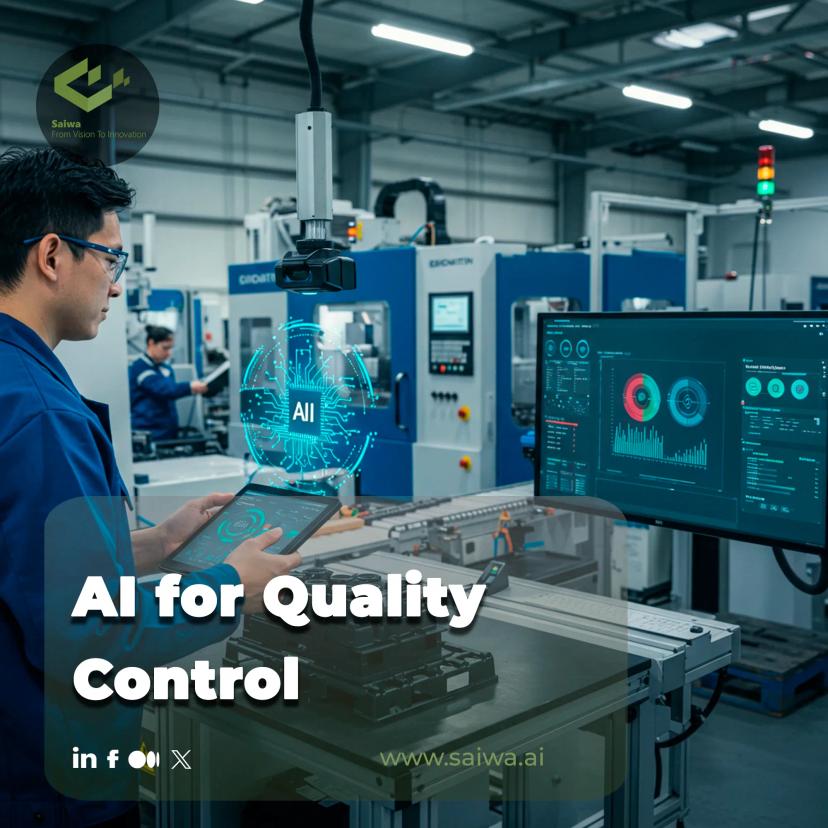
How to Use AI for Quality Control
Quality control, a critical aspect of any manufacturing process, has undergone significant transformation over the years. From manual inspections to automated systems, the quest for consistent product quality and operational efficiency has driven continuous innovation in quality control methods. Today, artificial intelligence (AI) is emerging as a game-changer, offering unprecedented opportunities to enhance quality control processes. It leverages machine learning algorithms and deep learning models to analyze vast amounts of data, identify intricate patterns, and automate complex inspection tasks.
Saiwa is an AI company that provides machine learning solutions through its platform, Fraime. Offering AI as a service, Saiwa's platform helps industries implement AI-powered tools such as anomaly detection, which is widely used in manufacturing for quality control. By identifying anomalies and deviations early in the production process, Saiwa enables manufacturers to proactively address potential defects, optimize workflows, and improve product quality without the need for extensive in-house AI expertise. This helps companies improve efficiency and reduce the costs associated with defective products or rework.
This article explores the evolution of quality control, the role of AI in this domain, its applications in manufacturing, the associated benefits and challenges, and the future trajectory of AI-driven quality control, ultimately aiming to provide a comprehensive understanding of how AI is revolutionizing quality management in the modern industrial landscape.
The Evolution of Quality Control
Quality control practices have evolved significantly over time, from rudimentary manual inspections to sophisticated automated systems. Early quality control methods relied heavily on human inspectors to visually inspect products for defects, a process that was often slow, error-prone, and limited in its ability to detect subtle defects.
Statistical process control (SPC) emerged as a more systematic approach, using statistical methods to monitor and control process variations, enabling a more data-driven approach to quality management. The advent of automation brought about automated inspection systems, which used sensors and cameras to detect defects, increasing speed and consistency.
