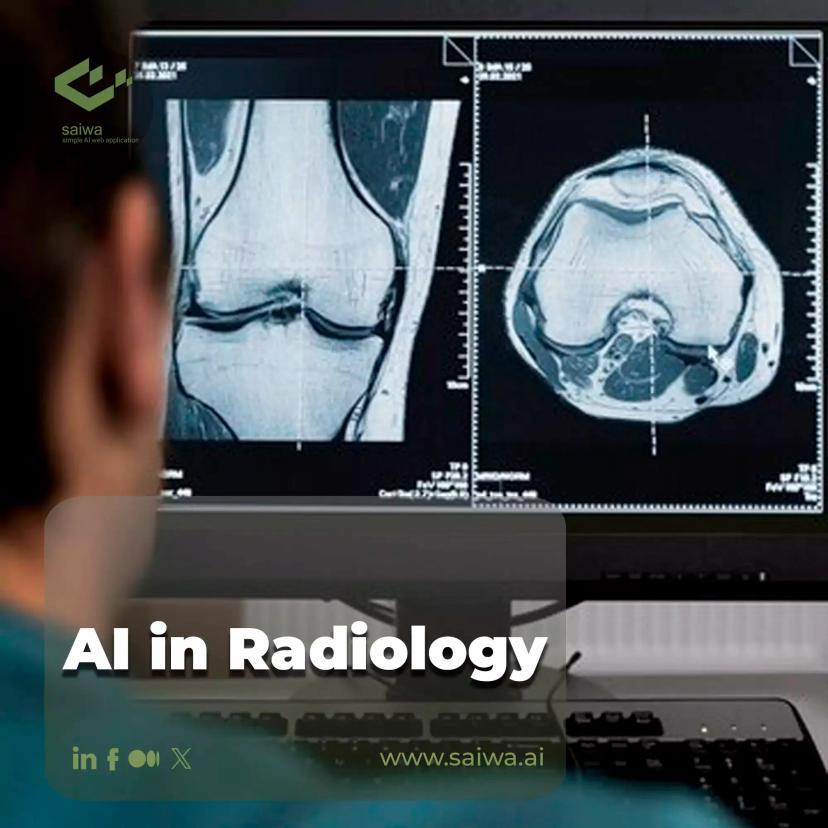
The New Age of Imaging | Exploring AI in Radiology
Radiology, the branch of medicine dealing with medical imaging for disease diagnosis, has undergone major workflow transformations with the emergence of artificial intelligence (AI) techniques like computer vision and deep learning. AI is automating tedious tasks, augmenting radiologists' capabilities, and uncovering novel insights from medical imaging data. This blog post explores the applications of AI in radiology workflow and techniques powering AI innovation. Tangible benefits for clinicians and patients, challenges hampering adoption, and the tremendous potential for AI to reshape radiology into a more efficient, accurate, and patient-centric field. Read Also: The Future of Healthcare | Revolutionizing AI in Healthcare
What is AI in Radiology?
AI in radiology refers to developing and applying artificial intelligence technologies to enhance multiple aspects of medical imaging workflow and analysis for purposes ranging from diagnosis to treatment planning and predictive analytics. This encompasses a wide spectrum of technologies, including deep learning approaches like convolutional neural networks for automated segmentation, classification, and processing of medical images. It also includes applying AI for optimizing radiology workflows, generating quantitative imaging biomarkers, providing clinical decision support, extracting insights from radiology reports using natural language processing, and discovering new correlations by data mining large repositories of medical imaging data.
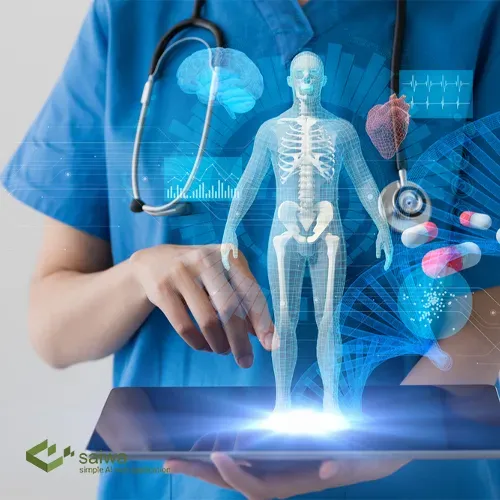
Why is AI Important for Radiology?
There are several key reasons why AI has the potential to transform radiology:
Automate routine diagnoses
AI can automate routine diagnostic workflow tasks like triaging studies, pre-analyzing images, detecting abnormalities, sorting exams, annotating findings, tracking longitudinal cases, and monitoring report turnaround times. By automating such repetitive tasks, AI enables radiologists to focus their precious time on higher-value activities like interacting with patients, complex diagnoses, and quality improvement.
