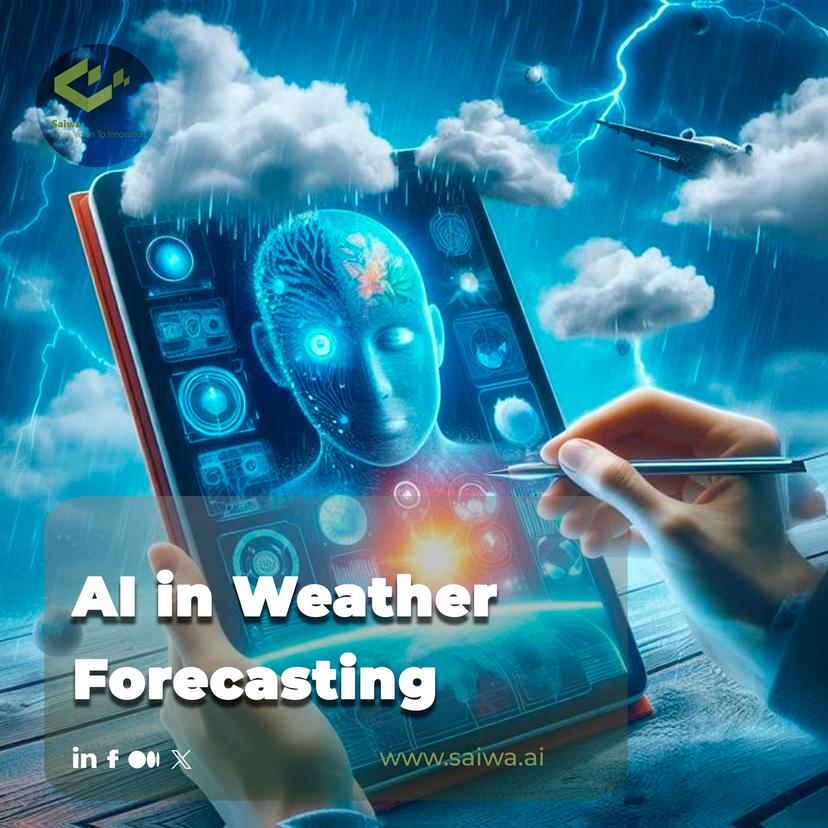
AI in Weather Forecasting | The Future is Clearer Than Ever
Weather forecasting, the practice of predicting the atmospheric state at a future time and specific location, has been a human pursuit for centuries. From ancient civilizations interpreting natural signs like cloud formations and animal behavior, to modern meteorological models crunching vast amounts of data, the quest for accurate predictions has constantly evolved. Initially, forecasting relied heavily on subjective observations and rudimentary instruments. The advent of computers and numerical weather prediction (NWP) marked a significant leap forward. Today, Artificial Intelligence (AI) is poised to revolutionize this field further, offering unprecedented accuracy, speed, and insights.
This article explores AI's transformative role in weather forecasting, examining its methodologies, applications, benefits, challenges, and future potential.
The Importance of Accurate Weather Forecasting
Accurate weather forecasting is not just about knowing whether to carry an umbrella. It's crucial for various sectors, impacting daily life, long-term planning, and even economic stability. Agriculture, a weather-dependent sector, relies on forecasts for optimal planting and harvesting schedules, irrigation management, and pest control.
Transportation, including aviation, shipping, and road travel, depends on accurate predictions to ensure safety, optimize routes, and minimize delays. Disaster management utilizes forecasts to prepare for and mitigate the impact of severe weather events like hurricanes, floods, and wildfires, enabling timely evacuations and resource allocation. Accurate predictions also inform energy consumption patterns, helping balance supply and demand, and optimize renewable energy generation.
Furthermore, weather forecasts are essential for public safety, enabling timely warnings and preparations for hazardous conditions, and protecting lives and property. Accurate forecasts can also benefit tourism, construction, and retail industries by informing operational decisions and minimizing disruptions.
