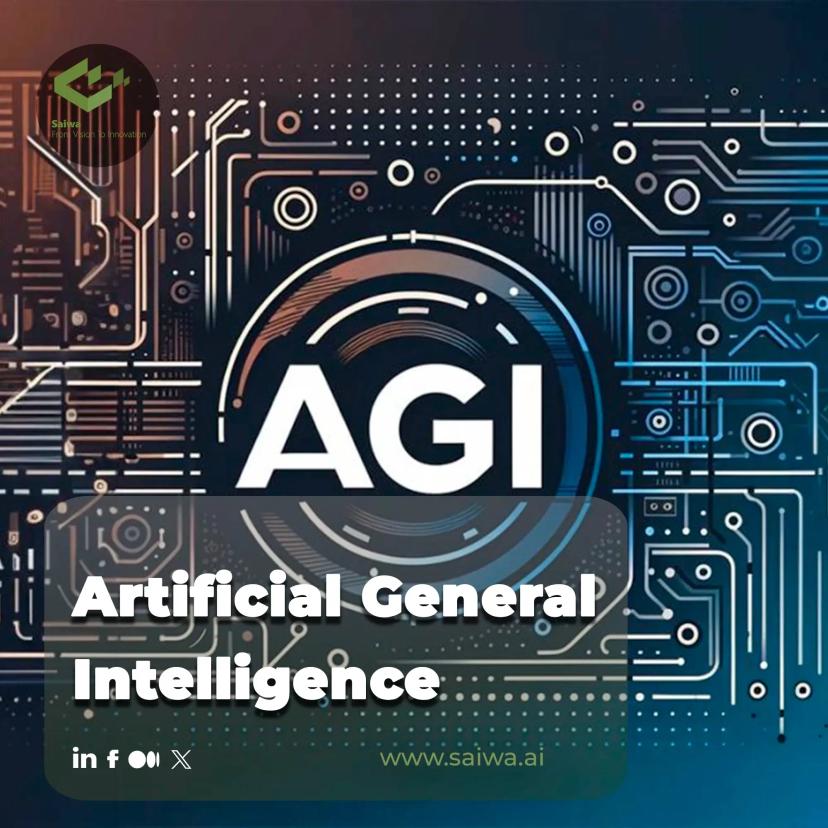
Demystifying Artificial General Intelligence (AGI) | Key Concepts and Challenges
The concept of artificial intelligence (AI) has evolved from a futuristic notion to a pervasive aspect of contemporary life. Its applications have become integral to numerous aspects of daily living, including the provision of personalized recommendations and the delivery of medical diagnoses. Nevertheless, these applications, impressive as they are, represent merely the initial stage of development.
One can envisage a future in which machines are not merely capable of performing specific tasks, but rather possess the capacity to understand, learn, and adapt to any intellectual challenge in a manner analogous to that of a human. This vision is encapsulated in the concept of artificial general intelligence (AGI).
This article will examine the theoretical foundations, key components, and potential implications of AGI, exploring both the promises and challenges that lie ahead in this ambitious quest.
What is Artificial General Intelligence (AGI)?
Artificial General Intelligence (AGI) refers to a type of artificial intelligence that possesses the ability to understand, learn, and apply knowledge across a wide range of tasks and domains, similar to the cognitive abilities of humans. Unlike narrow AI, which is designed to perform specific tasks (such as language translation or image recognition), AGI aims to replicate human intelligence's generality and versatility.