Now, AI is poised to revolutionize quality control, enabling more accurate, efficient, and adaptive processes by incorporating advanced algorithms capable of learning from data, adapting to changing conditions, and making predictions about potential quality issues, going beyond the limitations of traditional rule-based systems. This evolution reflects the continuous drive to improve product quality, optimize processes, and reduce costs in the manufacturing industry.
The Role of AI in Quality Control
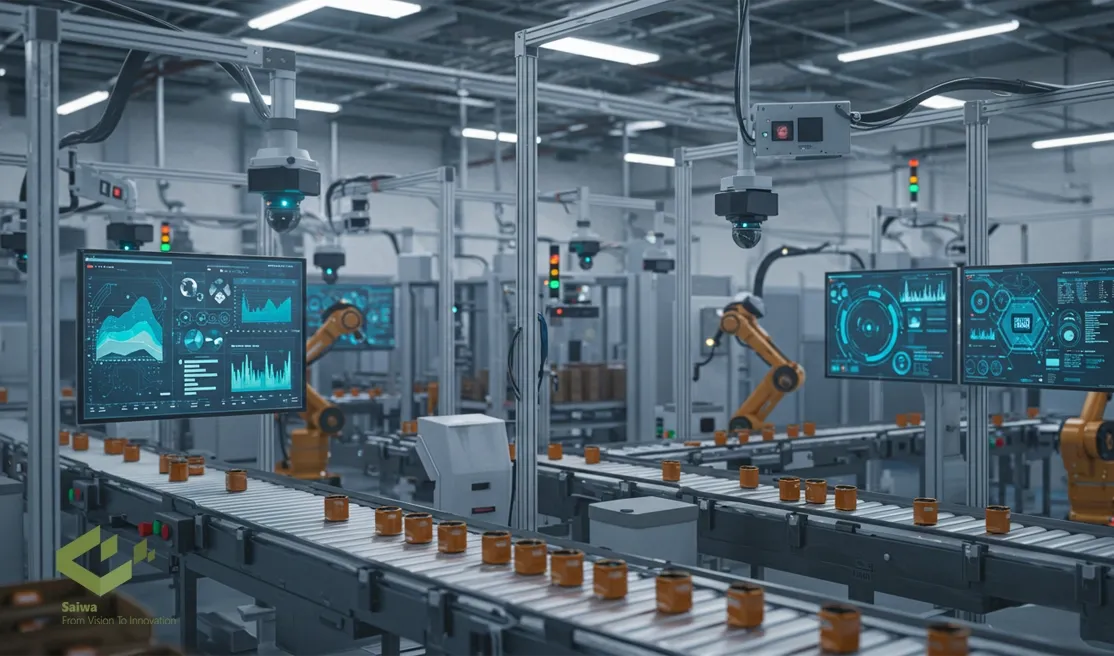
AI empowers quality control by automating complex tasks, analyzing vast amounts of data, and identifying subtle patterns that are difficult for humans to detect. AI algorithms can learn from data, adapt to changing conditions, and predict potential quality issues. This enables proactive quality management, preventing defects before they occur and minimizing waste.
Furthermore, AI can analyze data from various sources, including images, sensor readings, and production logs, to provide a holistic view of product quality and identify potential issues early in the production process.
This proactive approach minimizes the risk of defective products reaching the market and reduces the associated costs of rework, scrap, and warranty claims. AI also facilitates continuous improvement by identifying areas for process optimization and providing insights for enhanced decision-making, leading to more efficient and effective quality control processes.
By automating repetitive tasks, AI frees up human inspectors to focus on more complex and strategic activities, such as root cause analysis and process improvement initiatives, ultimately enhancing the overall quality management system.
