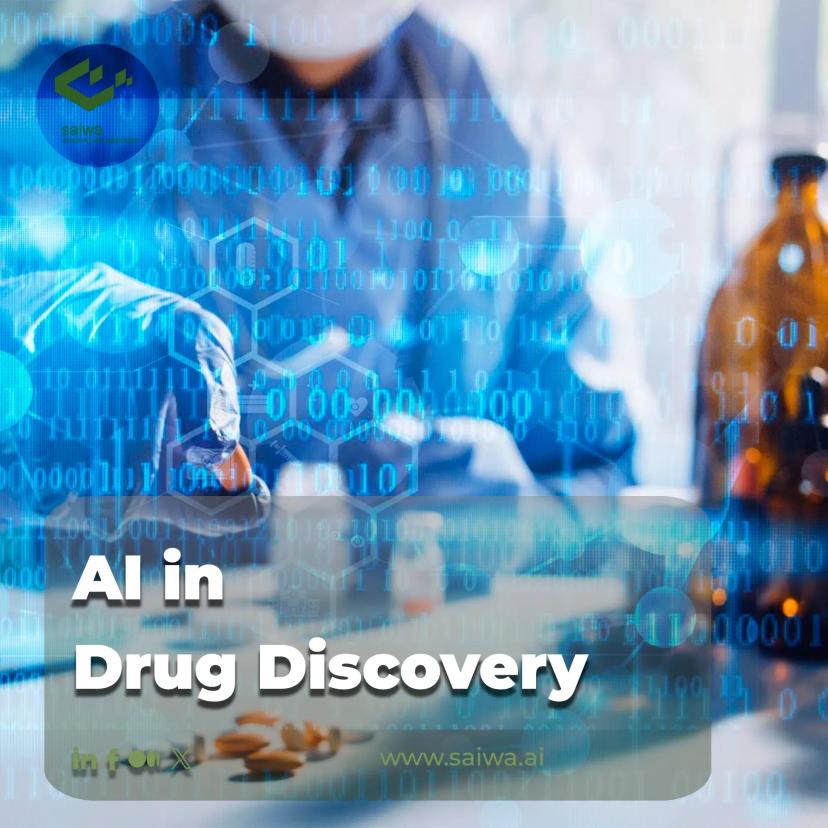
The Growing Role of AI in Drug Discovery
Bringing a new drug to market is an enormously complex and expensive process, often taking over a decade and costing upwards of $2 billion. The pharmaceutical industry faces increasing pressure to improve R&D productivity and efficiency. Artificial intelligence (AI) has recently emerged as a transformative technology to accelerate and enhance many aspects of drug discovery. From virtual screening of compound libraries to predicting clinical trial outcomes, machine learning and other AI in drug discovery techniques offer new possibilities to generate higher-quality drug candidates in less time and at lower costs.
This blog post provides an overview of core AI methods being applied in drug discovery and examples of leading companies pioneering these technologies. We discuss demonstrated benefits and current limitations, along with projections for the future impact of AI on the pharmaceutical sector. While still in its early stages, AI is poised to reshape biopharmaceutical R&D over the coming decade fundamentally.
Read Also: The Future of Healthcare | Revolutionizing AI in Healthcare
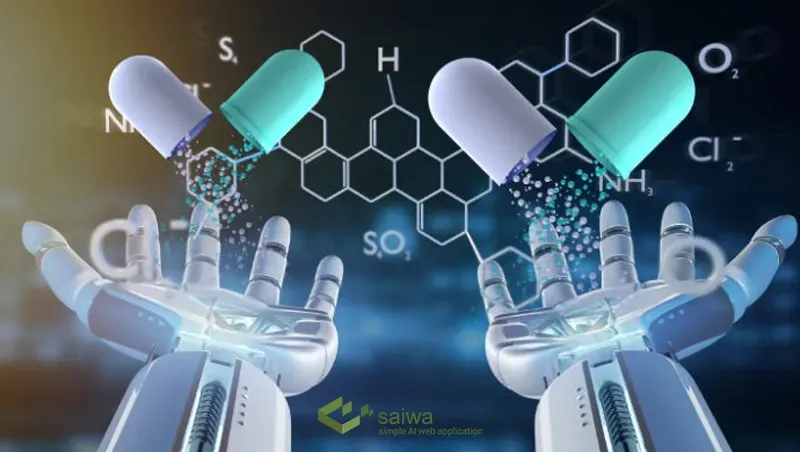
Understanding AI in Drug Discovery
Artificial intelligence refers to computer systems capable of tasks requiring human-level intelligence such as visual perception, speech recognition, and decision-making. AI includes an array of technologies from machine learning and deep learning to natural language processing and robotics. In AI in drug discovery field, AI leverages powerful computational algorithms to analyze complex biological, chemical, and medical data to generate insights for more efficient pharmaceutical R&D.
