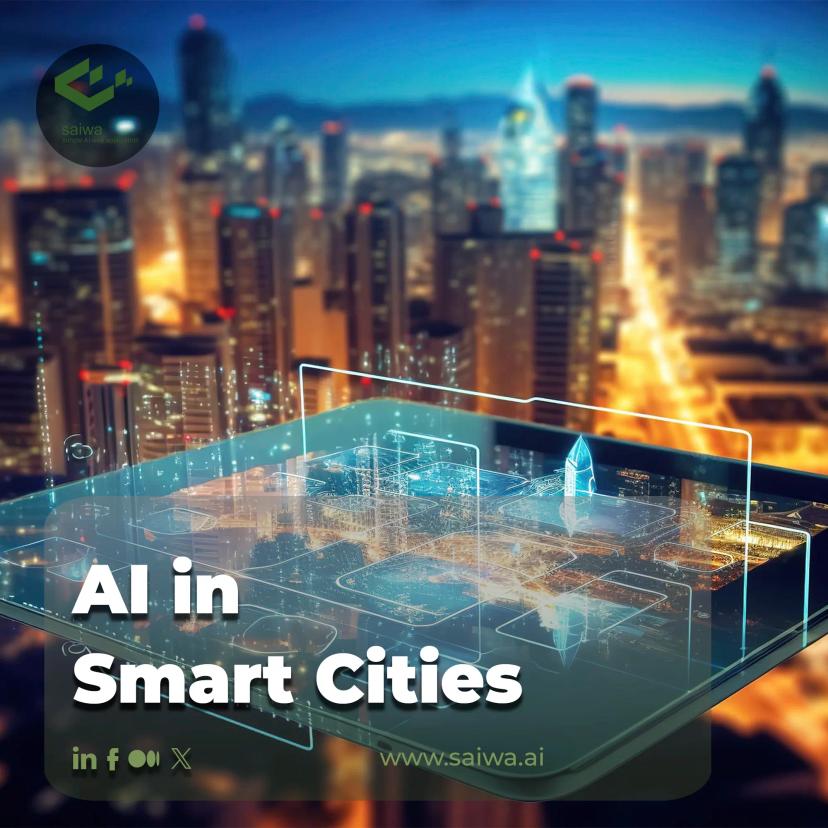
The Role of AI in Smart Cities |Transforming Urban Life
As urban populations continue to grow globally, cities are grappling with various issues, from traffic congestion to deteriorating infrastructure. Smart cities strive to improve citizens' quality of life by leveraging technology and data to provide services more efficiently. Artificial intelligence is proving to be an invaluable resource for cities to operate more intelligently and responsively.
This blog post examines the role of AI in smart cities and the significant ways artificial intelligence can revolutionize city life, including traffic management, public safety, infrastructure maintenance, sustainability, service delivery, and long-term planning. The post also addresses the challenges that cities face in embracing AI adoption.
AI applications in urban life
In today's rapidly changing city environments, the integration of AI in smart cities has become a transformative force, redefining how cities operate and improving the quality of urban life. The use of AI in urban settings has introduced a new era of innovation and efficiency, revolutionizing transportation, healthcare, energy management, and public safety.
As we explore the various aspects of AI's impact on urban living, we will discover how these smart systems are enhancing city operations while also promoting more eco-friendly, inclusive, and interconnected neighborhoods for tomorrow.