Theoretical Foundations
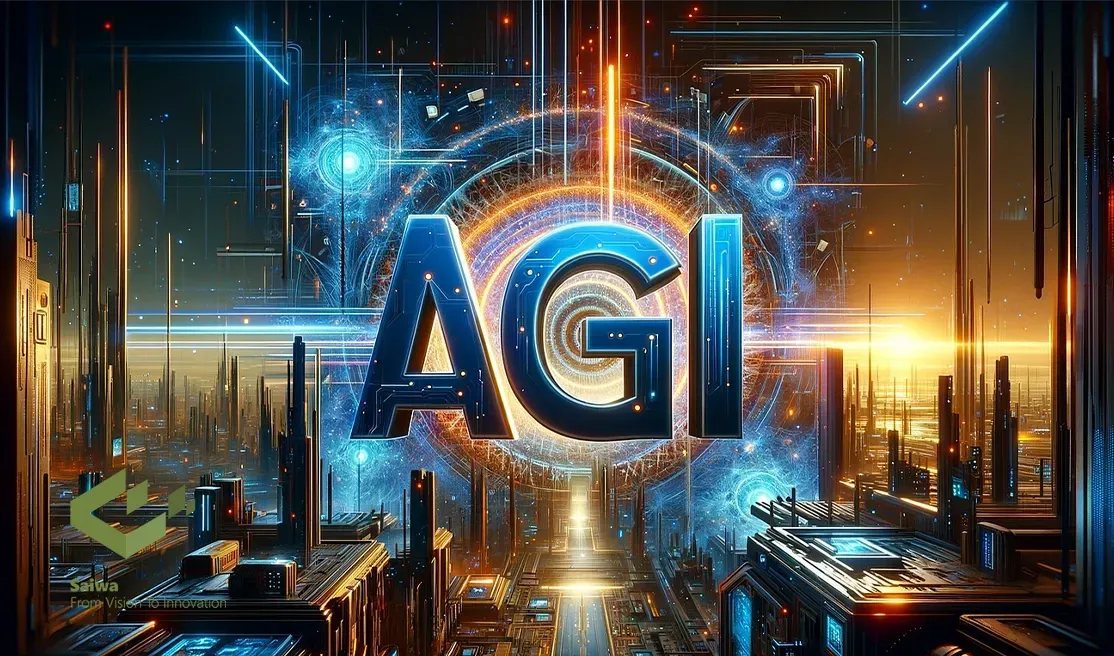
The pursuit of AGI is deeply intertwined with cognitive science, neuroscience, and philosophy. It draws inspiration from the human brain, aiming to decipher the principles underlying general intelligence and replicate them in machines.
Computational Theory of Mind
This theory posits that the human mind operates on computational principles, processing information through algorithms and representations. AGI research often leverages this framework, attempting to create artificial systems capable of similar computational processes.
Neuroscience and Connectomics
Understanding the structure and function of the human brain, particularly the intricate network of neurons and synapses, provides valuable insights for AGI development. Some approaches aim to mimic the brain's architecture, while others seek inspiration from its learning and adaptation mechanisms.
Philosophy of Mind
Philosophical inquiries into the nature of consciousness, self-awareness, and intelligence inform the ethical and societal implications of AGI. As we strive to create machines with human-level intelligence, grappling with these philosophical questions becomes increasingly crucial.
What is the difference between artificial intelligence and artificial general intelligence?
While the terms artificial intelligence (AI) and artificial general intelligence (AGI) are often used interchangeably, they represent distinct concepts within the realm of computer science.
Artificial Intelligence (AI)
Encompasses systems designed to perform specific tasks that typically require human intelligence. These systems excel within defined domains, such as playing chess, recognizing images, or translating languages. However, their capabilities are limited to the specific tasks they are trained for.
Read more: The Synergy of Artificial Intelligence and Human Intelligence | Unveiling the Future
Artificial General Intelligence (AGI)
Refers to a hypothetical type of AI that possesses human-level cognitive abilities across a wide range of domains. An AGI system would not be limited to pre-programmed tasks but could learn, adapt, and solve problems in diverse and novel situations, much like a human being.
The key distinction lies in the generality of intelligence. AI systems exhibit specialized intelligence, excelling at specific tasks, while AGI aims for generalized intelligence, capable of adapting to diverse challenges and learning new skills without explicit programming.
Key Components of AGI Systems
Developing AGI systems necessitates integrating various components that collectively contribute to their general intelligence capabilities:
Learning and Adaptation
Continuous Learning: AGI systems should not be limited to their initial training data but should continuously learn and adapt from new experiences and information, much like humans do throughout their lives.
Transfer Learning: The ability to apply knowledge gained in one domain to solve problems in different but related domains is crucial for general intelligence. AGI systems should be able to transfer learning across diverse tasks and environments.
Unsupervised and Reinforcement Learning: While supervised learning relies on labeled data, AGI systems should be capable of unsupervised learning, extracting patterns and knowledge from unlabeled data, and reinforcement learning, learning through trial and error based on rewards and penalties.
Natural Language Processing and Understanding
Natural Language Understanding: Comprehending the nuances of human language, including semantics, context, and implied meaning, is essential for AGI systems to interact with humans effectively.
Dialogue and Discourse Management: Engaging in meaningful conversations, understanding turn-taking, and maintaining coherence over extended dialogues are crucial aspects of natural language interaction.