Traditional Methods vs. AI in Quality Control
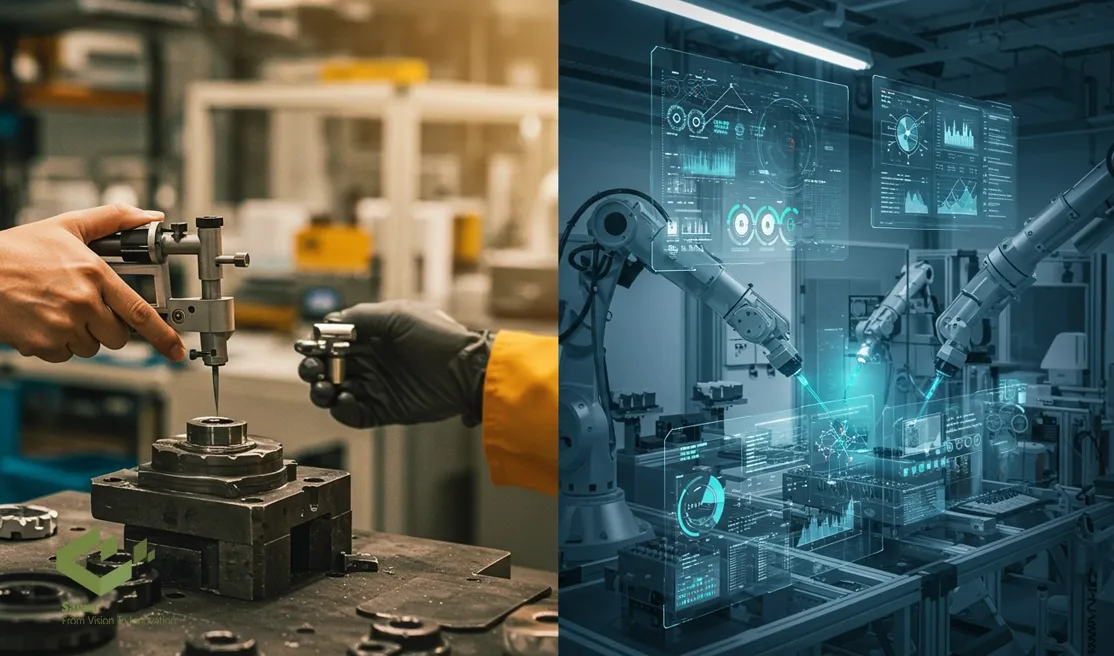
Traditional quality control methods, while effective to a point, have inherent limitations that AI can address. Manual inspections, which rely heavily on human visual acuity and judgment, are prone to human error, particularly in scenarios involving fatigue, complex defect identification, or high-volume production lines. In addition, manual inspections can be time-consuming and labor-intensive, leading to increased operating costs and potential bottlenecks in the production process.
Automated inspection systems, while more efficient than manual inspections in terms of speed and consistency, often lack the flexibility and adaptability of AI-powered systems, relying on pre-programmed rules and thresholds that may not capture the nuances of complex defects or adapt to variations in product characteristics.
AI, on the other hand, can analyze complex data sets, identify subtle patterns often imperceptible to human observation or traditional automated systems, and adapt to changing conditions, enabling more accurate and efficient quality control. This adaptability is particularly crucial in dynamic manufacturing environments where product specifications, materials, or processes may change over time.
How AI for Quality Control is Applied in Manufacturing: Key Use Cases
AI is being applied in various ways to enhance quality control in manufacturing.
Automated Visual Inspection
AI-powered computer vision systems can automate visual inspection tasks, detecting defects in products with high accuracy and speed. These systems leverage deep learning algorithms trained on vast image datasets to identify a wide range of defects, including scratches, dents, discoloration, and even microscopic cracks.
This eliminates the subjective nature of manual inspections, reducing labor costs and improving efficiency by enabling continuous, real-time inspection on production lines. Furthermore, these systems can be easily adapted to inspect different product types by retraining the AI models with new image data, offering a flexible and scalable solution for visual inspection.
Predictive Maintenance for Equipment Reliability
AI algorithms can predict equipment failures by analyzing sensor data and identifying patterns that indicate potential problems. This enables proactive maintenance, preventing costly downtime and ensuring equipment reliability.
By continuously monitoring sensor data such as temperature, vibration, and pressure, AI algorithms can detect anomalies and predict equipment failures before they occur. This allows maintenance teams to schedule repairs and replacements proactively, minimizing production disruptions and extending the lifespan of equipment.
Predictive maintenance can optimize maintenance schedules, reducing unnecessary maintenance activities that can be both costly and time-consuming, and ensuring that maintenance is performed only when needed.
Statistical Process Control & Optimization
AI can enhance Statistical Process Control (SPC) by analyzing process data and identifying optimal process parameters. This can improve product quality, reduce variability, and increase process efficiency. Traditional SPC methods rely on statistical analysis of sampled data, which may not capture the full complexity of the manufacturing process.