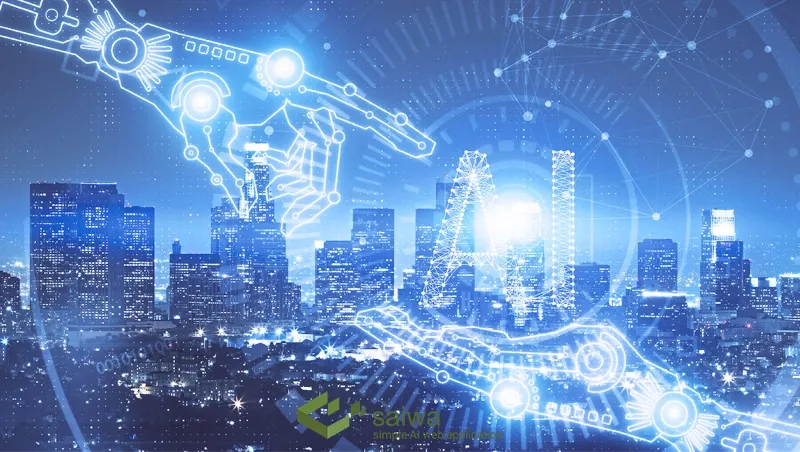
Leveraging AI to Enable Smarter Traffic Management
Growing traffic congestion results in significant economic losses for cities every year - from fuel waste and pollution to reduced worker productivity. These systems can provide valuable insights and aid in the efficient management of traffic flow, ultimately benefiting both commuters and the community. Intelligent transportation systems powered by artificial intelligence techniques, such as AI for smart cities, offer a powerful tool for cities to optimize mobility and minimize gridlock.
Computer vision algorithms can analyze real-time video feeds from thousands of traffic cameras throughout a city to detect areas of congestion. Adaptive AI traffic management agents can dynamically adjust traffic light phases and sequences to reduce bottlenecks by modifying light durations and coordination between intersections.
By continuously modeling complex variables like weather, events, day of the week, and even nearby construction schedules, AI algorithms can also forecast short-term traffic flows. This allows proactively re-routing incoming autonomous vehicles along less congested alternative paths before tie-ups materialize.
Further, anomaly detection algorithms coupled with license plate recognition can identify vehicles disobeying traffic rules like speeding, illegal turns, or driving in restricted zones. This enables real-time automated enforcement to improve compliance. Drawing on rich historical datasets, AI toll pricing models can also adjust rates based on projected demand to limit discretionary peak-hour traffic. Thoughtfully implemented, AI for smart cities innovations will be indispensable for maintaining fluid, optimized mobility as urbanization accelerates.
Using AI in Smart Cities to Enhance Public Safety
Another example of leveraging AI in smart cities is public safety matters. Public safety organizations like police departments and first responders are leveraging AI techniques like computer vision and predictive analytics to enhance their effectiveness. For instance, real-time video analytics algorithms can automatically analyze footage from thousands of security cameras across a city to rapidly detect crimes in progress, identify wanted criminals, detect missing children, and alert authorities far faster than traditional surveillance rooms.
This exponentially expands monitoring coverage and speed. In another example, extensive urban acoustic sensor networks coupled with AI gunshot detection models can instantly triangulate the origin of gunfire incidents based on audio signatures. By dispatching first responders to the exact GPS coordinates within seconds, this AI system is estimated to have increased victim survival rates by over 60% in some cities compared to relying only on calling the police.
Predictive policing techniques apply crime pattern analysis using statistical machine learning models trained on historical incident data. This identifies high-risk city blocks during specific hours where crimes are more likely to occur. Deploying police patrols proactively to such statistically high-risk areas during their risk windows serve as data-driven deterrence to prevent crime rather than just responding after incidents.
Further, geospatial analytics techniques can optimize the geographical placement of security resources like surveillance cameras, emergency callboxes, and police stations based on coverage considerations and proximity to past incidents. When developed responsibly with proper oversight to avoid biases, such AI systems demonstrate immense potential to enhance public safety comprehensively.
