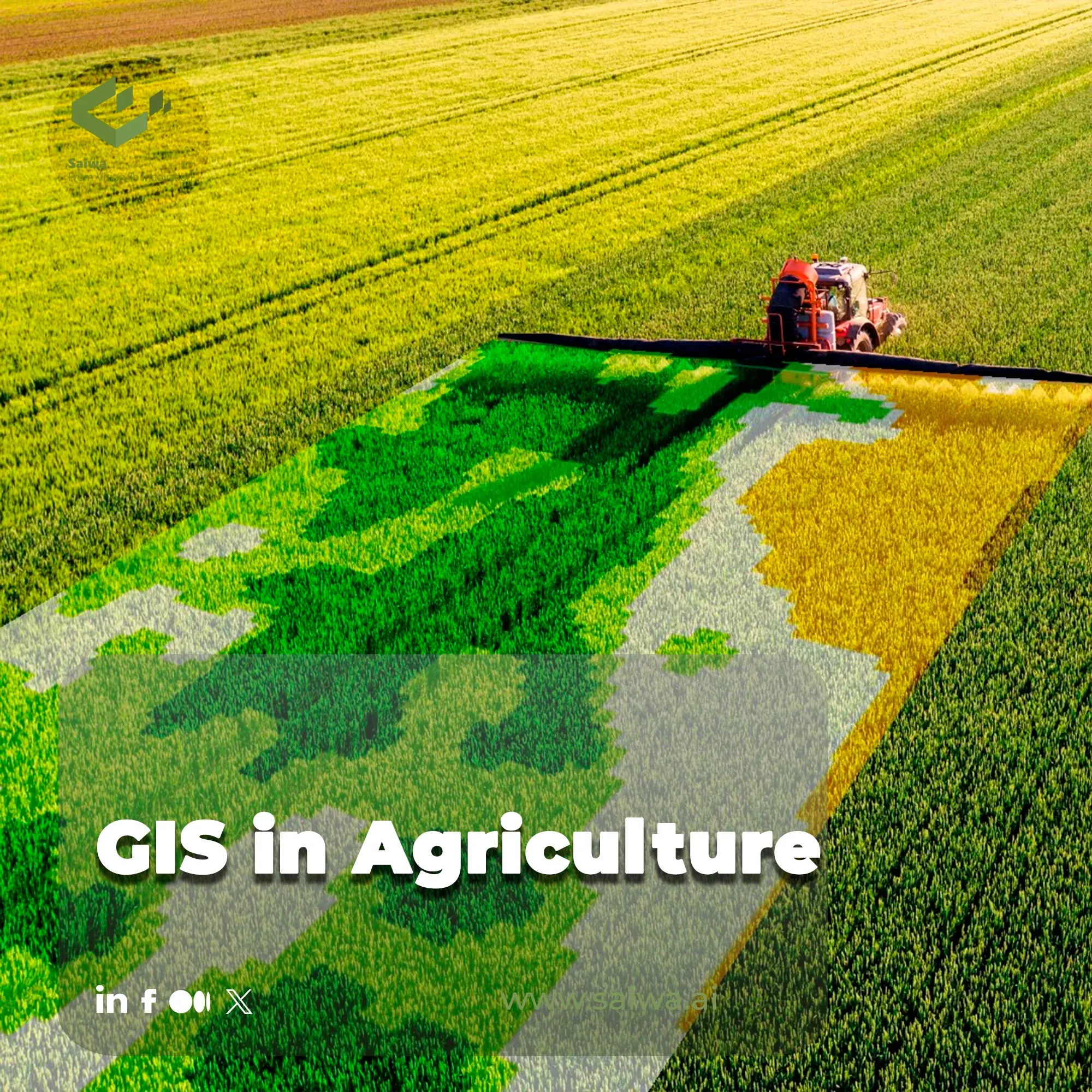
The agricultural sector faces numerous challenges in the 21st century, including increasing demand for food production, environmental sustainability concerns, and the need to optimize resource use. Geographic Information Systems (GIS) have emerged as powerful tools that can empower agricultural stakeholders to address these challenges effectively. GIS integrates spatial data about land, soil, crops, and climate with traditional agricultural information. This holistic approach provides a comprehensive understanding of agricultural landscapes, aiding decision-making from individual farms to regional planning initiatives. By leveraging GIS, farmers can enhance crop management, optimize resource allocation, and adopt sustainable agricultural practices, ultimately contributing to food security and environmental preservation.
Sairone is an integrated ML/AI drone platform developed by Saiwa, designed to revolutionize agriculture through advanced data analysis and environmental monitoring. As a data analysis tool, Sairone harnesses the power of artificial intelligence and machine learning to process vast amounts of agricultural data. This innovative platform combines drone technology with cutting-edge algorithms to provide real-time insights into crop health, soil conditions, and environmental factors. By utilizing Sairone, farmers can make informed decisions, improve resource efficiency, and enhance productivity. The platform's capabilities in precision agriculture and site-specific management enable optimized interventions, ensuring sustainable and efficient agricultural practices for the future.