Traditional Weather Forecasting Methods
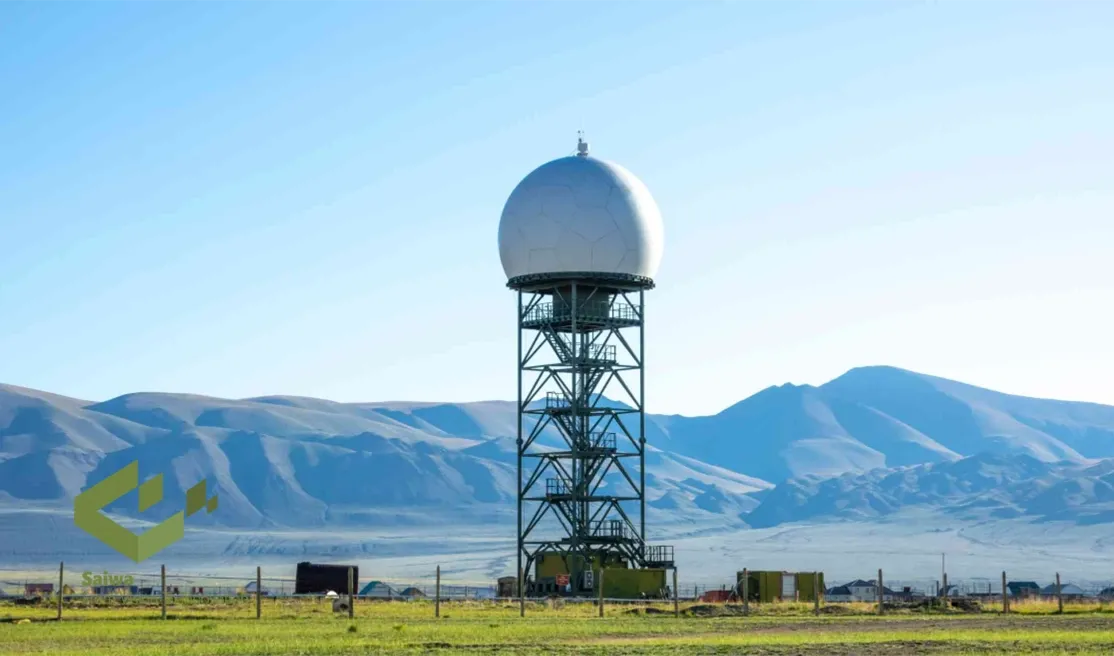
Traditional weather forecasting primarily relies on Numerical Weather Prediction (NWP). NWP uses complex mathematical models representing the physical processes of the atmosphere, including fluid dynamics, thermodynamics, and radiative transfer. These models are initialized with current weather observations from various sources like weather stations, balloons, aircraft, and satellites.
The models then solve complex equations to project the state of the atmosphere forward in time, generating forecasts. Data assimilation techniques are employed to incorporate new observations into the model as they become available, correcting errors and improving forecast accuracy. However, NWP models are computationally expensive, requiring powerful supercomputers.
They can also be limited by the resolution of the model grid, which determines the level of detail captured, and the accuracy of the initial conditions, which can be affected by observational errors and gaps. Furthermore, representing complex atmospheric phenomena like clouds and precipitation remains a challenge for NWP models.
How AI is Transforming Weather Forecasting
AI is transforming weather forecasting by augmenting and improving traditional methods in several key ways. AI algorithms can analyze massive datasets of weather observations, satellite imagery, radar data, social media feeds, and other relevant information to identify patterns and relationships that may not be apparent to human forecasters or captured by traditional models.
AI can also improve the efficiency of NWP models by optimizing data assimilation techniques, reducing computational costs, and improving the representation of subgrid-scale processes. In addition, AI can generate probabilistic forecasts that provide a range of possible outcomes and their associated probabilities, which is more valuable for decision-making under uncertainty than deterministic forecasts that provide a single prediction. AI can also learn from past predictions and their errors, continuously improving its predictive capabilities.