Using AI Tools
AI decision support tools provide radiologists with a second opinion, alerting them to potential missed findings, discrepant diagnoses, and recommended follow-up steps based on deep analysis of the images and patient health records. Such AI assistance can boost diagnostic accuracy and standardization.
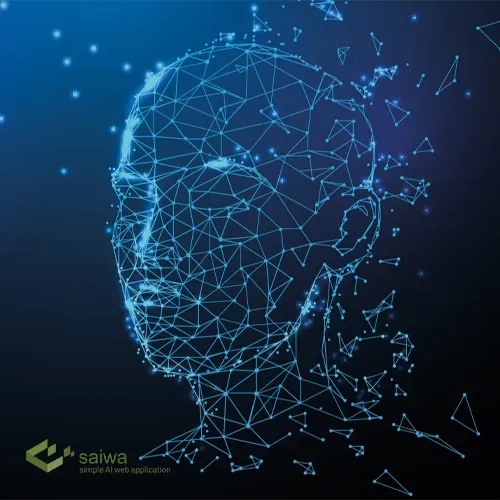
Data Mining Techniques
Data mining techniques allow extracting new insights and discoveries from vast amounts of retrospective data in the form of imaging studies, radiology reports, lab tests, clinical notes, etc. Much of this data is currently underutilized.
Optimize Utilization Resources
AI has the potential to optimize the utilization of radiology resources like scan equipment, clinical staff, radiologist time slots, and prioritization of emergent cases over routine studies by applying operational analytics - this improves patient experiences by reducing waiting times.
Novel AI Applications
Novel AI in radiology applications like image reconstruction and enhancement synthesizes new data to improve diagnosis from incomplete or sparse image data sets - this enables scenarios like lower-radiation CT scanning.
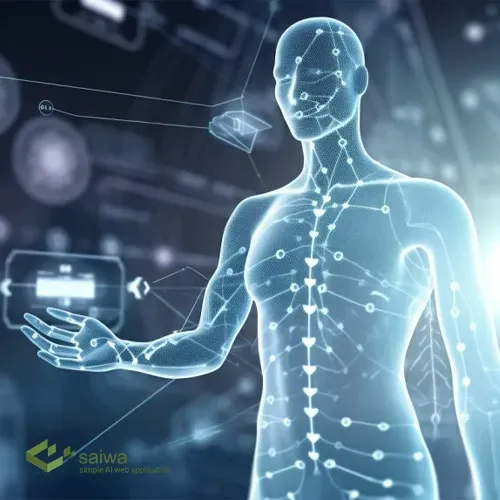
Accessibility
Cloud-based AI diagnostic assistance democratizes access to expert-level imaging interpretation for remote hospitals and underserved communities. AI also opens new dimensions like quantifying imaging biomarkers invisible to the human eye but valuable for precision diagnosis.
Applications of AI in Radiology
Some major application areas of AI transforming radiology include:
Image Analysis
AI algorithms excel at automating the initial analysis of medical images to identify areas for further investigation by radiologists. For instance, deep learning models can accurately detect lesions, tumors, fractures, and other abnormalities in modalities ranging from X-rays to MRI and CT scans. AI techniques also enable rapidly sorting medical images by properties like modality, body part, orientation, etc. This allows for prioritizing and flagging actionable cases.
Furthermore, AI can perform technical quality checks by detecting motion artifacts, noise, low contrast, and other issues to ensure proper visualization and diagnosis. AI systems catalyze radiology workflows by undertaking tedious annotating, sorting, flagging, and quality control tasks faster than human counterparts.
Quantitative Analytics
While radiologists manually assess medical images qualitatively, AI automation makes quantitative analytics possible by:
Automatically segmenting anatomical structures like tumors or organs and quantifying their volume, density, shape, and texture metrics precisely.
Tracking growth curves of tumors across longitudinal studies as biomarkers of therapeutic response and disease progression.
Measuring cardiac functional indicators like myocardial thickness, chamber volume, and ejection fraction from cardiac ultrasound and CT studies.
Standardizing measures prone to human subjectivity, such as scores indicating disease severity on imaging exams.
Such intelligent quantification augments clinicians' expertise and provides objective metrics to guide diagnosis and treatment decisions.