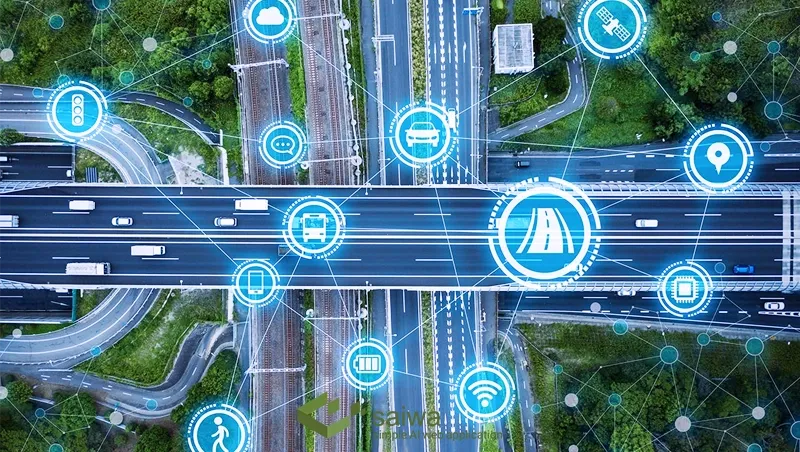
AI Predictive Maintenance to Optimize Infrastructure
Aging urban infrastructure such as roads, bridges, railways, and water pipes require vigilant monitoring and timely maintenance to provide reliable service. But strained city budgets make reactive repairs unsustainable. This is where AI-enabled predictive maintenance proves invaluable by enabling data-driven planning. For example, AI models can accurately predict potential component failures weeks or months before they apply machine learning algorithms to historical maintenance records, usage patterns, and telemetry sensor data from infrastructure assets like tunnels. This enables just-in-time maintenance that prevents catastrophic failures and costly unplanned downtime.
In water distribution systems, anomaly detection algorithms analyzing telemetry data on pressure, flow rate, pipe vibrations, and water quality characteristics can quickly pinpoint emerging leaks and contamination for rapid response before major pipe bursts occur. Further, automated computer vision analysis on live infrastructure camera feeds can complement periodic manual inspections by identifying surface cracks, spalls, and corrosion on bridges, roads, and buildings long before human inspectors notice. By enabling predictive and preventative maintenance, such AI techniques multiply the benefits of strained city infrastructure budgets.
Employing Conversational AI to Streamline Citizen Services
Municipalities are leveraging conversational AI interfaces and data analytics techniques to provide citizens access to services in a more efficient, accessible, and personalized manner. For instance, chatbots with natural language processing can automate answering common citizen queries regarding public transit schedules, utility bills, housing programs, and other frequent citizen service requests 24/7 without requiring human staffing.
Such virtual assistants provide convenient self-service, freeing city call center agents to handle more complex cases requiring human expertise and discretion. Sentiment analysis algorithms applied to public social media conversations can also help city officials identify citizen concerns and perceptions around new policies, events, or initiatives. This allows precisely targeting data-driven constituent outreach and engagement.
Intelligent smart city applications that integrate services such as transportation, utilities, and procurement through conversational AI enable easy access to city resources via voice or text. Features such as proactive, personalized notifications and recommendations allow for the customization of city services based on individual citizen needs and skills. Overall, thoughtfully implemented AI and data analytics enable the delivery of public services with greater administrative efficiency, user convenience, and outreach. However, proper safeguards must be in place to ensure full compliance with laws such as disability laws and privacy policies.
Read Also: The Role of Artificial Intelligence in Logistics
Employing AI to Drive Urban Sustainability
In addition to optimizing operations, AI innovations enable cities to pursue sustainability, efficiency, and conservation goals: Intelligent building management systems powered by machine learning can minimize energy waste in public buildings by optimally controlling lighting, heating, cooling, and ventilation levels based on predicted occupancy patterns.
In addition, by predicting localized waste generation rates based on demographics, city events, and consumer behavior models, AI-powered route optimization algorithms for waste collection vehicles can minimize mileage, fuel consumption, vehicle wear and tear, and traffic disruption. AI also enables environmental benefits-high-resolution, hyper-local air pollution simulation helps city planners strategically locate green spaces, bike lanes, and public transit to maximize communities' access to clean air. Finally, in large urban EV charging station networks, anomaly detection algorithms help quickly identify failing power relays, credit card readers, and charging stacks to keep stations operational and maximize EV uptime. Overall, AI innovations in smart infrastructure, transportation, and energy management are enabling growing cities to maintain sustainable, green, and efficient operations.