At the core of most AI applications in pharma are machine learning and machine learning as a service techniques that use statistical models trained on large datasets to make predictions or classifications without explicit programming. Popular methods include deep neural networks, random forests, support vector machines, and ensemble modeling. These algorithms uncover subtle patterns within multi-dimensional training data that can be used to predict properties and activities for new data points.
For example, deep convolutional neural networks can be trained on large collections of molecular structures labeled with their experimentally measured bioactivities. This allows the model to correlate structural features and descriptors with pharmacological behaviors. After sufficient training, the neural network can predict potential efficacy against a novel target for new molecules it has never seen before.
Other common AI methods used in drug discovery include:
Molecular modeling to simulate drug-target interactions
Virtual screening to rank or filter large libraries
Natural language processing to extract insights from text
Computer vision for histopathology image analysis
Reinforcement learning to dynamically optimize molecular structures
AI Methods and Applications in Drug Discovery
Powerful machine learning algorithms are enabling computers to tackle complex data analysis and predictive modeling challenges that were previously intractable. Approaching applicable AI in drug discovery include:
Virtual Screening:
Virtual screening leverages machine learning algorithms like deep neural networks to predict the activity of large libraries of drug-like compounds against biological targets. Models are trained on datasets of compounds with experimentally measured bioactivity data. Key steps include featurizing molecules into vector representations, training neural networks on labeled data, and predicting activity scores for novel structures. Virtual screening allows for rapidly prioritizing the most promising candidates for synthesis and testing.
Molecular Modeling:
Molecular docking is a simulation technique that models how drug compounds structurally bind to target proteins. AI in drug discovery scoring functions can predict binding affinity to rank order compounds. Docking provides insights into molecular interactions and optimization strategies. Quantum machine learning methods like those from Qulab Pharma combine quantum physics and AI to model electron distributions in atomistic drug-target systems for enhanced accuracy compared to classical docking.
Text Mining:
NLP algorithms mine scientific papers, clinical trial reports, and health records for connections between drugs, targets, side effects, and disease biomarkers. This supports hypothesis generation and drug repurposing opportunities.
Clinical Trial Optimization:
AI enables better trial design, patient recruitment, and monitoring via improved biomarker selection, cohort modeling, and outcome predictions. This can reduce costs and improve safety.
Benefits and Advantages of AI in Drug Discovery
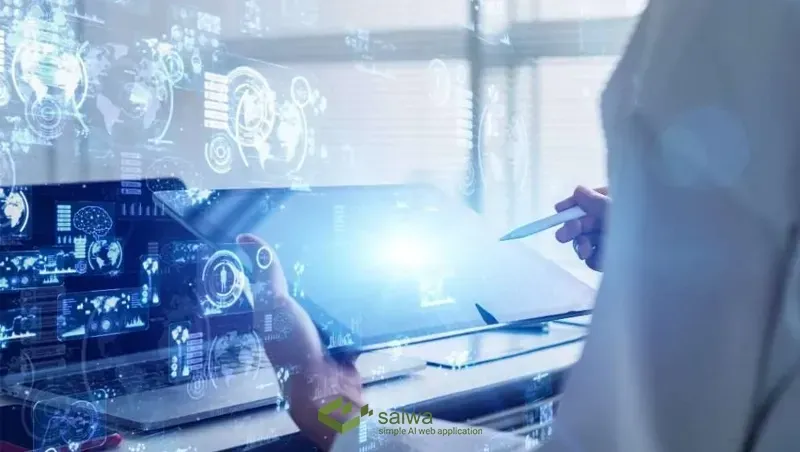
Applying AI in drug discovery throughout pharmaceutical R&D offers many potential benefits:
Faster Discovery Timelines
AI in drug discovery enables rapid in silico screening of huge chemical search spaces orders of magnitude faster than manual approaches. For example, screening millions of compounds per day using a quantum AI platform is reachable. This accelerated exploration lets researchers quickly identify the most promising candidates to test experimentally, avoiding spending months or years on ineffective molecules. AI simulation and generative models also speed up molecular optimization steps.