Advanced Techniques in AI Weather Forecasting
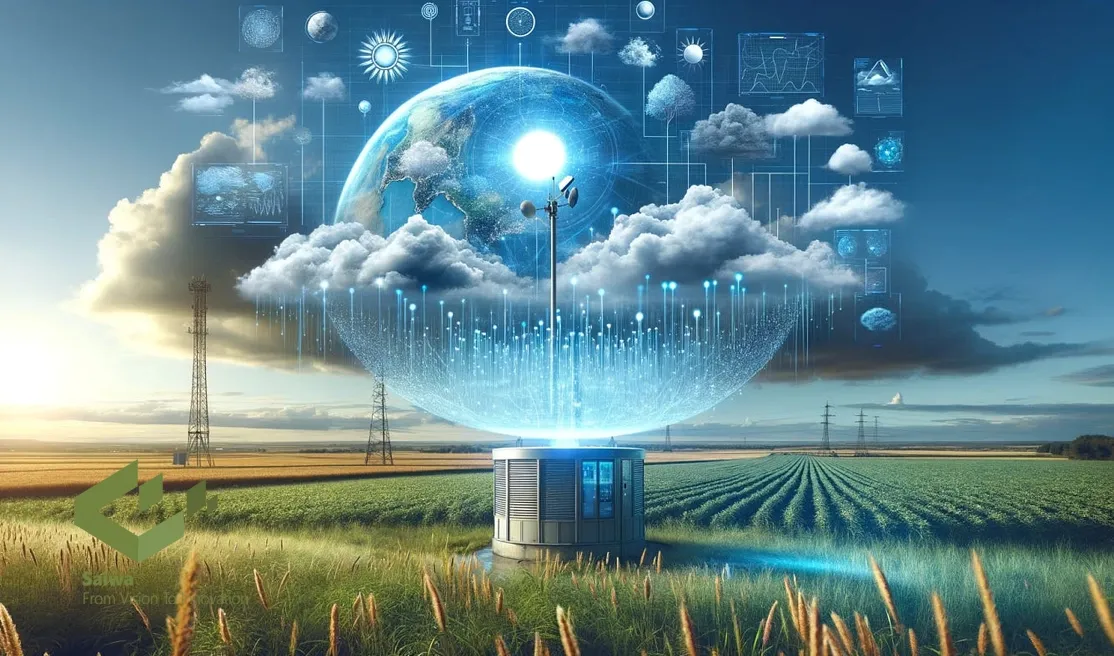
Several advanced AI techniques are being employed in weather forecasting:
Machine Learning Algorithms
Machine learning algorithms, a subset of AI, enable computers to learn from data without the necessity of explicit programming. Supervised learning algorithms, including artificial neural networks (ANNs) that emulate the structure of the human brain and support vector machines (SVMs) that identify optimal hyperplanes for data separation, can be trained on historical weather data to predict future weather conditions.
To illustrate, an artificial neural network (ANN) can be trained to predict temperature based on historical data sets comprising temperature, humidity, and wind measurements. Unsupervised learning algorithms, such as clustering algorithms, which group similar data points, can identify hidden patterns and structures in weather data, thereby facilitating a more profound comprehension of atmospheric processes and the discovery of new predictors.
Reinforcement learning algorithms, which learn through trial and error by interacting with an environment, can optimize forecasting strategies by learning from feedback and adapting to changing conditions. For example, a reinforcement learning agent can learn to optimize the parameters of a nowcasting model to maximize its accuracy.
Data Assimilation
Data assimilation can be defined as the process of integrating observations into numerical models to improve their initial conditions. Artificial intelligence (AI) can facilitate data assimilation by developing more sophisticated algorithms that can accommodate a range of data sources, including unconventional sources such as crowdsourced data from smartphones, and account for uncertainties in observations.
For example, AI can discern the distinctive error profiles of disparate observation types and assign appropriate weights during the assimilation process. This results in the generation of more accurate initial conditions for NWP models, which in turn yields enhanced forecast outcomes.
Ensemble Forecasting
Ensemble forecasting entails the execution of multiple NWP models with slight discrepancies in their initial conditions or model parameters. The underlying premise is that the dispersion of the ensemble members is indicative of the inherent uncertainty associated with the forecast. Artificial intelligence (AI) can be employed to integrate the output of these ensemble members into a unified, more precise, and probabilistic forecast. In contrast to the straightforward averaging of ensemble members, artificial intelligence can be employed to identify optimal weighting schemes based on historical performance metrics. Additionally, artificial intelligence can be employed to generate probabilistic forecasts by analyzing the distribution of the ensemble members and estimating the probability of different weather outcomes.
