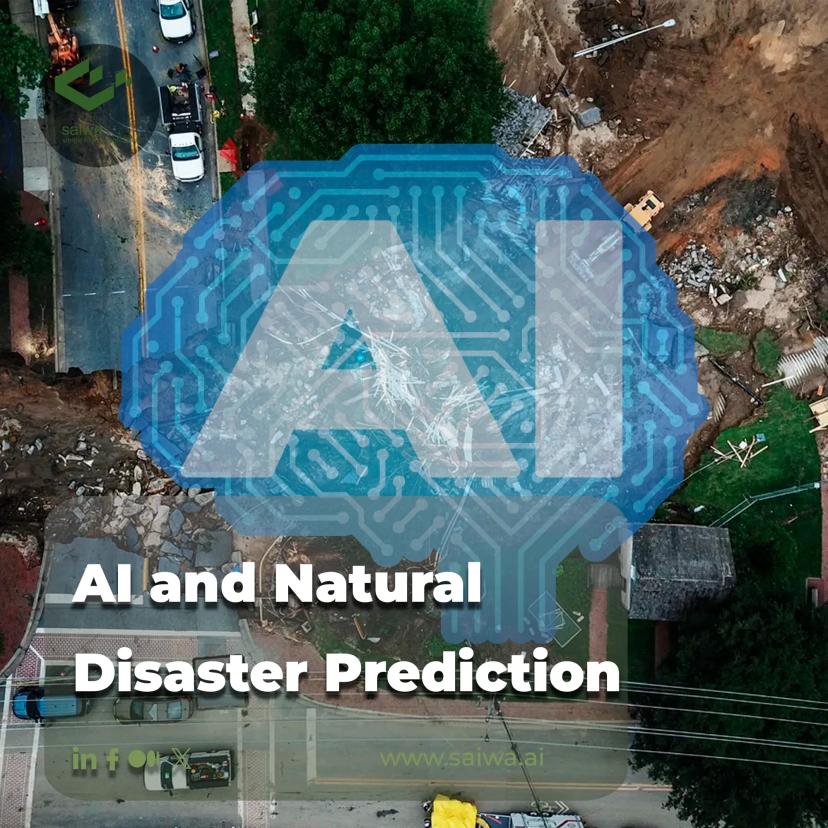
AI and Natural Disaster Prediction | All You Need to Know
Natural disasters such as hurricanes, wildfires, floods, and earthquakes cause massive destruction worldwide each year. Reliable early warning systems are crucial to mitigate risks and damage by informing evacuation planning and emergency response. However, inherent uncertainties and complex variables make predicting these chaotic events exceptionally challenging.
Recent advances in artificial intelligence as a service (AIaaS) and machine learning offer new opportunities to strengthen forecasting and risk quantification of natural disasters. From honing hurricane path projections to flagging wildfires from satellite data, AI-enhanced models promise to save lives and resources through enhanced preparedness.
This blog post provides an overview of core AI and Natural Disaster Prediction techniques applied to various natural disaster prediction efforts and examples of current models and systems. We discuss AI's significant advantages, current limitations, and barriers to operational deployment. AI has vast potential to revolutionize environmental prediction and boost resilience – but only if intelligently integrated with domain expertise and local realities.
Importance for Disaster Management
Reliable early warning systems enable disaster management agencies to initiate protective protocols minimizing risks. Warnings on cyclones, floods or storms allow securing of key infrastructure, readying medical supplies, positioning emergency personnel and guiding threatened populations towards safe zones. Overall, natural disaster prediction using machine learning fills a vital gap within comprehensive prediction and preparedness capabilities.
Using AI to Predict Natural Disasters
Advanced warning of impending natural disasters can save countless lives by enabling evacuations, preparations, and mobilization of emergency responders. However, accurate and timely disaster prediction represents an immensely complex challenge. Artificial intelligence (AI) techniques offer new opportunities to improve forecasting accuracy across various hazardous events. In this paper, we provide an overview of AI approaches for disaster prediction, examples of current systems, advantages and challenges, and an outlook on the future potential of AI in this domain.