Informing Urban Planning Decisions Via AI
Urban planning teams make long-term, large-scale infrastructure and zoning decisions impacting cities for decades. AI in smart cities simulation, generative design and predictive analytics provide invaluable guidance by allowing data-driven decision-making:
Agent-based urban simulation models incorporating population growth rates, economic projections, geographical constraints and mobility patterns allows realistically projecting long-term housing capacity, office space and transportation infrastructure needs. This helps validate capital improvement plans to prevent under or overinvestment.
AI generative design tools allow interactively developing optimal zoning and land-use plans tailored to a city's unique priorities - balancing commercial, residential, recreational, industrial and ecological conservation needs while conforming to terrain.
Machine learning forecasting of demographic indicators helps predict population composition - allowing planners to project future demand for transit, utilities, housing types and other facilities. Lastly, predictive analytics identifies economically disadvantaged or decaying neighborhoods requiring proactive public investment into affordable housing and amenities to prevent gentrification and equitably uplift citizens.
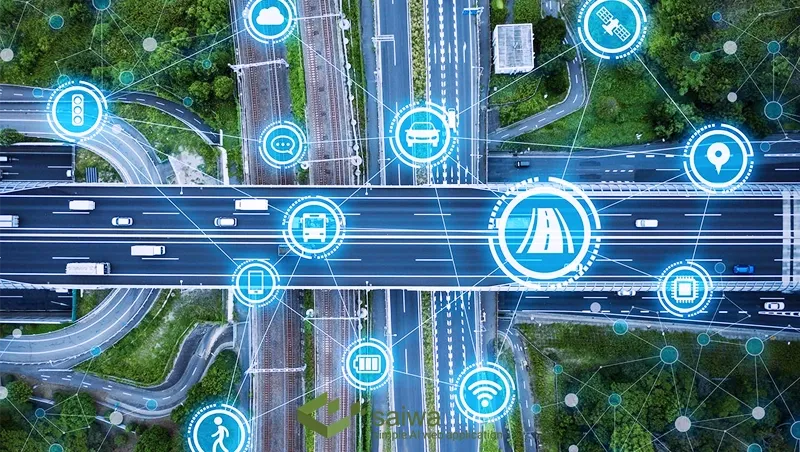
Challenges Cities Face in Mainstream AI Adoption
While promising, cities still face impediments today in comprehensively embracing power of AI in smart cities technologies:
Pervasive urban video surveillance and collection of citizen data sparks justified privacy concerns that require responsible data governance through policies enforcing stringent access controls, impact assessments, and public transparency. Technical skill gaps within municipal IT departments may inhibit procuring, developing, and maintaining complex AI systems - requiring strategic capability building through training programs and partnerships
Cybersecurity vulnerabilities arising from connecting urban infrastructure also require holistic safeguards to maintain public safety. Finally, the lack of model explainability and suitability criteria hinders compliance with public sector accountability norms - fields like AI safety and standards can help address this gap. A collaborative, ethical approach to tackling these concerns head-on will enable cities to integrate AI judiciously.
Envisioning Future Cities with Mainstream AI Adoption
As research continues advancing algorithms and urban data networks increase, mainstream AI in smart cities could enable envisioning futures where: Intelligent assistants provide citizens personalized access to municipal services via natural conversations unconstrained by apps and outdated phone menu trees.
Shared public resources like parking spots, EV chargers, and computers at schools/libraries are dynamically optimized based on real-time demand to maximize asset utilization. Lastly, comprehensive public sentiment analytics, predictive analytics, and participatory budgeting could enable more responsive, transparent, and participatory data-driven governance. By proactively shaping innovation trajectories through partnerships, cities worldwide have an opportunity to uplift citizen welfare through purpose-driven AI assimilation.
Conclusion
Thoughtfully designed AI solutions have immense potential to help cities overcome mounting urban challenges – from mobility to infrastructure maintenance and service delivery to sustainability. But pragmatic adoption balancing benefits and ethical risks is imperative to unlock AI’s true quality-of-life enhancing powers. With wise implementation and an emphasis on human-centric AI in smart cities development, cities can leverage these emerging tools to create safe, efficient, and equitable living environments even as populations swell. Technological innovation must serve public values and priorities to nurture truly smart and humane cities.