Explainable AI (XAI)
The objective of explainable AI (XAI) is to render AI-driven forecasts more transparent and comprehensible. It is often observed that traditional AI models, particularly those based on deep learning, are perceived as "black boxes" due to the difficulty in comprehending the underlying mechanisms that lead to their predictions.
The application of XAI techniques can facilitate the identification of the factors that contribute to a specific forecast, thereby enhancing the trust placed in such forecasts by both forecasters and users. Furthermore, the use of XAI enables forecasters to more effectively interpret and communicate the predictions that they have made. For instance, XAI can ascertain which input variables (e.g., temperature, humidity, wind speed) exert the greatest influence on a specific forecast.
Applications of AI in Weather forecasting
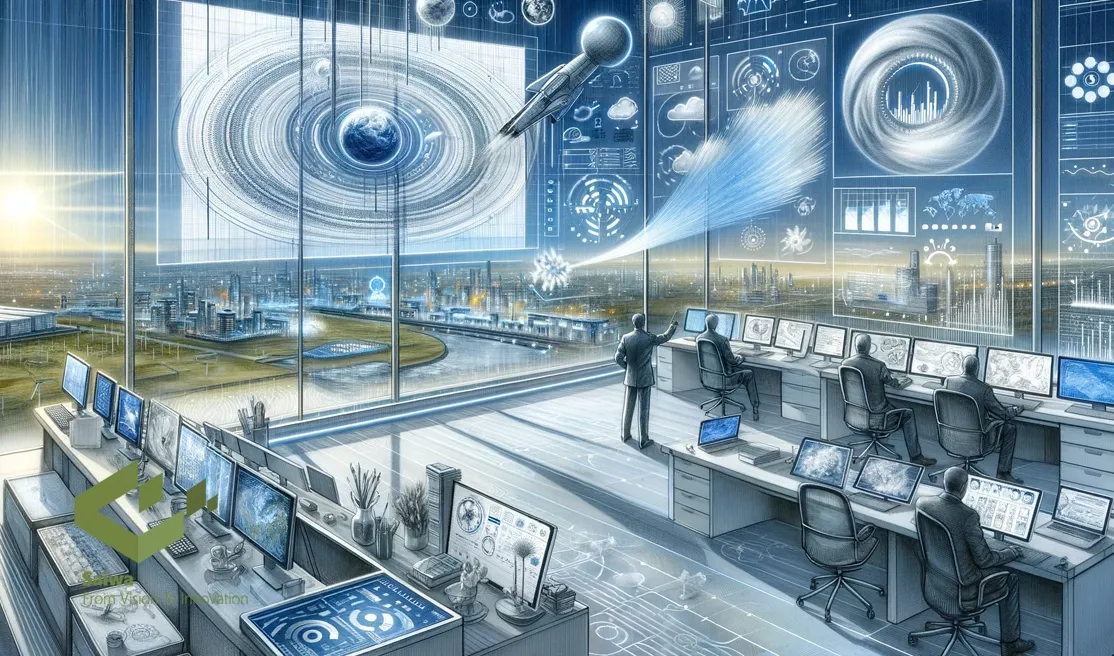
AI is being applied to various aspects of weather forecasting:
Nowcasting and Short-Term Forecasting
AI excels at nowcasting, and predicting weather conditions in the very short term (0-6 hours). AI algorithms can analyze real-time data from radar, satellites, and surface stations to predict the evolution of precipitation, thunderstorms, fog, and other rapidly developing weather phenomena. This is particularly useful for predicting flash floods, severe thunderstorms, and other hazardous weather events that require immediate action.
Medium-Range Weather Forecasts and Long-Range Weather Forecasts
AI is also being used to improve medium-range (2-10 days) and long-range (more than 10 days) weather forecasts. By learning from historical data and identifying long-term patterns, AI can improve the accuracy of these forecasts, particularly for predicting temperature and precipitation trends.
For example, AI can improve the prediction of heat waves, cold snaps, and extended droughts. Long-term forecasting remains a challenge, but AI offers the potential to improve seasonal and sub-seasonal forecasts, which are critical for agricultural planning and water resource management.