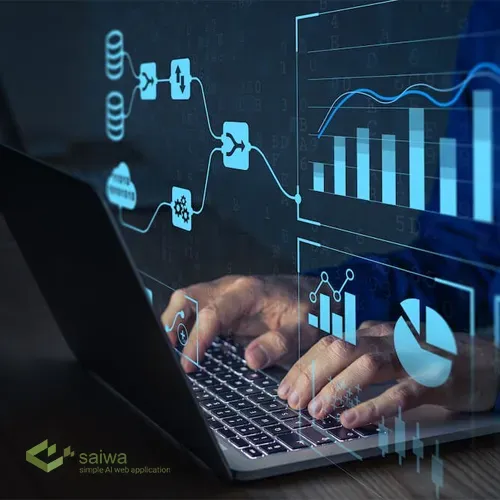
Image Reconstruction
In cases where medical images are noisy, have low resolution, or are missing essential data, AI techniques can restore and enhance images by:
Performing denoising and artifact reduction to improve clarity for diagnosis.
Inpainting missing regions in ultrasound scans obstructed by bone or air.
Super-resolution to increase the resolution of images beyond sensor limits using deep learning.
Synthesizing 3D CT scans from very sparse X-ray projections using deep neural networks. This enables lower radiation dose scanning.
Predicting full-body MRI scans from limited FOV acquisitions using generative networks to accelerate imaging.
By reconstructing optimized medical images from incomplete data, AI expands the utility of scarce imaging modalities.
Workflow Optimization
Inefficiencies like resource contention, patient backlogs, redundant exams, and equipment downtimes are commonplace in complex radiology workflows. AI optimization helps alleviate these issues and streamline radiology workflows by:
Predicting future exam volume surges based on trends so staff and resources can be proactively allocated.
Intelligently triaging and prioritizing time-sensitive cases like strokes and traumas over routine exams to minimize delays.
Ensuring optimal sequencing and de-duplication of imaging exams needed for a patient workup based on their history.
Dynamically reallocating radiologists, residents, exam rooms, and equipment across imaging modalities to balance utilization.
Proactively flagging patients requiring follow-up studies based on diagnosis history and guidelines to reduce oversight.
AI enables data-driven radiology workflow optimization, planning, and management to improve patient throughput, speed, and satisfaction.
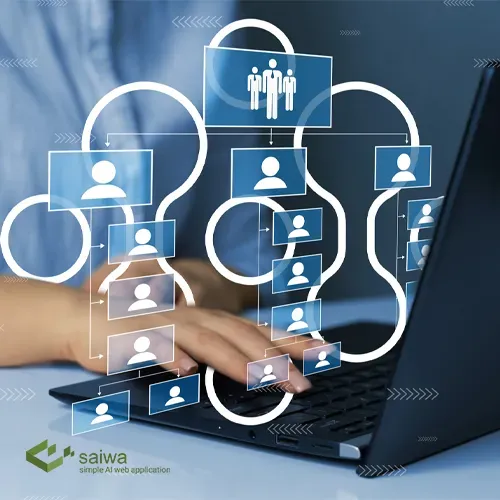
Benefits of AI in Radiology
Some major benefits that health systems can realize by thoughtfully incorporating AI in radiology tools workflows include:
Increased Diagnostic Accuracy
By automating mundane tasks like nodule detection and quantitative biomarker measurement, AI reduces errors and oversight, leading to greater diagnostic accuracy. Decision support also makes diagnosis more standardized and consistent.
Reduced Clinician Workload
AI assistance for tasks like image preprocessing, sorting, and analysis reduces radiologists' caseload volume, especially for repetitive Studies like chest X-rays. This allows them to focus on higher-value activities like interacting with patients.
Faster Turnaround Times
Automating initial triage and analysis of studies with AI allows prioritizing and accelerating time-sensitive exams like stroke CTs to enable faster treatment. Increased throughput also cuts waiting times and backlogs.
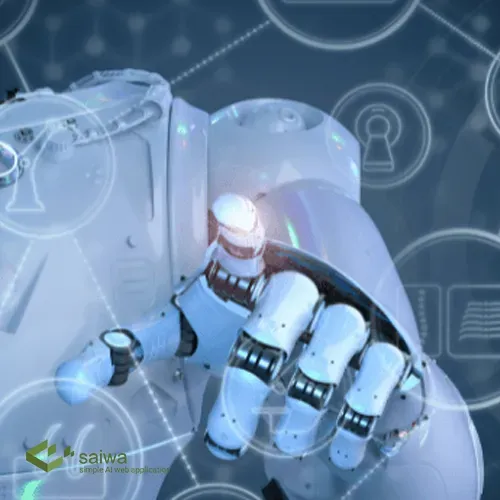
Synthesized Insights
AI can jointly analyze images from various modalities like CT, MRI, and PET scans to improve decision confidence, similar to having multiple expert opinions. This amplifies human expertise.
