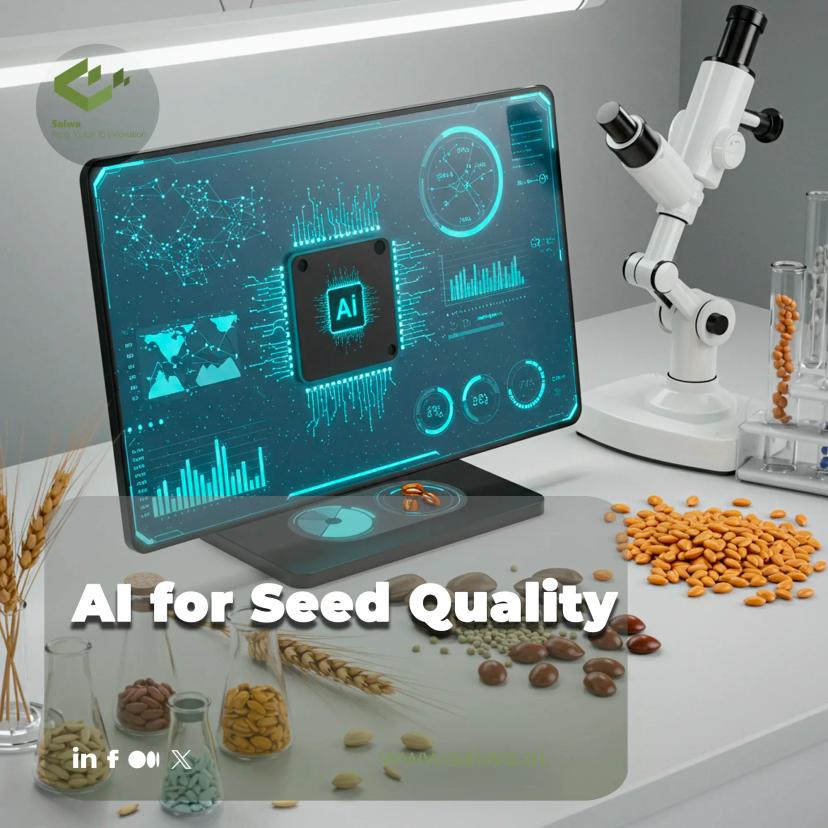
AI for Seed Quality | Smarter Farming Solutions
Seed quality plays a pivotal role in agriculture, directly influencing crop yield, plant health, and overall food security. Traditional methods of seed quality assessment often involve manual inspection, which can be time-consuming, labor-intensive, and prone to human error. The advent of artificial intelligence (AI) offers a transformative approach to seed quality evaluation, promising enhanced accuracy, efficiency, and cost-effectiveness.
Sairone, developed by Saiwa, is an AI and machine learning platform specifically designed for agricultural applications. By integrating with various drone technologies, Sairone provides critical insights into crop detection, disease identification, and nitrogen content analysis. These capabilities are particularly relevant for improving seed quality assessment, enabling accurate analysis of seed defects and diseases, and optimizing seed selection processes. With Sairone's advanced capabilities, farmers can make more informed decisions about seed quality, ensuring better crop health and yield results.
This article explores the applications of AI for seed quality testing, examining its benefits, challenges, and future prospects. We will examine how AI-based technologies are revolutionizing seed analysis, from automated imaging and predictive analysis to disease detection and genetic improvement. The implications of this technological shift are significant, potentially reshaping the seed industry and contributing to sustainable agricultural practices.
What is AI for Seed Quality?
Definition and Overview
AI for seed quality refers to the application of artificial intelligence techniques to assess and enhance the quality of seeds. This involves utilizing machine learning algorithms, computer vision, and data analytics to automate and improve various aspects of seed testing, from identifying physical defects and diseases to predicting germination rates and overall seed performance.
AI-powered systems can analyze large datasets of seed images and other relevant information to identify patterns and make predictions with a level of accuracy and speed that surpasses traditional methods. This approach allows for a more comprehensive and objective evaluation of seed quality, leading to better decision-making in seed production, selection, and planting.