Extreme Weather Event Prediction
The ability to predict extreme weather events, including hurricanes, tornadoes, floods, and wildfires, represents a crucial application of AI. AI algorithms are capable of analyzing vast datasets comprising historical weather data, satellite imagery, and other pertinent information in order to identify potential precursors and patterns associated with these events.
This enables the enhancement of early warning systems and facilitates the implementation of timely evacuations and other protective measures. Additionally, AI can assist in forecasting the intensity and trajectory of these events, which is of paramount importance for minimizing their impact.
Climate Modeling and Prediction
The role of AI in climate modeling and prediction is becoming increasingly significant. Climate models are sophisticated simulations of the Earth's climate system that are employed to project prospective climate change scenarios.
By analyzing extensive climate datasets and simulating intricate climate processes, artificial intelligence can enhance our comprehension of climate change and its prospective consequences. Additionally, AI can be employed to enhance the parameterization of climate models, representing processes that are insufficiently resolved to be explicitly represented, and to diminish the computational expense associated with executing these intricate simulations.
Benefits of AI in Weather Prediction
The integration of AI into weather forecasting offers numerous benefits:
Improved Accuracy: AI can significantly improve the accuracy of weather forecasts across various timescales, particularly for short-term predictions and extreme weather events, leading to better decision-making and reduced economic losses.
Enhanced Lead Times: AI can provide earlier warnings for severe weather, giving individuals, communities, and emergency services more time for preparation and mitigation, potentially saving lives and property.
Reduced Uncertainty: AI can generate probabilistic forecasts, quantifying the uncertainty associated with predictions and enabling better risk assessment and decision-making under uncertainty. This allows users to understand the range of possible outcomes and their likelihood.
Increased Efficiency: AI can automate certain aspects of weather forecasting, such as data processing, quality control, and even the generation of forecast products, freeing up human forecasters to focus on more complex tasks like interpretation, communication, and research.
Improved Communication: XAI techniques can make AI-driven forecasts more transparent and understandable, improving communication with the public and decision-makers, increasing trust, and facilitating better-informed decisions.
Challenges of AI in Weather forecasting
Despite its potential, AI in weather forecasting faces several challenges:
Data Quality and Availability: AI algorithms require large, high-quality datasets for training and validation. Data gaps, inconsistencies, and biases in historical data can limit the performance of AI models and lead to inaccurate predictions. Furthermore, access to high-resolution data, especially in developing countries, can be limited.
Computational Resources: Training and running complex AI models, especially deep learning models, can require significant computational resources, including powerful GPUs and large amounts of memory. This can be a barrier for smaller organizations and researchers with limited access to such resources.
Interpretability and Explainability: While XAI is making progress, understanding how complex AI models arrive at their predictions can still be challenging, hindering trust and acceptance among forecasters and users. This is particularly important for high-impact decisions related to extreme weather events.
Generalizability: AI models trained on one geographic region or type of weather event may not perform well in other contexts. A key challenge is developing models that can generalize across different regions and weather phenomena.
Bias in Data: AI models can inherit biases present in the training data, leading to inaccurate or unfair predictions. For example, if the training data primarily represents weather patterns in developed countries, the model may not perform well in developing countries with different climates and weather patterns.
Real-World Examples of AI in Weather Forecasting
Several organizations are already using AI in operational weather forecasting:
Google's DeepMind: Developed a nowcasting model called "DGMR" that utilizes deep generative models to predict precipitation up to two hours ahead. DGMR has been shown to outperform traditional nowcasting methods in terms of accuracy and lead time.
The National Oceanic and Atmospheric Administration (NOAA): Utilizing AI to improve hurricane track and intensity forecasts, as well as to predict other severe weather events like tornadoes and winter storms. NOAA is also exploring the use of AI for improving data assimilation and numerical weather prediction.
The European Centre for Medium-Range Weather Forecasts (ECMWF): Exploring the use of AI for various forecasting applications, including ensemble forecasting, data assimilation, and the prediction of sub-seasonal to seasonal climate variability.
