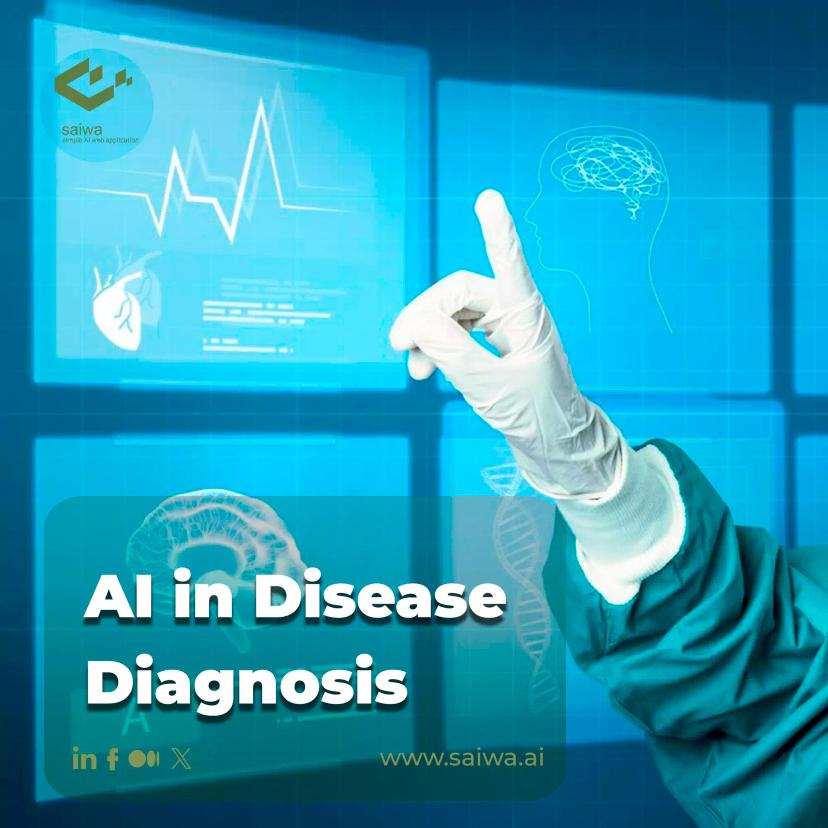
Artificial Intelligence in Disease Diagnosis
Accurate and timely diagnosis of diseases is crucial for effective treatment and management of patients. Artificial intelligence (AI) advances are providing new opportunities to improve disease diagnosis using sophisticated algorithms and large datasets. AI has the potential to revolutionize medical imaging, analyze complex physiological data, and integrate information from diverse sources to support clinical decision-making. However, there are also challenges and ethical considerations that must be addressed as Artificial Intelligence in disease diagnosis is developed and implemented.
Importance of Accurate Disease Diagnosis
An accurate diagnosis is the critical first step in caring for any patient. However, studies show that diagnostic errors affect approximately 12 million U.S. adults annually. Misdiagnosis can lead to inappropriate, delayed or no treatment, resulting in poor outcomes and higher costs. Diagnostic errors are estimated to contribute to approximately 10% of patient deaths and 17% of adverse hospital events.
Timely and accurate diagnosis allows for early intervention to cure or better manage disease, halt progression, prevent complications, and reduce mortality. Accurate diagnosis also avoids unnecessary tests, invasive procedures, and patient anxiety. At a system level, reducing diagnostic errors can significantly reduce healthcare costs.
AI has the potential to transform medical diagnosis by augmenting clinicians with data-driven insights, reducing human error and bias, and integrating information from multiple sources. AI can analyze much larger amounts of patient data to discover patterns that can aid in diagnosis. It can also provide decision support in complex cases.
Read Also: The Future of Healthcare | Revolutionizing AI in Healthcare
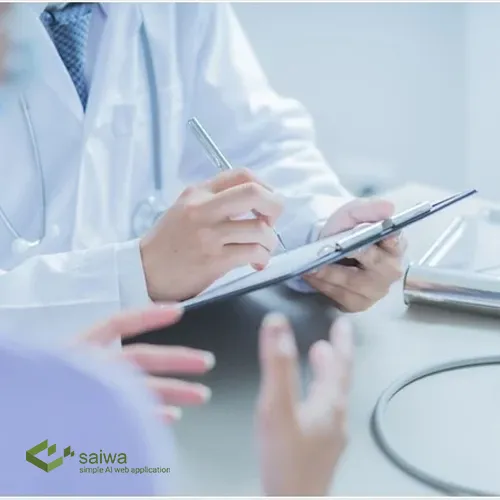
Understanding Artificial Intelligence in disease diagnosis
AI refers to computer systems that are capable of tasks that typically require human intelligence, such as visual perception, speech recognition, and decision-making. Artificial Intelligence in disease diagnosis includes techniques such as machine learning, neural networks, natural language processing, and computer vision.