Retrospective Data Mining
Mining historical image archives with AI can uncover novel insights and recognize early predictors of disease unrealized previously. Such data mining augments existing knowledge.
Challenges with AI in Radiology
However, some key challenges need addressing for the successful adoption of AI in radiology:
The potential for over-reliance on AI diagnostics without human verification can compromise patient safety. AI is best used as an assistive rather than independent technology.
Inadequate clinical validation of AI algorithms through large multi-center trials before deployment in actual clinical practice can undermine acceptance. Rigorous testing is crucial.
Difficulty integrating closed proprietary hospital IT systems with modern cloud-based AI hinders widespread implementation. APIs and microservices architectures can alleviate this.
Cybersecurity vulnerabilities arise from connecting patient data across systems. Holistic cybersecurity is imperative for patient safety and confidentiality.
The lack of robust model explainability and transparency hinders clinician trust in opaque "black box" AI. Developing interpretable AI is vital.
Shortage of large annotated medical imaging datasets required for training accurate AI models, especially for rare conditions. Data sharing and synthesis techniques can expand available data.
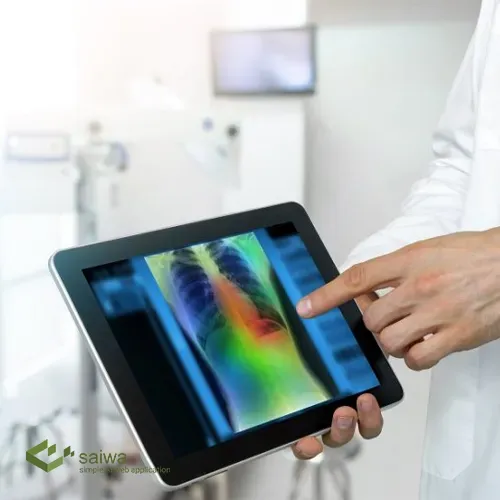
Ethics of AI in Radiology
To ensure AI enhances rather than undermines patient well-being, developers and health systems must uphold key ethical principles.
Protecting patient data privacy through responsible data governance, transparent consent processes, access controls, and maintaining anonymity during analytics.
Eliminating algorithmic biases along gender or ethnic lines and regularly auditing models for factors affecting fairness.
Extensive clinical validation of AI tools through rigorous multi-site trials before deployment. AI should enhance clinician capabilities rather than replace human judgment.
Enabling algorithmic explainability so providers clearly understand how AI models operate, their diagnostic limitations, and potential failure modes. Transparency nurtures trust.
Establishing robust cybersecurity practices safeguarding confidential patient records from potential data breaches when integrating AI across systems.
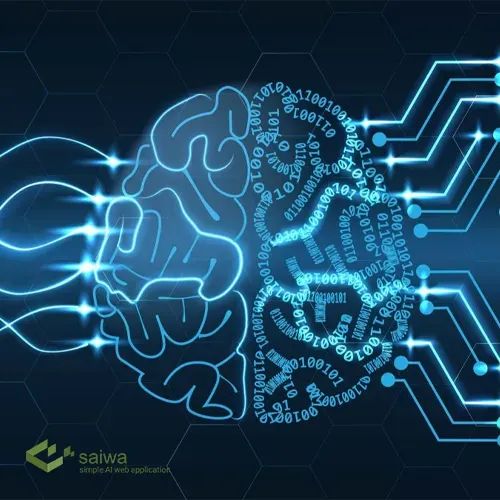
The Future of AI in Radiology
The rapid evolution of artificial intelligence techniques like deep learning and their demonstrated potential for transforming medical imaging analytics hints at a fascinating future landscape of radiology powered by AI.
Based on rapid advances, the future landscape of radiology powered by AI may look like:
Seamless embedment of AI diagnostics across imaging workflows, providing clinicians augmented intelligence at the point of care.
Democratization of specialty-level radiology expertise on-demand via cloud-based AI decision support systems.
Granular AI quantification and multi-parametric analytics are becoming integral for precision diagnosis, prognosis, and treatment planning.
Continual mining of exponentially growing medical imaging data reserves to drive new discovery and population health insights.
An expanded role for radiologists as overseers of suites of AI tools that automatically handle high-volume routine tasks.
Conclusion
AI in radiology techniques like deep learning and computer vision transform radiology by automating image quantification, optimizing workflows, providing intelligent decision support, and uncovering insights from data. Validating AI's real-world impact and addressing adoption barriers will help translate these algorithmic innovations into patient benefits. AI promises to augment radiologists' expertise, boost productivity and accuracy, enhance patient experiences, and uncover life-saving discoveries on the path to more intelligent and effective medicine.