of the road. The effectiveness of image recognition in AVs directly depends on the quality and accuracy of the data annotation process.
Object Detection
Object detection is a critical function within image recognition, focusing on identifying and classifying specific objects within an image, such as vehicles, pedestrians, cyclists, and traffic signs. Human annotators meticulously label these objects, providing the essential ground truth data that train object detection models.
Various annotation techniques are employed to achieve accurate object identification, including bounding boxes, polygons, and semantic segmentation. These techniques allow for precise labeling of objects, outlining their shapes and positions within the image. The accuracy and precision of object detection are paramount for safe autonomous driving, enabling the vehicle to react appropriately to its surroundings.
Lane Detection
Lane detection is essential for maintaining the autonomous vehicle's correct position on the road, ensuring it stays within its designated lane. Annotators carefully label lane markings in images, providing the visual cues that the AV uses to perceive lane boundaries.
This process typically involves drawing lines or polygons along the lane markings, creating a clear definition of the lane's boundaries. Accurate lane detection is crucial for safe and controlled navigation, preventing lane departures and ensuring the vehicle follows the intended path.
Mapping and Localization
Data annotation contributes significantly to the creation of highly detailed maps used by autonomous vehicles for localization and navigation. Annotators label landmarks, road features, and other relevant information within images, providing the spatial context that allows the AV to accurately determine its location. This labeled data is used to build and refine maps, enabling the vehicle to pinpoint its position within the environment and plan its route effectively. Accurate mapping and localization are fundamental for efficient and reliable autonomous navigation.
Prediction and Planning
Data annotation plays a key role in developing the predictive models that allow autonomous vehicles to anticipate the behavior of other road users and plan their trajectory accordingly. By labeling the movements and actions of other vehicles and pedestrians, annotators provide the data necessary to train these sophisticated predictive models.
This data enables the AV to understand and predict the likely actions of other road users, allowing it to make proactive driving decisions and enhance safety. Predictive capabilities are essential for navigating complex traffic scenarios and ensuring safe interactions with other road users.
Data Annotation's Role in Enhancing Radar and Sensor Systems
Beyond image recognition, data annotation is essential for optimizing the performance of radar and sensor systems, which provide critical information about the vehicle's environment.
LiDAR and radar sensors
LiDAR and radar sensors provide crucial data about the vehicle's surroundings, including distance to objects, velocity, and reflectivity. Data annotation is used to label this sensor data, enabling the autonomous vehicle to accurately interpret the readings and build a comprehensive understanding of its environment.
This process involves labeling point clouds generated by LiDAR and radar with corresponding object classes and locations, enhancing the accuracy and reliability of the sensor data. This labeled data enables the AV to perceive its surroundings with greater precision, improving its ability to navigate safely.
Integrating data from various sources
Autonomous vehicles rely on a fusion of data from multiple sensors, including cameras, LiDAR, radar, and GPS. Data annotation facilitates the seamless integration of data from these diverse sources. By synchronizing and labeling data from different sensors, annotators ensure consistency and accuracy, enabling the vehicle to create a more complete and reliable representation of its environment. This data fusion enhances the AV's situational awareness and improves its ability to make informed driving decisions.
The ability to detect pedestrians and recognize traffic signs
Detecting pedestrians and recognizing traffic signs are critical safety functions for autonomous vehicles. Data annotation plays a vital role in training the models responsible for these functions. Annotators meticulously label pedestrians and traffic signs in images and sensor data, providing the ground truth information needed to train these models.
Accurate detection of pedestrians and traffic signs is paramount for safe autonomous driving, enabling the vehicle to react appropriately and avoid potential hazards.