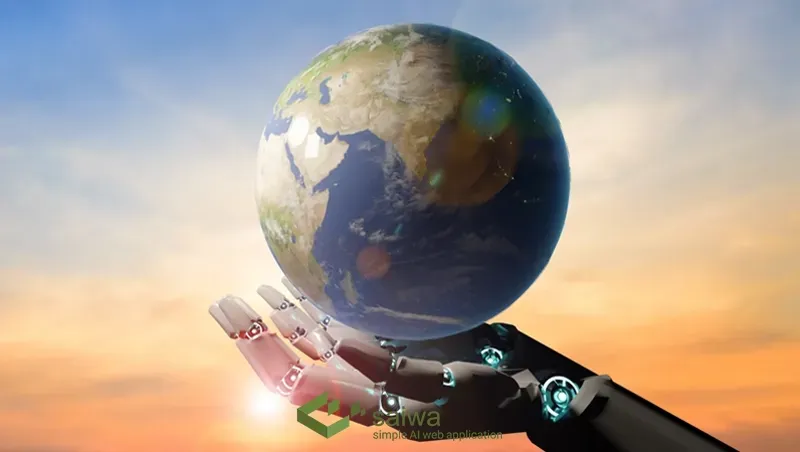
How Does It Work?
AI leverages advanced machine learning algorithms to detect subtle patterns in massive multivariate datasets relevant to disaster formation. By "training" on large labeled datasets, systems can learn to model extremely complex phenomena. Statistical methods then allow assigning probabilistic forecasts to convey uncertainty.
Common methods include neural networks to identify nonlinear relationships, computer vision for analyzing earth observation imagery, natural language processing to extract insights from disaster reports, and reinforcement learning to optimize predictive models.
For example, a convolutional neural network could be trained on satellite images of conditions preceding historical wildfires alongside meteorological data. This allows the model to learn combinations of vegetation moisture, terrain, temperature, winds, etc., that tend to lead to ignition so it can recognize similar patterns in new data. AI takes advantage of exponential growth in environmental monitoring data volume and variety.
Integrating Traditional Knowledge and Local Observations
While AI and natural disaster prediction using machine learning techniques offer powerful tools for natural disaster prediction, it is essential to recognize the value of traditional knowledge and local observations. Indigenous communities and local populations have accumulated invaluable experience and knowledge about their environments, often spanning generations. Integrating this knowledge with AI-based models can enhance their accuracy, relevance, and acceptance within affected communities.
Traditional ecological knowledge (TEK) encompasses the cumulative body of knowledge, practices, and beliefs developed by indigenous and local communities through their long-term interactions with the natural environment. This knowledge can provide insights into environmental patterns, early warning signs, and effective coping strategies that may not be captured in conventional scientific data or models.
Read Also: AI in Ecology | Expanding Ecology’s Vision with AI
AI Methods for Disaster Forecasting
AI is well suited for natural disaster modeling because of its ability to analyze vast amounts of multi-dimensional data and detect subtle patterns. Several machine learning techniques are powering new forecasting systems:
Neural networks identify nonlinear relationships in historical disaster data that improve model accuracy.
Computer vision analyzes high-resolution aerial or satellite imagery using object recognition and change detection algorithms. This enables real-time disaster monitoring, like flagging wildfire ignition before visible smoke.
Natural language processing extracts useful insights from disaster-related documents and reports to augment other data. Analyzing post-wildfire assessment reports could uncover patterns linking fire damage to terrain, vegetation, and climate variables.
Simulation ensembles combining AI-enhanced fluid dynamics, atmospheric models, and hydrological simulations with uncertainty quantification provide highly localized forecasts. AI can help optimize model inputs and parameters.