ClearSky Analytics: Uses AI to provide specialized weather forecasts for the renewable energy sector, helping optimize wind and solar power generation.
ClimaCell: Leverages AI and a dense network of IoT sensors to provide highly localized and accurate weather forecasts for businesses and individuals.
The Future of AI in Weather Forecasting
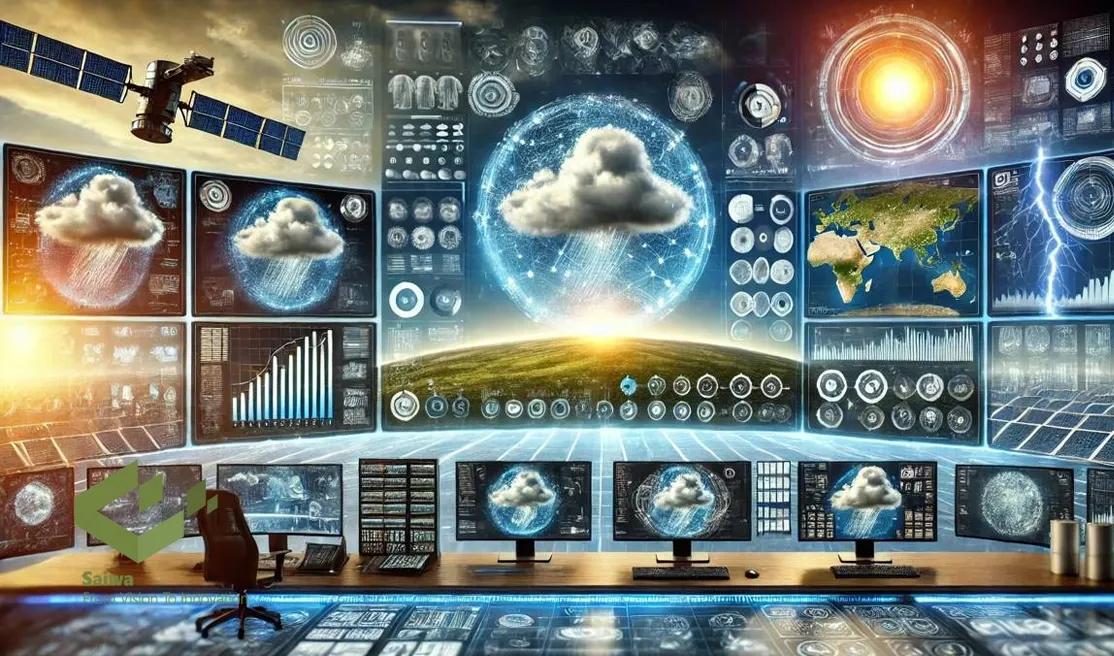
The prospective role of AI in meteorological forecasting is promising. As AI algorithms become more sophisticated, datasets become larger and more comprehensive, and computational resources continue to increase, it is anticipated that AI will play an even greater role in improving the accuracy, timeliness, and usefulness of weather predictions.
Further advancements in XAI will foster greater trust and transparency, thereby facilitating the broader adoption of AI-driven forecasts. Moreover, the incorporation of AI with other nascent technologies, such as the Internet of Things (IoT), which furnishes copious and real-time environmental data, and high-performance computing, which facilitates the execution of more intricate models at higher resolutions, will further augment our capacity to comprehend and anticipate meteorological phenomena. This will result in the generation of more precise and targeted forecasts, thereby facilitating more effective decision-making across a range of sectors and enhancing our resilience to weather and climate-related risks.
Conclusion
Artificial intelligence is transforming the field of weather forecasting, offering the potential for unprecedented accuracy and insights. By employing the capabilities of machine learning, data assimilation, ensemble forecasting, and other sophisticated techniques, AI is transforming the manner in which weather events are predicted and responded to. Although challenges persist, the future of AI in weather forecasting is promising.
It offers a more accurate and detailed understanding of atmospheric conditions, which in turn enables more effective preparation for the challenges and opportunities that lie ahead. As AI continues to evolve and integrate with other technologies, it is reasonable to anticipate further significant advancements in weather forecasting, which will contribute to a safer and more sustainable future.