Technologies Involved
Several key technologies underpin the application of AI for seed quality:
Machine Learning (ML)
ML algorithms, particularly deep learning models, are trained on vast datasets of seed images and associated quality data. These algorithms learn to recognize patterns and features indicative of seed quality, enabling automated classification and prediction. Different ML models, such as Convolutional Neural Networks (CNNs) for image analysis and Recurrent Neural Networks (RNNs) for time-series data, are employed depending on the specific application.
Computer Vision
Computer vision techniques enable computers to "see" and interpret images, mimicking the human visual system. In seed quality assessment, computer vision algorithms analyze images of seeds to identify physical characteristics, detect defects, and assess overall quality. This technology allows for rapid and automated analysis of large numbers of seeds, significantly increasing throughput compared to manual inspection.
Data Analytics
Data analytics plays a crucial role in extracting insights from the vast amounts of data generated during seed testing. Statistical methods and data visualization tools are used to identify trends, correlations, and anomalies in seed quality data, providing valuable information for decision-making. Predictive analytics can be used to forecast seed performance based on historical data and environmental factors.
How AI Improves Seed Quality Testing
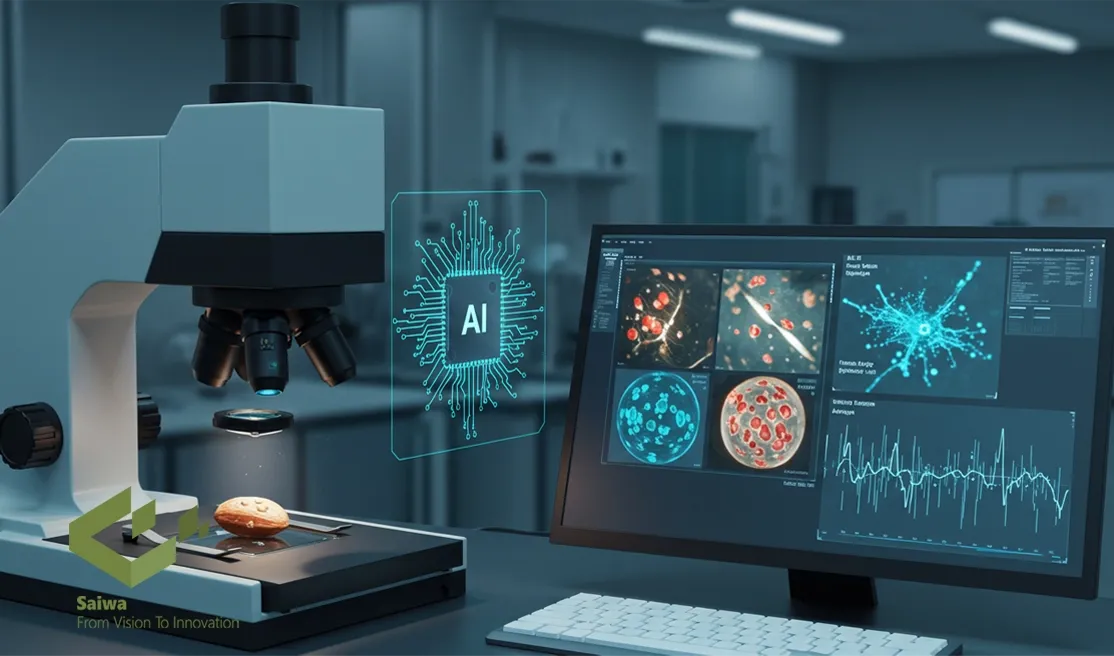
AI enhances seed quality testing in several key ways:
Automated Seed Analysis
AI automates many aspects of seed testing, reducing the need for manual inspection. This significantly increases the speed and efficiency of the process, allowing for the analysis of larger seed samples in less time. Automated systems can handle repetitive tasks such as Seedling counting, sizing, and classifying seeds, freeing up human resources for more complex analyses. This automation also reduces the risk of human error, leading to more consistent and reliable results.
AI-Powered Imaging
AI-powered imaging systems utilize advanced computer vision algorithms to analyze seed images with high precision. These systems can detect subtle defects and variations in seed morphology that may not be visible to the naked eye.
This enhanced level of detail allows for more accurate identification of seed quality issues, leading to better selection and management practices. AI-powered imaging can also be used to assess seed viability and predict germination rates.