Meteorology and Storm Forecasting
Modern natural disaster prediction using machine learning relies heavily on machine learning supplemented by physical atmospheric modeling. Neural networks analyze vast datasets spanning surface and satellite observations, buoy readings and computational fluid models to forecast precipitation, tropical cyclones, storm surges etc. Accurate meteorological simulations require processing numerous weather variables like temperature, humidity, and wind pressures across multiple altitudes globally.
Machine learning has significantly improved scope and lead times of storm warnings as algorithms assimilate indicators unnoticed by humans. Models also generate probabilistic forecasts conveying uncertainty ranges crucial for optimal decision-making. Such enhanced early weather warnings curb substantial losses to property, agriculture, and infrastructure across regions.
AI-driven Decision Support Systems for Disaster Response
While accurate natural disaster prediction using machine learning is crucial, the effective translation of these predictions into timely and coordinated response actions is equally important. AI-driven decision support systems (DSS) can play a vital role in bridging this gap, providing actionable insights and recommendations to disaster management agencies and emergency responders.
An AI-driven DSS for disaster response typically integrates multiple components, including:
Predictive models: Incorporating AI-based natural disaster prediction models, such as those discussed in the article, to generate forecasts and risk assessments.
Resource allocation optimization: AI techniques like constraint programming, mixed-integer programming, and multi-objective optimization can be used to optimize the allocation of limited resources (e.g., emergency personnel, equipment, evacuation routes) based on predicted disaster impacts and priorities.
Logistical planning: AI can assist in planning and coordinating logistics for disaster response, such as routing emergency vehicles, scheduling relief supply deliveries, and identifying optimal staging areas.
By integrating these components, AI-driven DSS can provide comprehensive decision support, from early warning and risk assessment to resource allocation, logistics planning, and impact mitigation. These systems can enhance the efficiency, coordination, and effectiveness of disaster response efforts, ultimately contributing to the reduction of loss of life and property damage.
What Natural Disasters Can AI Predict?
In the AI and Natural Disaster Prediction field, AI models are being developed and tested for a range of natural hazards:
Hurricanes
Hurricane track and intensity forecasting has improved through neural network analysis of meteorological data, sea surface temperatures, upper atmospheric wind patterns, and CNN-processed satellite imagery. Systems like ClimaCell's Hurricane Guidance provide frequent hurricane forecast updates and probability cones much faster than conventional models.
Wildfires
Computer vision algorithms can continuously monitor overhead imagery to identify emerging wildfire hotspots earlier than human analysts alone. Automated satellite analysis by companies like Space Know detects smoke plumes and burn scars to map fire spread in real time. Early warning allows for mobilizing suppression resources before fires grow out of control.
Floods
AI can ingest river gauge and precipitation data alongside HYCOM hydrological models to predict flood timing, severity, and damage. One Concern combines CNNs with hydraulic simulations to estimate potential flood depths at the individual building level across cities. Such precision fosters proactive mitigation.
Volcanic Eruptions
Sensors provide rich datasets on pre-eruption indicators like seismicity, ground deformation, and gas emissions. Machine learning analysis may identify precursor patterns to provide early alerts. However, further research is needed as eruptions remain difficult to forecast.
Earthquakes
True short-term earthquake prediction is not possible due to random natural fault dynamics. However, AI shows promise for generating real-time shaking alerts using distributed seismic and GPS networks for rapid-onset events. This enables automatic safety system activation.
Volcanic eruptions prediction harnesses satellite measurements tracking rise of magma, accumulation of subsurface molten rock and associated tremors and gas emissions. Thermal anomalies, sideways ground inflammation and abnormalities in the harmonic tremor also signal trouble. Simulation of ash clouds and lava flows based on these variables provides early warnings to communities downstream formulated by algorithms.