What Is GIS In Agriculture?
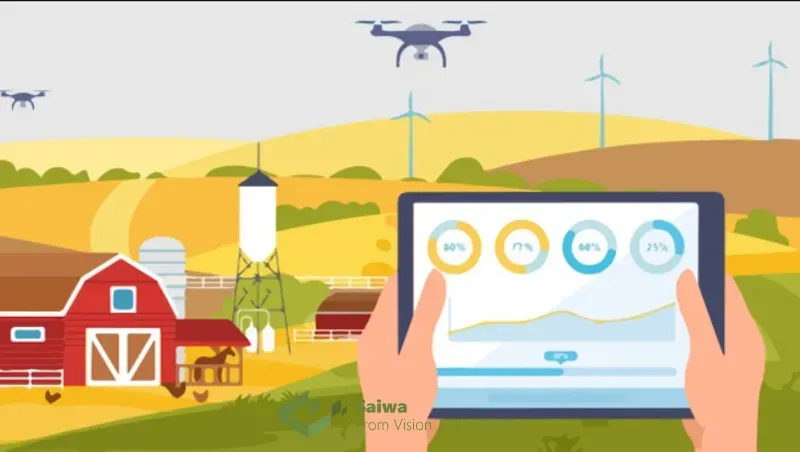
Geographic information systems (GIS) are computer-based systems that facilitate the capture, storage, analysis, and management of geographic data. In the context of agriculture, GIS integrates spatial information about land, soil, crops, climate, and other relevant factors with traditional agricultural data. This enables a comprehensive grasp of agricultural landscapes, thereby facilitating well-informed decision-making at diverse levels, from the individual farm to regional planning initiatives.
History of GIS in Agriculture
The story of GIS in Agriculture is one of innovation and efficiency. While the roots of mapping and land management stretch back centuries, the formal marriage of GIS technology with agriculture began in the early 1960s. The use of GIS in Agriculture started when Canada developed one of the first applications to manage land use and create maps for their national inventory. This marked a turning point, demonstrating the potential of GIS to revolutionize agricultural practices.
Following this initial spark, the use of GIS in Agriculture slowly gained traction. Early applications primarily focused on data collection and generating basic land-use maps. These maps provided farmers with a more comprehensive picture of their land, offering insights into soil types, topography, and historical land use patterns. This fundamental information laid the groundwork for future advancements.
Throughout the late 20th century, advancements in computer technology and data analysis techniques continued to fuel the development of GIS in Agriculture. The ability to store and manipulate large datasets became increasingly accessible, allowing for more sophisticated applications. This period also saw the rise of specialized software programs designed specifically for agricultural use.
By the turn of the 21st century, GIS in Agriculture was poised for a significant leap forward. The emergence of technologies like GPS and remote sensing further expanded the capabilities of GIS. This paved the way for the applications we see today, such as precision agriculture and real-time field monitoring.
The story of GIS in agriculture caused a revolution and innovation in this industry. From its beginnings as a data collection tool, it has evolved into a powerful force shaping the future of agriculture.
Read More: Drones and GIS Mapping | Best Drones for Aerial Precision
Data Acquisition and Management
Effective utilization of GIS in agriculture hinges on the quality and availability of spatial data. Here's an overview of common data acquisition and management techniques:
Remote Sensing and Satellite Imagery
Multispectral and hyperspectral imaging
Satellites and airborne sensors collect data across a range of wavelengths within the electromagnetic spectrum. Multispectral imagery provides information on visible, near-infrared, and shortwave infrared bands, thereby enabling the analysis of vegetation health, crop growth stages, and land use patterns. Hyperspectral imagery captures data across hundreds of spectral bands, thereby facilitating the acquisition of more detailed insights into crop health ,Crop Disease Detection and biophysical properties.
Vegetation indices and crop monitoring
By analyzing specific combinations of spectral bands in satellite imagery, scientists can derive vegetation indices that quantify parameters such as leaf area index, chlorophyll content, and biomass. These indices are of great importance for the monitoring of crop health, the tracking of crop growth cycles, and the identification of areas exhibiting potential stress or yield decline.
Aerial photography and UAV/drone data
The utilisation of high-resolution aerial photographs and data obtained from unmanned aerial vehicles (UAVs), colloquially termed 'drones', provides a comprehensive insight into the delineation of field boundaries, the configuration of infrastructure, and the condition of crops. These data sources are of particular value in the creation of precise field maps and the monitoring of small-scale variations within agricultural landscapes.
Read Also: Satellite and Drone Imagery | A Comparative Analysis
Geographic Data Collection and Field Mapping
GPS and GNSS technologies
Global Positioning Systems (GPS) and Global Navigation Satellite Systems (GNSS) facilitate the real-time acquisition of geospatial data in the field. Farmers may utilize handheld GPS devices to document field boundaries, soil sampling locations, and other pertinent points of interest. Subsequently, the data can be integrated with a geographic information system (GIS) for the purposes of spatial analysis and visualization.