The Advantages of Using Data Annotation Services in Autonomous Vehicle Development
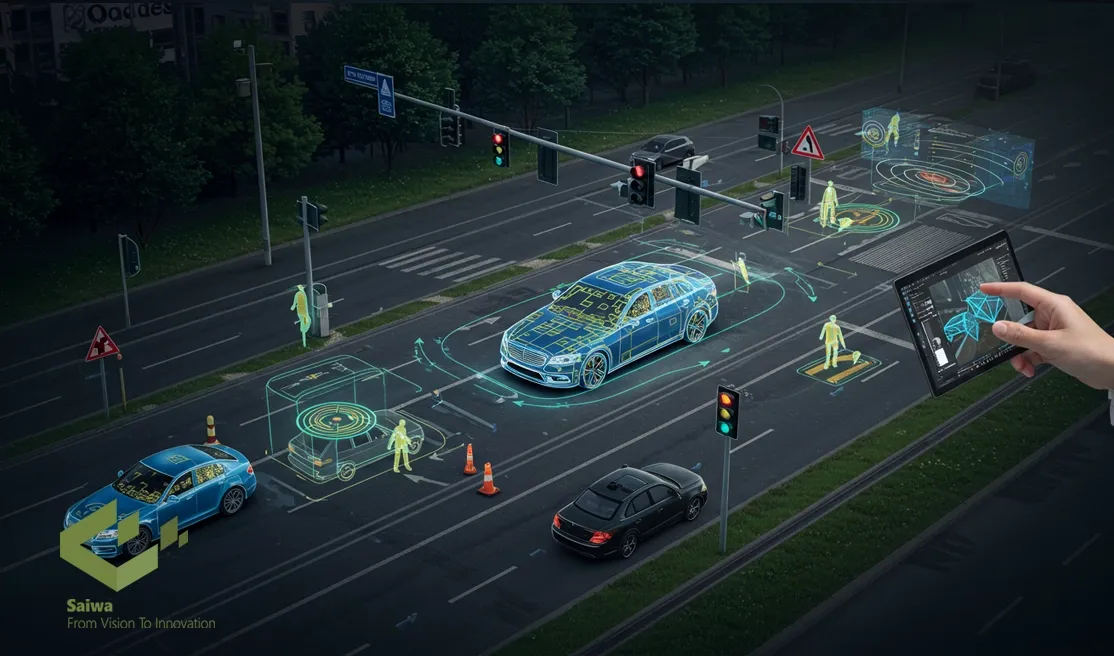
Leveraging specialized data annotation services offers several advantages for autonomous vehicle development.
Enhancing Accuracy and Safety: High-quality data annotation directly contributes to the accuracy and safety of autonomous vehicles. Accurate annotations ensure that the vehicle's perception systems can reliably identify and classify objects, leading to safer and more reliable driving decisions.
Cost and Time Efficiency: Outsourcing data annotation to specialized services can be more cost-effective and time-efficient than building and managing an in-house annotation team. These services can handle large volumes of data quickly and efficiently, freeing up internal resources to focus on other aspects of AV development.
Scalability: Data annotation services can easily scale their operations to meet the growing demands of autonomous vehicle development. As the volume of data required for training AV models increases, these services can readily adapt to provide the necessary annotation capacity.
Technologies Driving Data Annotation for Autonomous Vehicles
Several technologies are driving advancements in data annotation for autonomous vehicles.
Automation Tools and AI Support for Annotation
Automated annotation tools and AI-powered assistance are transforming the data annotation landscape for autonomous vehicles. These tools leverage machine learning algorithms to pre-label data, suggest annotations, and identify potential errors, significantly reducing the manual effort required.
They can automatically recognize and label objects, lane markings, and other features, accelerating the annotation process and improving efficiency. AI-powered tools can also learn from human annotators, further refining their accuracy and effectiveness over time. This automation is crucial for handling the massive datasets required for training AV models.
Quality Control Processes
Robust quality control processes are essential for ensuring the accuracy and consistency of annotated data used in autonomous vehicle development. These processes involve rigorous checks and validations at various stages of the annotation pipeline.
Automated checks can identify inconsistencies and potential errors, while human reviewers provide expert oversight and ensure adherence to annotation guidelines. Comprehensive quality control processes are crucial for maintaining the integrity of the training data and ensuring the reliability of AV models.
Collaboration with Specialists and Third-Party Services
Collaboration with specialists and third-party annotation services provides access to valuable expertise and specialized tools, enhancing the quality and efficiency of the annotation process. These services often employ experienced annotators with domain-specific knowledge, ensuring accurate and consistent annotations.
They also have access to advanced annotation platforms and tools, streamlining the annotation workflow and improving productivity. Furthermore, collaboration with third-party services can provide access to diverse datasets, enhancing the robustness and generalizability of AV models.