In healthcare, AI can be applied to various clinical tasks:
Medical Imaging: AI can analyze medical images such as X-rays, CT scans, MRI, and microscopy images to detect abnormalities and patterns indicative of disease.
Patient Data Analysis: Electronic Health Record (EHR) data of patients can be mined using AI to uncover trends and risk factors for disease.
Diagnostic Decision Support: By combining patient test reports, medical history, and symptoms, AI systems can provide diagnostic recommendations to clinicians.
Early Warning Systems: AI can monitor multiple data streams from ICU equipment and patient vitals to predict adverse events or deterioration.
Genomics: Large genomic datasets can be rapidly analyzed by AI to correlate genes, mutations, and biomarkers with disease risks and drug responses.
Read Also: The New Age of Imaging | Exploring AI in Radiology
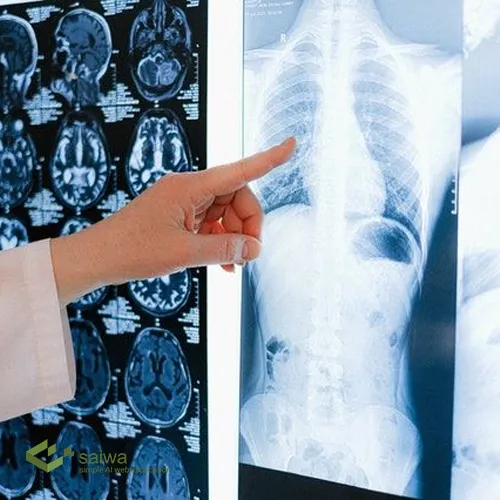
Role of Medical Imaging in Disease Diagnosis
Medical imaging technologies such as X-ray, CT, MRI, and ultrasound play a pivotal role in disease screening, initial diagnosis, and follow-up examinations. Interpreting these complex images relies extensively on the visual expertise of radiologists and clinicians. However, significant rates of imaging diagnostic errors have been reported.
AI and deep learning are poised to transform medical imaging by automating the detection of abnormalities, aiding interpretation, and improving accuracy. Some applications include:
Identifying pathology in images (e.g., tumours, fractures, pneumonia)
Detecting lesions and micro-calcifications in mammograms
Classifying skin lesions from dermoscopic images
Spotting diabetic retinopathy abnormalities in retinal fundus photographs
Quantifying changes in follow-up scans to monitor disease progression or response to therapy
Large annotated datasets are required to train AI algorithms to detect patterns in medical images. Multi-modal frameworks that combine text reports with images can also improve performance. AI in disease diagnosis field will not replace radiologists but rather work alongside them to spot hard-to-detect abnormalities and reduce errors.
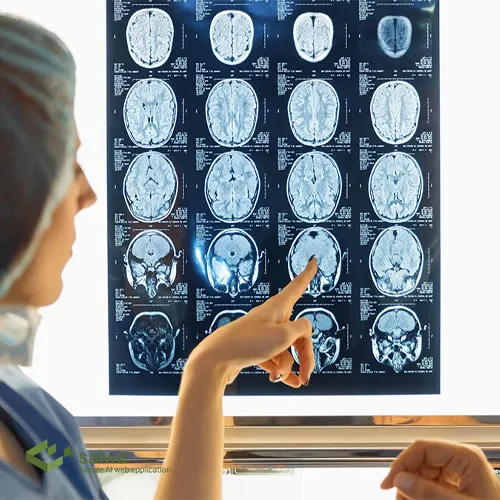
AI Techniques in Disease Diagnosis
Some of the main AI in disease diagnosis techniques include:
Machine Learning:
Algorithms like support vector machines, random forests, and neural networks can be trained to identify disease patterns in medical data. For example, machine learning can integrate patient symptoms, medical history, and test results to predict likely diagnoses.