Predictive Analytics for Seed Quality
AI algorithms can analyze historical seed data, environmental factors, and other relevant information to predict future seed performance. This predictive capability allows seed producers and farmers to make informed decisions about seed selection, planting, and crop management. Predictive analytics can also be used to optimize seed storage conditions and minimize losses due to deterioration.
Benefits of AI in Seed Quality Evaluation
The adoption of AI for seed quality evaluation offers several significant benefits:
Increased Efficiency and Speed
AI automates many time-consuming tasks in seed testing, significantly increasing the speed and efficiency of the process. This includes automating tasks like counting, sizing, and initial quality assessments, which traditionally require manual labor.
This allows for rapid analysis of large seed samples, enabling faster turnaround times and more timely decision-making, ultimately accelerating the entire seed production and distribution cycle. This increased speed allows breeders and producers to respond more quickly to market demands and changing environmental conditions.
Higher Precision and Accuracy
AI-powered systems can analyze seed images and data with greater precision and accuracy than traditional methods, which often rely on subjective human observation. AI algorithms can detect subtle variations and anomalies that might be missed by the human eye, leading to more reliable and objective assessments of seed quality.
This reduces the risk of human error and improves the overall quality of seed stock, ensuring that farmers have access to the highest quality seeds for planting. This improved accuracy also contributes to better resource management by minimizing the planting of low-quality seeds.
Cost Reduction
By automating tasks and increasing efficiency, AI can reduce the overall cost of seed quality testing. This automation minimizes labor costs and reduces the need for expensive manual testing procedures. This can lead to significant cost savings for seed producers and farmers, making high-quality seed more accessible and affordable. These cost savings can be reinvested in research and development, further advancing the field of seed quality analysis.
Applications of AI in Seed Quality Testing
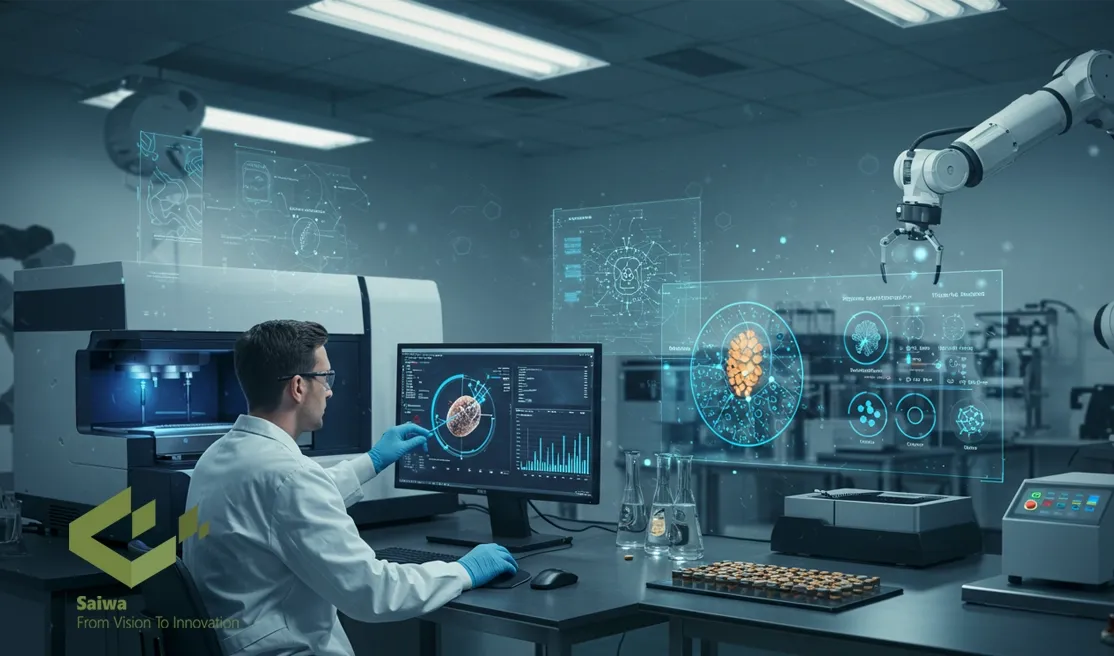
AI is being applied in various aspects of seed quality testing:
Seed Sorting and Grading
AI-powered systems can automatically sort and grade seeds based on size, shape, color, and other physical characteristics. This ensures that seeds meet specific quality standards and are suitable for planting, leading to more uniform crop stands and improved yields.