AI algorithms can analyze vast amounts of real-time data from various sources, including sensors, machines, and production logs, to identify subtle patterns and correlations that affect product quality. This enables more precise control and optimization of process parameters, leading to improved product consistency and reduced variability.
Accuracy Measurement & Quality Assurance
AI can automate accuracy measurements and quality assurance processes, ensuring that products meet specified tolerances and standards. This can improve product consistency and reduce the risk of defects. AI algorithms can analyze data from various sources, including sensors, cameras, and databases, to provide a comprehensive view of product quality.
This allows for real-time monitoring of product characteristics and identification of deviations from specified tolerances. By automating these measurements and analyses, AI can significantly reduce the time and effort required for quality assurance, while also improving the accuracy and reliability of the process.
This leads to greater product consistency and reduces the likelihood of defects reaching the customer, enhancing overall product quality and customer satisfaction.
The Impact of AI for Quality Control in Manufacturing Processes: Key Benefits
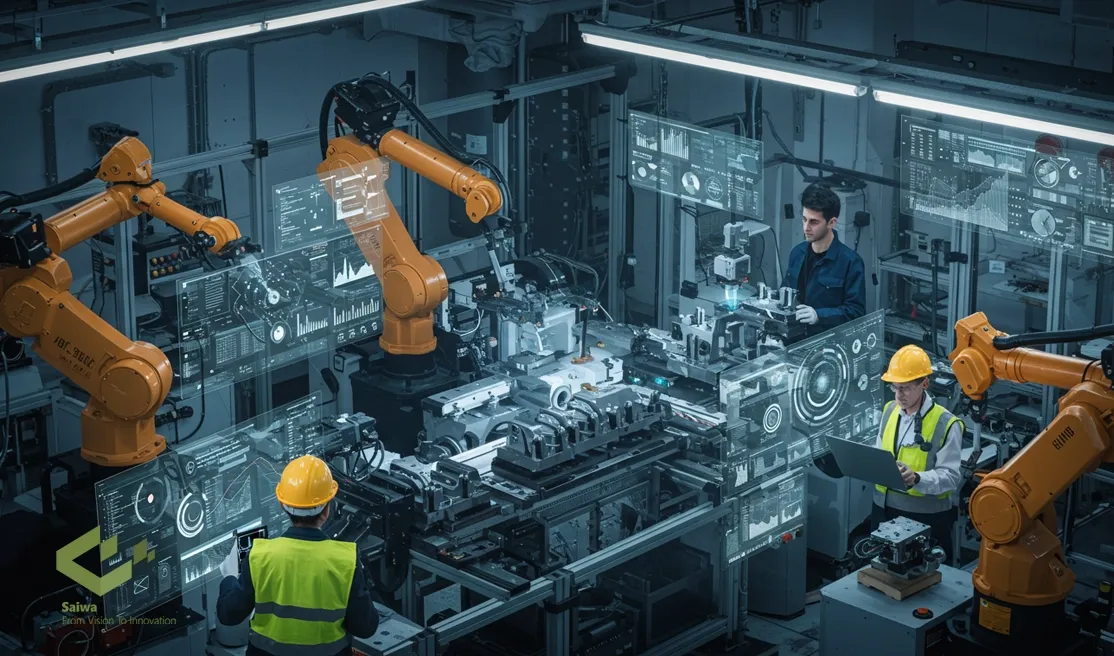
Implementing AI for quality control offers numerous benefits, significantly impacting manufacturing processes and overall business performance.
Enhanced Accuracy and Reliability
AI-powered systems can achieve significantly higher accuracy and reliability in defect detection and quality assessment compared to traditional methods, which are often subject to human error and limitations in detecting subtle flaws.
AI algorithms, particularly deep learning models trained on vast datasets, can discern intricate patterns and anomalies indicative of defects, leading to more precise and consistent quality evaluations. This reduces the risk of defective products reaching customers, protecting brand reputation, enhancing customer satisfaction, and minimizing the potential for costly product recalls or warranty claims.