Lower Costs
By reducing the time and labor needed for manual molecular modeling and patchy database searches, AI analytic platforms lower drug discovery costs. Fewer late-stage experimental failures further increase savings. One estimate suggests AI could reduce costs per approved drug by up to 70%. Smaller biotech firms can access powerful cloud-based AI platforms without major infrastructure investments.
Improved Productivity
AI automation of complex workflows allows research teams to focus their time on higher-value interpretive tasks. Enhanced data integration uncovers hidden connections in siloed sources. Text mining extracts useful knowledge from unstructured scientific text. Together, these productivity gains enable teams to explore and analyze vastly more hypotheses. AI in drug discovery is projected to expand pharmaceutical R&D productivity many times over.
Novelty and Innovation
Machine learning models excel at uncovering novel hidden patterns within large, diverse datasets. This data-driven approach escapes the intrinsic biases of human specialists that can constrain creativity. Generative AI directly produces brand-new molecular structures and targets rather than just screening existing options. One study found that AI-generated molecules had more unique scaffolds than traditional medicinal chemistry. AI thus expands the solution space.
Personalization
AI analysis of huge personalized biological datasets promises to enable tailored therapies specific to individuals' genetics, microbiome, lifestyles, and phenotypes. This shift from one-size-fits-all drugs to precision medicine will be enabled by AI insights into biomarkers, side effect risks, and treatment responses for each patient.
Challenges and Limitations for AI in Drug Discovery
An ongoing challenge for AI in the pharmaceutical industry is building trust and acceptance of computer-generated drug candidates. Most researchers want to understand the reasoning behind AI predictions. However, the black-box nature of deep neural networks makes this difficult. Approaches such as integrated gradient techniques to highlight influential training features help. Nevertheless, interpretable AI remains an ongoing area of research. Extensive prospective experimental validation is essential. A semantic search engine called Euretos provides context for AI predictions. Close collaboration between AI experts and molecular scientists can build trust.
AI models built with limited or biased training data also run the risk of producing biased results or perpetuating biases. Most historical drug discovery data represent limited demographics. Ensuring that datasets cover diverse populations will be critical to avoid inequities. AI predictions may also lack novelty if models simply learn to emulate old approaches rather than identify creative solutions. Explicitly rewarding innovation during training could help overcome this limitation. Work is also underway to benchmark AI systems using standardized datasets from sources such as ChEMBL to enable transparent comparisons. Overall, combining the strengths of human and AI systems into an effective hybrid process is the ideal drug discovery workflow.
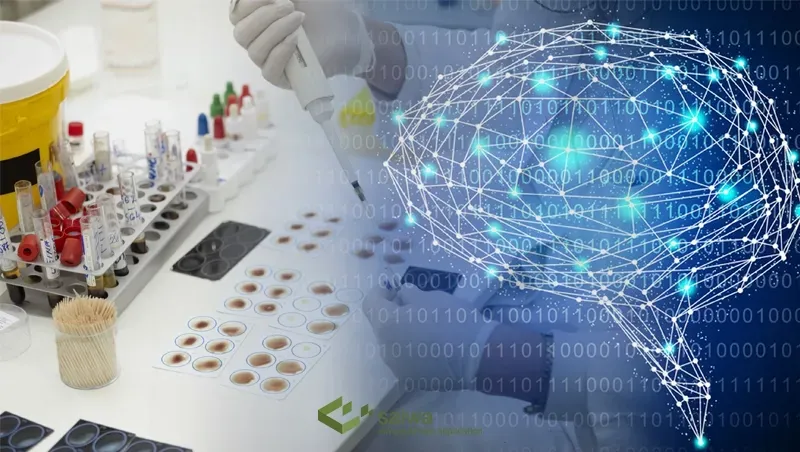
Conclusion
AI in drug discovery is rapidly becoming an integral part of modern biopharmaceutical research. Machine learning and simulation techniques are enabling more productive, efficient, and innovative drug discovery than traditional manual approaches. Although still in its infancy, AI has immense potential to generate higher-quality medical therapies while reducing costs and development timelines. This emerging technology will be truly transformative if industry leaders and researchers work together to maximize its benefits responsibly.