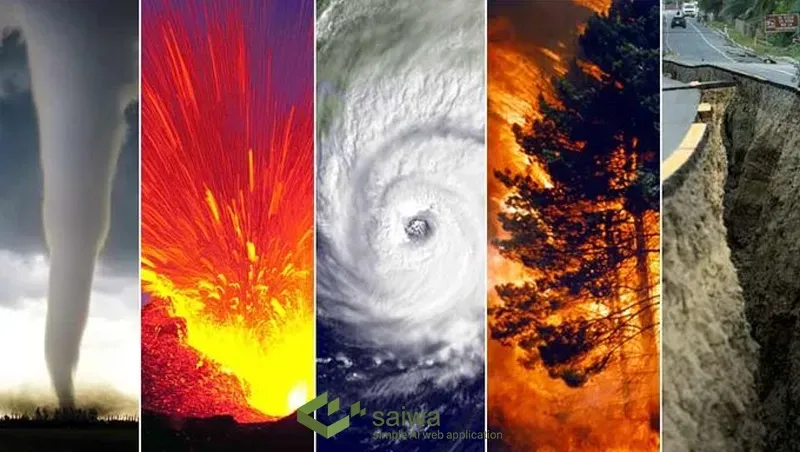
Fires
Because of the search for flames, firefighters in mountainous terrain may not know until they reach the fire that it is difficult to climb. Some places are impenetrable for fire engines and it takes a lot of time to walk to those places, and the use of helicopters or airplanes is necessary in this situation. The use of technology helps them identify the flames faster and more accurately.
Artificial intelligence systems use sensors that are strategically placed in target areas to detect chemical traces, smoke particles and gases in the air and receive temperature data. The data is analyzed by a cloud-based system that updates digital maps and sends alerts and coordinates to responders' cellphones.
Algorithms are designed to examine sensor signals with the aim of distinguishing between smoke from non-hazardous sources such as residential chimneys or fires and smoke from hazardous fires. Turning on multiple sensors can be very helpful because the intensity of multiple sensors is more likely to indicate that the fire is spreading.
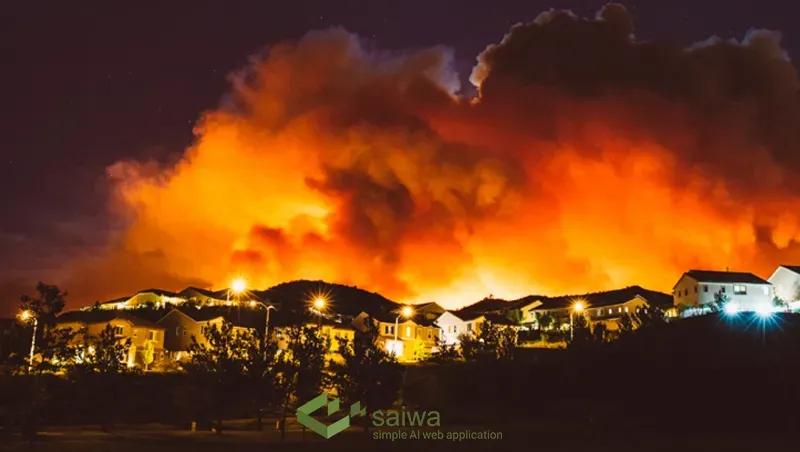
Potential of IoT Sensors and Drones
In natural disaster prediction using machine learning field, proliferation of wireless IoT sensors allows establishing vast environmental monitoring networks detecting minute seismic shifts, soil moisture changes or temperature anomalies instantly transmitting data to cloud analytics. Combining such granular ground-truth feeds with aerial scans from drones and satellites provides invaluable surrogate indicators for disaster prediction systems. Drones also enable rapid visual confirmation to validate warnings for targeted alerts.
Advantages of AI and Natural Disaster Prediction
Compared to traditional physical modeling, AI-enhanced prediction offers multiple advantages:
Analyze vast data volumes unmanageable for manual techniques
Rapidly update models by retraining on new disaster event data
Customize forecasts to local geographies using high-resolution inputs
Continually enhance accuracy over time via feedback loops
Leverage expanding data streams from satellites, IoT sensors, and simulations
Provide probabilistic risk quantification to support decision-making under uncertainty
AI empowers responding to accelerating climate changes and events outside historical norms that challenge purely physics-based approaches. AI's flexible data integration and pattern recognition capabilities are ideal for disasters' complex, chaotic nature.