Better Efficiency and Productivity
AI automates time-consuming quality control tasks, such as visual inspections and data analysis, freeing up human resources for more strategic activities that require human expertise and judgment, such as process improvement initiatives and root cause analysis.
This automation not only increases overall productivity by accelerating inspection and analysis processes but also reduces labor costs associated with manual quality control tasks. Furthermore, AI-powered systems can operate continuously, enabling 24/7 monitoring and inspection, further enhancing efficiency and output.
Continuous Learning and Improvement
AI algorithms, particularly machine learning models, can learn from data and adapt to changing conditions, enabling continuous improvement in quality control processes. As new data becomes available, the AI models can be retrained and refined, improving their accuracy and effectiveness over time.
This adaptability ensures that quality control systems remain effective and relevant even as product specifications, manufacturing processes, or environmental conditions evolve. This continuous learning loop fosters a culture of ongoing improvement and optimization within the quality control process.
Waste and Costs Reduction
By preventing defects early in the production process and optimizing process parameters, AI can significantly reduce waste and costs associated with rework, scrap, and warranty claims. Early surface defect detection allows for timely intervention and corrective action, minimizing the need for costly rework or scrapping of defective products.
Process optimization through AI-driven insights can lead to more efficient resource utilization and reduced material waste. These combined effects contribute to significant cost savings and improved profitability.
Enhanced Decision-Making Capabilities
AI provides valuable insights into product quality and process performance by analyzing complex datasets and identifying trends and correlations that may not be readily apparent through traditional methods.
This data-driven insight enables more informed decision-making across various aspects of the manufacturing process, from product design and development to production planning and resource allocation. These improved decision-making capabilities can lead to optimized product designs, more efficient processes, and enhanced overall business performance.
Challenges and Key Considerations: AI for Quality Control
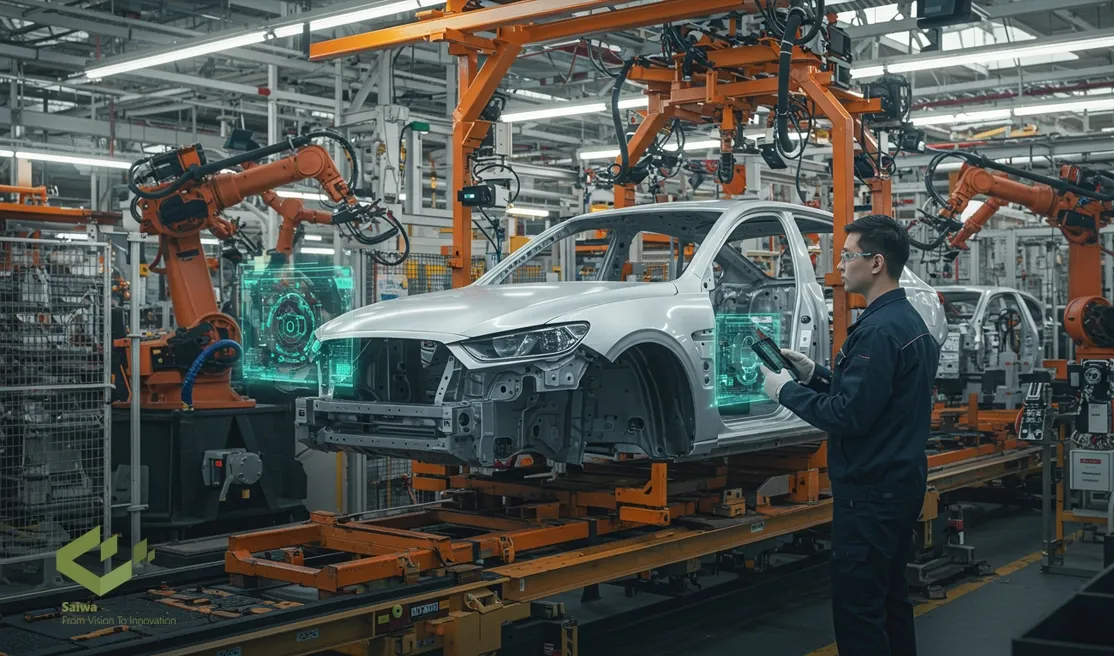
While AI offers significant advantages for quality control, there are also challenges and considerations to address before implementing AI-driven solutions.