Challenges Encountered in Data Annotation for Autonomous Vehicles
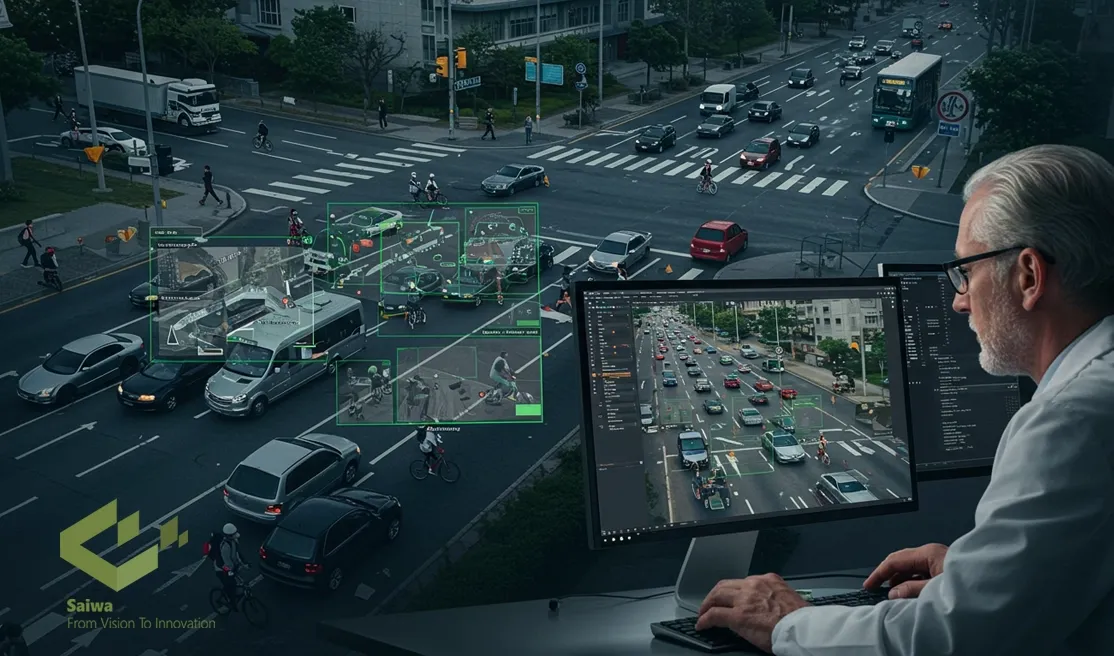
Despite its importance, data annotation for autonomous vehicles faces several significant challenges. These challenges range from managing large teams of annotators to ensuring consistent data quality and addressing privacy concerns. Overcoming these challenges is crucial for realizing the full potential of autonomous driving technology.
A Large Team of Annotators
The vast amounts of data required for AV development often necessitate a large team of annotators, which can be challenging to manage and coordinate. Ensuring consistent annotation quality across a large team requires clear guidelines, thorough training, and effective communication. Managing the workflow and tracking progress can also be complex, requiring specialized project management tools and strategies.
Selecting the Right Annotation Tool
Choosing the appropriate annotation tool is crucial for efficient and accurate data annotation. Different tools offer various features and functionalities, and selecting the right tool depends on the specific requirements of the project. Factors to consider include the type of data being annotated, the desired annotation format, the level of automation required, and the budget.
Consistent Data Quality
Maintaining consistent data quality across a large team of annotators can be a significant challenge. Variations in annotation styles and interpretations can introduce inconsistencies in the data, which can negatively impact the performance of AV models. Establishing clear annotation guidelines, providing thorough training, and implementing quality control checks are crucial for mitigating this challenge.
Privacy and Security Concerns
Data used for training AV models often contains sensitive information, such as images of people and locations. Protecting the privacy and security of this data is paramount. Implementing robust data governance policies, anonymizing data where possible, and using secure data storage and transfer methods are essential for addressing privacy and security concerns.
Cost Escalation
The cost of data annotation can escalate quickly, especially for large-scale projects. The need for large teams of annotators, specialized tools, and rigorous quality control processes can contribute to rising costs. Managing costs effectively requires careful planning, resource allocation, and exploring cost-effective annotation strategies, such as leveraging automation and outsourcing to specialized services.
Conclusion
Data annotation is an integral part of developing safe and reliable autonomous vehicles. It provides the foundation upon which these vehicles perceive, interpret, and interact with the world. While challenges remain, ongoing advancements in annotation technologies and methodologies are continuously improving the efficiency and accuracy of the annotation process. As the field of autonomous driving continues to evolve, data annotation will remain a critical driver of innovation, paving the way for a future of safer and more intelligent transportation.