Common Sense Reasoning: Human communication relies heavily on common sense knowledge and assumptions. AGI systems need to develop a robust understanding of common sense to interact naturally and effectively with humans.
Problem-Solving and Decision-Making
Planning and Reasoning: AGI systems should be capable of setting goals, planning actions to achieve those goals, and adapting their plans based on changing circumstances.
Causal Reasoning: Understanding cause-and-effect relationships is essential for making informed decisions and predicting the consequences of actions.
Risk Assessment and Uncertainty Management: Real-world scenarios often involve uncertainty and risk. AGI systems need to assess risks, make decisions under uncertainty, and adapt their strategies accordingly.
Perception and Sensory Processing
Computer Vision: Interpreting and understanding visual information from the environment, much like human vision, is crucial for AGI systems to navigate and interact with the physical world.
Auditory Processing: Recognizing and interpreting sounds, including speech, music, and environmental sounds, enables AGI systems to interact with the world through auditory input.
Multimodal Integration: Combining information from multiple sensory modalities, such as vision, hearing, and touch, provides a richer understanding of the environment, similar to human perception.
Memory and Knowledge Representation
Long-Term Memory: Storing and retrieving vast amounts of information over extended periods is crucial for learning, adaptation, and knowledge accumulation.
Semantic Networks and Knowledge Graphs: Representing knowledge in structured formats, such as semantic networks or knowledge graphs, enables AGI systems to reason, make inferences, and connect different pieces of information.
Episodic Memory: Recalling past experiences and events, similar to human episodic memory, can contribute to learning, adaptation, and decision-making in novel situations.
Approaches to Developing AGI
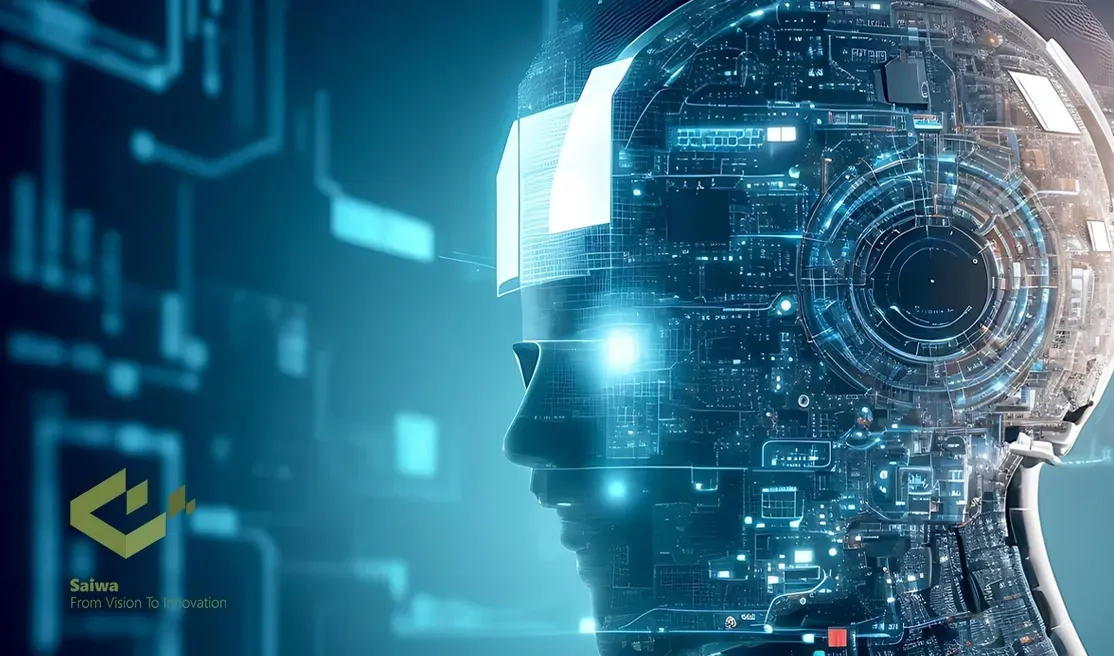
Machine Learning and Deep Learning
Deep Neural Networks: Inspired by the structure of the human brain, deep neural networks have achieved remarkable success in various AI tasks. Researchers are exploring their potential for AGI, leveraging their ability to learn complex patterns and representations from vast datasets.
Reinforcement Learning: Training agents to interact with environments and learn through trial and error, guided by rewards and penalties, shows promise for developing AGI systems capable of adapting to dynamic environments.