Data Requirements
AI algorithms, especially deep learning models, require large amounts of high-quality data for training and effective performance. Acquiring, cleaning, labeling, and preparing this data can be a significant undertaking, requiring substantial time, resources, and expertise in data management. The quality of the data directly impacts the accuracy and reliability of the AI models, making data acquisition and preparation a critical aspect of AI implementation.
Integration with Existing Systems
Integrating AI-powered quality control systems with existing manufacturing systems, such as production line equipment, data acquisition systems, and enterprise resource planning (ERP) software, can be complex and require specialized expertise in systems integration and software engineering. Ensuring seamless data flow and interoperability between different systems is essential for the effective deployment of AI-driven quality control.
Cost of Implementation
Implementing AI-powered quality control systems can involve significant upfront costs, including hardware infrastructure for data processing and storage, software licenses for AI platforms and tools, and integration expenses associated with connecting AI systems to existing infrastructure. Organizations need to carefully evaluate the return on investment (ROI) and develop a comprehensive budget that accounts for all these costs.
Expertise and Skill Gaps
Developing, deploying, and maintaining AI solutions requires specialized skills and expertise in areas such as machine learning, data science, and software engineering. These skills may be lacking in some organizations, necessitating investment in training and development or the hiring of specialized personnel. Addressing these skill gaps is crucial for successful AI adoption and ensuring the long-term sustainability of AI-driven quality control systems.
Real-World Examples: AI for Quality Control
Several companies are already leveraging AI for quality control with impressive results.
BMW Group’s AI-based Inspection
BMW uses AI-powered computer vision systems to automate the inspection of painted car bodies, detecting defects such as scratches, dents, and inconsistencies in paint finish with high accuracy and speed.
This AI-driven system analyzes images captured by high-resolution cameras positioned along the production line, identifying even minute imperfections that might be missed by human inspectors. This automation significantly speeds up the inspection process, increases detection rates, and ensures consistent quality across all vehicles. By replacing manual inspections with this AI-powered system, BMW has improved efficiency, reduced inspection time, and enhanced the overall quality of its painted car bodies.
Intel’s AI-Powered In-Line Inspection
Intel utilizes AI for in-line inspection of semiconductor wafers, a critical process in chip manufacturing. AI algorithms analyze images of the wafers in real-time, identifying defects such as particles, scratches, and other anomalies early in the production process.
This early detection minimizes waste by preventing further processing of defective wafers and enabling timely corrective actions in the manufacturing process. By implementing AI-powered in-line inspection, Intel has improved yield rates, reduced material waste, and enhanced the overall efficiency of its semiconductor manufacturing operations.
The Future of AI in Quality Control
The future of AI in quality control is bright, with continued advancements in AI technologies and increasing adoption across industries. AI is expected to play an even greater role in automating quality control processes, optimizing production, and ensuring product quality. Emerging technologies such as edge AI, which brings AI processing closer to the data source, and explainable AI (XAI), which provides insights into the reasoning behind AI decisions, are expected to further enhance the capabilities of AI-driven quality control systems.
Edge AI will enable faster and more efficient real-time inspections, while XAI will increase trust and transparency in AI-driven quality control decisions. Furthermore, the integration of AI with other advanced technologies, such as Industrial Internet of Things (IIoT) and digital twin technology, will create even more sophisticated and comprehensive quality control systems, enabling predictive and prescriptive quality management capabilities.
Summing Up
AI is transforming quality control in manufacturing, offering unprecedented capabilities for enhancing accuracy, efficiency, and adaptability. By automating complex tasks, analyzing vast amounts of data, and adapting to changing conditions, AI empowers manufacturers to achieve higher levels of product quality, reduce costs, and enhance overall competitiveness.
While challenges remain in terms of data requirements, integration complexities, and the need for specialized expertise, the benefits of AI-driven quality control are undeniable. By addressing these challenges and embracing the potential of AI, businesses can unlock new opportunities for innovation and efficiency in their quality management processes, ultimately leading to improved product quality, enhanced customer satisfaction, and increased profitability in the increasingly competitive global market.