This automated sorting and grading process is significantly faster and more efficient than traditional manual methods, allowing for large-scale processing of seeds. This also ensures consistency in seed quality across different batches.
Detecting Seed Diseases and Defects
AI algorithms can be trained to identify specific seed diseases and defects, such as fungal infections or physical damage. This early detection allows for prompt intervention and prevents the spread of diseases, safeguarding crop health and yield.
AI-powered image analysis can detect subtle signs of disease or damage that might be missed by the human eye, enabling more accurate and timely diagnosis. This early detection is crucial for preventing widespread crop losses and ensuring food security.
Breeding Programs and Genetic Improvement
AI can be used to analyze genetic data and predict the performance of different seed varieties. This information is valuable for breeding programs, enabling the development of new seed varieties with improved traits, such as higher yield, disease resistance, and drought tolerance.
By analyzing vast amounts of genomic data, AI can identify desirable genes and predict the performance of different genetic combinations, accelerating the breeding process and leading to the development of superior seed stock. This data-driven approach to breeding allows for a more targeted and efficient selection of desirable traits.
AI and Predictive Seed Quality Analytics
AI is playing an increasingly important role in predictive seed quality analytics:
Data-Driven Seed Selection
AI algorithms can analyze vast amounts of data, including seed characteristics, environmental factors, and historical performance data, to guide seed selection. This data-driven approach allows farmers to choose the best seed varieties for their specific growing conditions, maximizing yield potential.
By considering factors such as soil type, climate, and pest prevalence, AI can recommend seed varieties that are best suited to the specific environment, increasing the likelihood of successful crop establishment and high yields. This personalized approach to seed selection can significantly improve the efficiency and profitability of farming operations.
Improved Forecasting of Crop Yields
AI can be used to predict crop yields based on seed quality, environmental conditions, and other relevant factors. This information is valuable for farmers, enabling them to make informed decisions about planting, fertilization, and other crop management practices.
By accurately forecasting crop yields, farmers can optimize resource allocation, minimize waste, and make informed decisions about marketing and sales. This predictive capability can significantly enhance the profitability and sustainability of agricultural operations.
Challenges in Implementing AI for Seed Quality
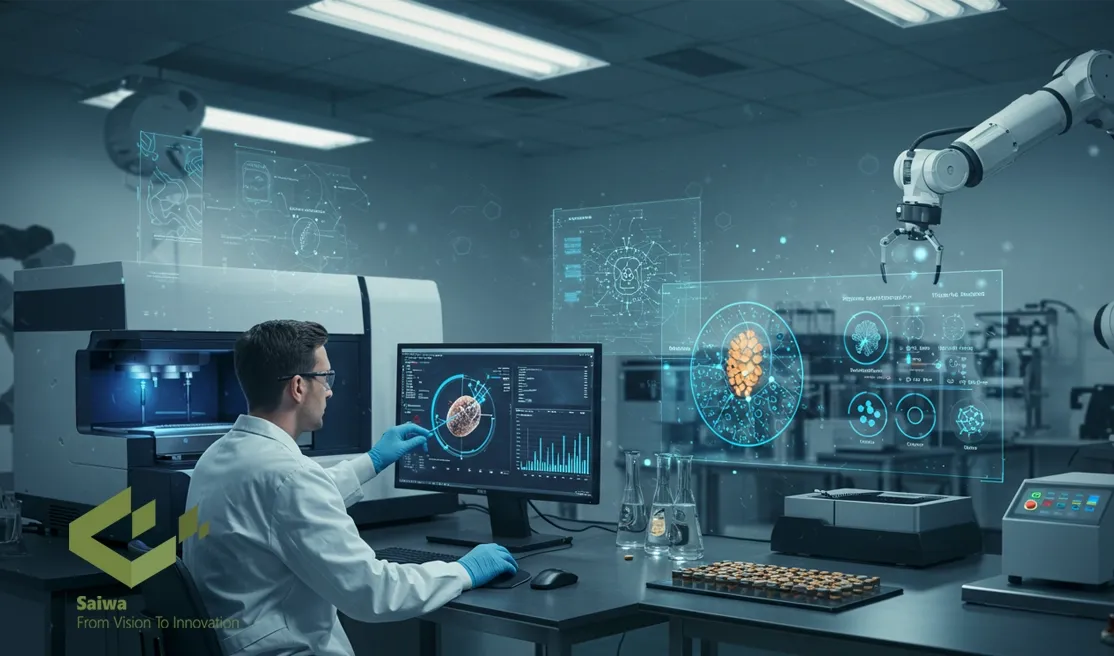
Despite the numerous benefits, there are challenges in implementing AI for seed quality:
Data Quality and Availability
AI algorithms require large, high-quality datasets for training and validation. These datasets must be representative of the diverse range of seed varieties, environmental conditions, and potential defects. The availability of such datasets can be a limiting factor, particularly for specific crop types or regions where data collection may be challenging or expensive.