Evolutionary Algorithms: Mimicking the process of natural selection, evolutionary algorithms generate and evaluate generations of solutions, gradually evolving towards more intelligent systems.
Hybrid Approaches
Neuro-Symbolic AI: Combining the strengths of symbolic AI, which excels at logical reasoning and knowledge representation, with the pattern recognition capabilities of neural networks, aims to create more robust and interpretable AGI systems.
Cognitive Architectures: Inspired by cognitive science, cognitive architectures attempt to create comprehensive frameworks that integrate various cognitive functions, such as perception, memory, learning, and reasoning.
Developmental Robotics: Drawing inspiration from child development, this approach focuses on creating robots that learn and develop their cognitive abilities through interaction with the environment, much like human children do.
Brain-Inspired Computing
Neuromorphic Computing: Developing hardware that mimics the structure and function of the human brain, using artificial neurons and synapses, aims to create more energy-efficient and powerful computing platforms for AGI.
Brain-Computer Interfaces (BCIs): Establishing direct communication pathways between the brain and external devices could potentially accelerate AGI development by leveraging the brain's computational power and learning mechanisms.
Examples of Artificial General Intelligence
While true AGI remains a hypothetical concept, several research projects and systems exhibit characteristics that align with the goals of AGI:
OpenAI's GPT-3: A large language model capable of generating human-quality text, translating languages, writing different kinds of creative content, and answering your questions in an informative way. However, it lacks common sense reasoning and struggles with tasks requiring real-world understanding.
DeepMind's AlphaZero: A reinforcement learning system that mastered the games of chess, shogi, and Go without any human knowledge, demonstrating remarkable generalization capabilities within the domain of board games.
Google AI's Gato: A single transformer model capable of performing over 600 different tasks, including image captioning, robotic control, and playing Atari games, showcasing a degree of generalization across diverse domains.
Challenges and Limitations
Despite significant progress in AI, achieving AGI presents formidable challenges:
Defining and Measuring Intelligence: Lack of a universally accepted definition of intelligence makes it challenging to assess and compare the progress of AGI systems.
Common Sense Reasoning and Knowledge Representation: Endowing machines with human-like common sense reasoning and the ability to represent and reason about real-world knowledge remains a significant hurdle.
Data Efficiency and Generalization: Current AI systems often require massive amounts of data to learn effectively. AGI systems need to learn from fewer examples and generalize their knowledge to novel situations.
Explainability and Interpretability: Understanding the decision-making processes of complex AI systems is crucial for trust and accountability. However, many deep learning models lack transparency, making it difficult to interpret their reasoning.
Ethical Considerations
The development of AGI raises profound ethical considerations that require careful deliberation:
Job Displacement and Economic Impact: AGI systems could potentially automate a wide range of jobs, leading to economic disruption and the need for societal adaptation.
Bias and Fairness: AI systems can inherit and amplify biases present in their training data, potentially leading to unfair or discriminatory outcomes.
Autonomous Weapons Systems: The development of autonomous weapons systems powered by AGI raises significant ethical concerns regarding accountability, control, and the potential for unintended consequences.
Singularity and Existential Risk: Some experts speculate that AGI could lead to a technological singularity, a hypothetical point in time when AI surpasses human intelligence, potentially posing existential risks to humanity.
AGI and the Future
The pursuit of AGI holds immense potential to transform various aspects of our lives:
Scientific Discovery and Innovation: AGI systems could accelerate scientific breakthroughs by analyzing vast datasets, identifying patterns, and generating hypotheses, leading to advancements in medicine, materials science, and other fields.
Solving Complex Global Challenges: AGI could contribute to addressing pressing global issues such as climate change, poverty, and disease by optimizing resource allocation, developing sustainable solutions, and accelerating research efforts.
Enhancing Human Capabilities: AGI systems could augment human intelligence, creativity, and problem-solving abilities, leading to new forms of collaboration and innovation.
Conclusion
Artificial general intelligence represents one of the most profound frontiers in computer science, promising to revolutionize our world in ways that are difficult to anticipate. While the path to achieving AGI is fraught with significant challenges, the potential benefits—including the acceleration of scientific discovery and the resolution of global issues—are immense.
As we progress in this uncharted domain, it is imperative to address the ethical implications and strive to ensure that AGI serves the best interests of all humanity. The path towards AGI is not merely a technological undertaking; it represents a pivotal juncture in our collective future.
To secure a positive outcome, we must approach AGI development with careful consideration, ethical responsibility, and a steadfast commitment to the greater good.