Challenges of AI in Natural Disaster Prediction
However, besides AI and Natural Disaster Prediction benefits, significant barriers remain to operational AI deployment:
Addressing the black-box nature of neural networks for decision-makers to trust predictions
Quantifying uncertainties and communicating forecast probabilities effectively
Acquiring sufficient labeled training data, especially on rare or unprecedented events
Generalizing models to new locations that differ from original training distributions
Seamlessly integrating AI components into existing forecasting systems and workflows
Avoiding overconfidence in AI outputs, requiring ongoing human supervision and interpretation
The ultimate goal is to combine scientific, experiential, and AI approaches into optimal hybrid forecasting architectures. AI's flexibility offers little value in isolation. Thoughtful human-machine collaboration is needed to transform early warning capabilities while addressing ethical risks.
Importance of Interpretable Models for Natural Disaster Prediction Using Machine Learning
While machine learning achieves strong predictive performance, model transparency is also crucial for disaster planning applications. Complex neural networks behave as black boxes lacking in interpretability. Using methods like SHAP that explain model outputs is vital for trust and actionability. If meteorologists don't understand what trends the model responds to, they cannot effectively incorporate its outputs into their existing workflows. Visualization techniques that highlight influential regions like weather fronts also facilitate expert evaluation. Only if domain practitioners have visibility into model reasoning can AI meaningfully augment human intelligence for disaster preparation.
The Future of AI Prediction in Natural Disaster Prediction
Future satellite coverage and computing power improvements will continue expanding AI's forecasting capabilities. Over the next decade, experts predict:
Explosion in high-resolution earth observation data from public and private sector satellites
Enhanced simulation systems integrating real-time sensor networks like Doppler radar
More meteorological agencies trusting and adopting neural network forecasts
Leveraging crowdsourced photos, social media, and Internet of Things sensor data
Smart city integration for localized real-time disaster anticipation
More investment in disaster-related AI by technology firms and startups
However, even with advanced AI, fundamental limits to prediction will remain. Forecasting extreme events outside historical precedents may not be possible. Communication, interpretability, and ethics will be ongoing challenges. Ultimately, AI should augment, not replace, human expertise.
Ethical Considerations Around Warnings
Employing natural disaster prediction using machine learning raises important ethical questions around warning distribution:
Models pre-trained primarily on data from developed nations may inadequately represent risks in underserved regions. Careful testing on diverse geographical areas is imperative.
Similarly, blindly applying models risks over- or under-estimating threats to disadvantaged communities lacking resources to act on alarms. Equity should be prioritized.
Over-warning of false alarms also carries risks like evacuation costs and loss of public trust. Caution is warranted in issuing alerts, especially with lower confidence predictions.
Being transparent about algorithmic limitations and uncertainties enables the public to make informed decisions aligned with their risk tolerance.
Overall, ethical AI applied to disaster prediction should aim to empower the most vulnerable through thoughtful and inclusive development, testing, and communication of predictive capabilities for all.
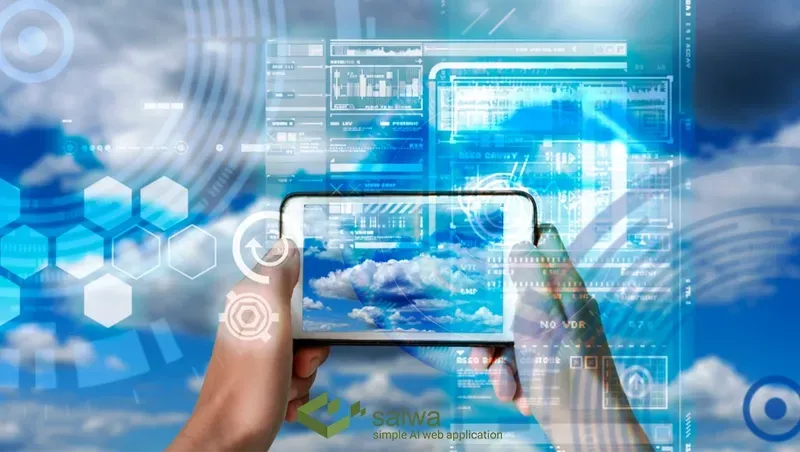
Natural disaster prediction using machine learning
Natural disasters are a constant threat to society and natural habitats and are highly unpredictable. These events affect over 100 million people worldwide annually and can disproportionately affect certain regions and populations, widening the capacity gap between societies.