Ensuring data quality and consistency is crucial for the accuracy and reliability of AI-powered systems, as biased or incomplete data can lead to inaccurate predictions and unreliable results. Building and maintaining these datasets requires ongoing investment in data infrastructure and collaboration among different stakeholders in the seed industry.
Cost and Accessibility for Small Farms
The cost of implementing AI-powered seed testing systems can be a barrier for small-scale farmers who may lack the financial resources to invest in new technologies. This cost includes not only the initial investment in hardware and software but also the ongoing costs of maintenance, training, and data management.
Making these technologies more accessible and affordable is essential for widespread adoption and equitable access to the benefits of AI in agriculture, ensuring that smallholder farmers are not left behind in the technological revolution. Developing affordable and user-friendly AI solutions tailored to the needs and resources of small farms is crucial for promoting inclusivity and maximizing the impact of AI in agriculture.
Integration with Traditional Seed Testing Methods
Integrating AI-powered systems with existing seed testing workflows and laboratory procedures can be complex. This requires adapting existing protocols, training personnel on new technologies, and ensuring seamless data exchange between different systems.
Developing seamless integration strategies and standardized protocols is crucial for maximizing the efficiency and effectiveness of AI in seed quality evaluation, minimizing disruption to existing workflows, and facilitating the smooth transition to AI-powered systems. This integration also requires addressing issues of data compatibility and interoperability between different platforms and systems.
The Future of AI in Seed Quality Evaluation
The future of AI in seed quality evaluation is promising:
Advancements in AI Technologies
Ongoing advancements in AI technologies, such as deep learning and computer vision, are continuously improving the accuracy, speed, and capabilities of AI-powered seed testing systems. These advancements are paving the way for more sophisticated and comprehensive seed quality analysis, enabling the detection of subtle defects, prediction of seed performance, and development of new seed varieties with improved traits.
These advancements are also leading to more robust and reliable AI models that can perform accurately under diverse environmental conditions and with limited data. The development of more powerful and efficient AI algorithms is driving innovation in seed testing and opening up new possibilities for improving seed quality.
Wider Adoption in the Seed Industry
As the benefits of AI become more apparent, its adoption in the seed industry is expected to increase. This wider adoption will drive further innovation and development, leading to more advanced and accessible AI-powered solutions for seed quality evaluation.
Increased adoption will also lead to the generation of more data, which can be used to further refine AI models and improve their accuracy. This positive feedback loop between adoption and innovation will accelerate the development and deployment of AI-powered solutions in the seed industry, benefiting all stakeholders along the value chain.
Conclusion
AI is transforming the field of seed quality evaluation, offering significant improvements in efficiency, accuracy, and cost-effectiveness. From automated seed analysis and AI-powered imaging to predictive analytics and disease detection, AI is revolutionizing various aspects of seed testing. While challenges remain in terms of data availability, cost, and integration, the future of AI in seed quality evaluation is bright.
Continued research and development, coupled with wider adoption in the seed industry, will unlock the full potential of AI in enhancing seed quality, contributing to sustainable agriculture, and ensuring global food security. Overcoming these challenges will require collaborative efforts from researchers, technology developers, and policymakers, to ensure that the benefits of AI are accessible to all stakeholders in the agricultural sector.