Deep Learning:
Deep neural networks have revolutionized image and speech recognition. In healthcare, convolutional neural networks are extensively used to analyze 2D medical images. Recurrent neural networks can process sequential data like physiologic waveforms.
Natural language processing (NLP):
NLP techniques enable computers to understand clinical text notes. This allows key symptoms, risk factors, and diagnostic details to be extracted from unstructured electronic records.
Computer Vision:
In addition to processing medical images, computer vision in healthcare can also analyze video feeds during procedures or subjective visual data like skin lesions to support diagnostics.
Big Data and Artificial Intelligence in disease diagnosis
The application of AI to diagnose diseases has been enabled by the explosion of complex medical data. Some key data sources leveraged by AI include:
Electronic Health Records (EHRs): Longitudinal EHR data containing symptoms, history, diagnostic test results, prescriptions, etc. can be mined using AI to uncover trends.
Medical Imaging Databases: Large curated repositories of labeled medical images are used to train computer vision algorithms. ImageNet, PADChest, and DeepLesion are some examples.
Genomics Data: High-throughput sequencing has generated vast genomic datasets linking genes and mutations to disease risks that AI can rapidly analyze.
Wearables Data: Consumer wearable devices provide continuous streams of physiological signals. Fed into AI algorithms, these might provide early diagnosis.
Multimodal Data: AI systems combining both images and text data have more contextual information and have achieved better diagnostic performance.
However, issues around access, privacy, curation, biases, and regulatory compliance around these datasets present challenges.
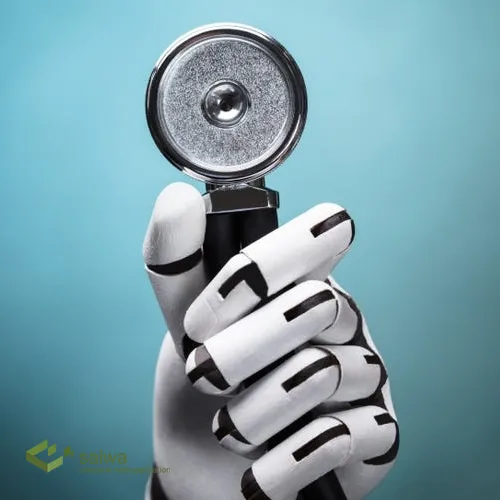
Challenges and Ethical Considerations
While Artificial Intelligence in disease diagnosis has promising diagnostic applications, integrating it into clinical practice also raises some challenges and ethical considerations:
Potential bias: Training datasets that are small or skewed may propagate biases and affect model performance in underrepresented patient groups.
Privacy risks: AI systems require large amounts of patient data, which raises privacy and data security concerns that must be addressed.
Interpretability: Deep learning models are complex black boxes and lack interpretability. This can impede medical acceptance even if performance is good.
Medico-legal issues: Liability in cases of misdiagnosis using AI must be addressed through approvals and regulations.
Job disruption: AI may change radiology workflows and affect jobs, leading to physician resistance. Change management is vital.
Patient acceptance: Lack of transparency around AI may lead to reduced patient trust and acceptance. Patient perspectives must be considered.
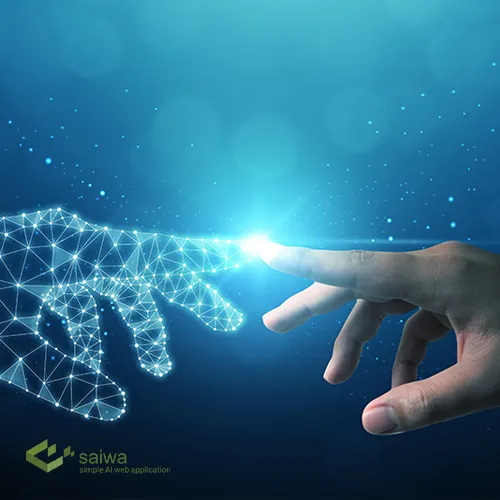
Conclusion
AI has significant potential to transform disease diagnosis by improving accuracy, supporting clinical decision-making, and reducing errors. It can extract insights from diverse and large-scale medical data. However, thoughtful development and regulation are needed to address risks related to bias, privacy, and interpretability of Artificial Intelligence in disease diagnosis systems. A collaborative approach that includes clinician and patient perspectives will enable the translation of these emerging technologies into patient benefit.