In the past years and in the face of various natural disasters, the interest in Natural disaster prediction using machine learning has increased. In many fields, including medicine, finance and other cases, machine learning has attracted the attention of many people due to shrinking processing timelines, increasing computing power and increasing the usability of large data sets. In the Natural disaster prediction using machine learning, there are many benefits for society and nature because it can provide the possibility of investing on a large amount of available spatial data, improving the effectiveness of emergency communication and increasing the accuracy of prediction.
Role of AI in Post-Disaster Recovery
The role of AI in post-disaster recovery is becoming increasingly important, complementing its capabilities in natural disaster prediction using machine learning. After a disaster occurs, AI can swiftly assess damage, optimize resource allocation, and guide reconstruction efforts. By analyzing data from satellite imagery, social media, and IoT sensors, AI systems can identify the most affected areas, ensuring that relief and recovery efforts are directed where they are needed most.
AI-driven models, rooted in natural disaster prediction using machine learning, can assess structural damage and predict secondary hazards, such as landslides or flooding. This real-time analysis supports the efficient deployment of emergency services, enabling faster and more effective responses.
In addition, AI can streamline the logistics of disaster recovery by optimizing supply chains, routing emergency vehicles, and coordinating volunteer efforts. These AI capabilities, based on natural disaster prediction using machine learning, enhance the speed and efficiency of recovery operations.
AI also contributes to long-term rebuilding efforts by providing insights into resilient infrastructure design. By learning from past disasters, AI systems can recommend construction practices and materials that are more likely to withstand future events, ensuring communities are better prepared.
Overall, the integration of AI in post-disaster recovery, alongside natural disaster prediction using machine learning, plays a critical role in building more resilient communities and improving recovery outcomes.
Explainable AI Models for Disaster Prediction
Explainable AI (XAI) models are revolutionizing methods to predict natural disasters by offering transparency and improved accuracy. Unlike traditional machine learning models, which often function as black boxes, explainable models provide insights into how predictions are made. This added transparency is essential, especially for disaster prediction, where understanding the rationale behind forecasts can help communities and emergency responders make better, informed decisions.
Enhanced Model Transparency
By visualizing and interpreting model outputs, XAI allows disaster management teams to see exactly how and why certain factors, like seismic activity or weather patterns, influence predictions. This transparency strengthens methods to predict natural disasters by building confidence in the forecast results. Additionally, it helps stakeholders understand the specific risk factors for each prediction, enabling them to make data-backed decisions.
Improved Accuracy and Response
Explainable models improve accuracy by considering complex interactions between environmental data points, which enhances methods to predict natural disasters with more precise, location-specific forecasts. With these insights, teams can prepare specific response strategies based on the likely severity of an event, minimizing both potential damage and loss of life.
Real-time Adaptability
With XAI models, disaster predictions can be adjusted in real-time based on new data. This adaptability makes methods to predict natural disasters more responsive, enabling rapid updates as environmental conditions shift. The ability to update predictions as situations change is vital for fast, accurate decision-making during disaster events.
Conclusion
We reviewed some AI and Natural Disaster Prediction techniques in this blog post. AI significantly promises to strengthen early warning capabilities for natural disasters, potentially saving lives and property. However, thoughtfully addressing current limitations and integrating social factors will be critical to translating promising technology into operational reality. AI could transform environmental prediction and boost community resilience globally if developed responsibly through academic-industry-government collaboration.