Mobile GIS and data collection apps
The advent of mobile computing has enabled the use of smartphones and tablets for the collection of data in the field, facilitating the collection of data in real-time and in situ. Mobile GIS applications permit farmers to capture location data, record observations, and take photographs, all of which can be seamlessly integrated with cloud-based GIS platforms.
Crowdsourcing and participatory mapping
Crowdsourcing initiatives and participatory mapping approaches can be valuable for the collection of spatial data in agriculture. Local communities can contribute data on crop types, agricultural practices, and traditional ecological knowledge, thereby enriching the available information for informed decision-making.
Data Management and Geodatabases
Spatial data formats and standards
Spatial data comes in various formats, such as shapefiles, geodatabases, and raster images. Standardized formats ensure interoperability between different GIS software packages and facilitate data sharing.
Database design and management
Designing and managing geodatabases is crucial for efficiently storing, organizing, and querying spatial data related to agricultural operations. Effective database design considers the type, scale, and accuracy of the data, along with the specific needs of agricultural decision-making.
Cloud-based and distributed GIS platforms
Cloud-based GIS platforms offer flexible and scalable solutions for data storage, management, and collaboration. These platforms enable farmers and agricultural organizations to access and share data remotely, fostering collaboration and promoting data accessibility.
Spatial Analysis and Modeling
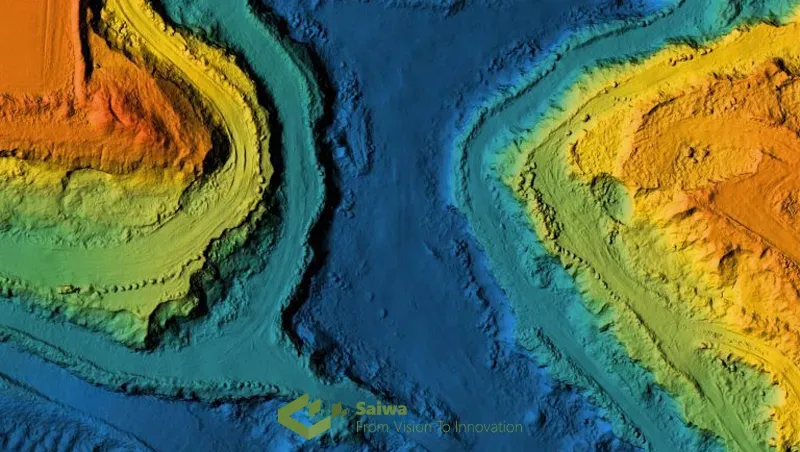
GIS empowers users to conduct spatial analysis and modeling, transforming raw data into actionable insights for agricultural applications. Here are some key examples:
Terrain and Topographic Analysis
Digital Elevation Models (DEMs)
Digital Elevation Models (DEMs) represent the elevation or topography of a landscape. GIS can utilize DEMs for slope analysis, aspect mapping (the direction a slope faces), and watershed delineation, all of which are crucial factors for understanding water flow, soil erosion potential, and microclimatic variations within agricultural landscapes.
Slope, aspect, and watershed delineation
The influence of slope and aspect on factors such as sun exposure, soil drainage, and wind erosion are a significant one. Geographic information systems (GIS) can be utilized to analyze digital elevation models (DEMs) in order to generate slope and aspect maps, which can then be employed to inform decisions pertaining to crop suitability, irrigation planning, and the placement of agricultural infrastructure. Similarly, watershed delineation assists in the identification of the natural drainage areas within a landscape, which is of great importance for the comprehension of water flow patterns, the administration of irrigation systems, and the mitigation of soil erosion risks.
Soil Erosion and Runoff Modeling
Terrain characteristics like slope, aspect, and vegetation cover significantly influence soil erosion and runoff processes. GIS can integrate DEMs with soil type data and rainfall patterns to model soil erosion potential across a field. This information is vital for implementing soil conservation practices, such as terracing or cover cropping, to minimize soil erosion and protect agricultural productivity.
Crop Suitability and Land Evaluation
Soil mapping and classification
Soil properties like texture, drainage, and nutrient content significantly influence crop growth and yield potential. GIS can integrate soil survey data and other relevant information to create detailed soil maps, which are essential for land evaluation and crop suitability analysis.
Crop suitability analysis
By overlaying information on soil characteristics, climate data, topography, and economic factors, GIS can generate crop suitability maps. These maps indicate the areas within a landscape that are most suitable for growing specific crops based on their ecological requirements and economic viability.
Land capability and suitability assessment
Land capability classification systems categorize land based on its suitability for sustained agricultural production. GIS can integrate various factors to assess land capability, supporting informed decisions on land use planning and identifying areas with potential for agricultural expansion.
Precision Agriculture and Site-Specific Management
Yield mapping and variability analysis
Yield mapping involves collecting data on crop yields across a field using combine harvesters equipped with GPS technology. GIS can analyze yield data to identify spatial patterns of variability within a field. This information can be used to identify areas with low or high yields, allowing for targeted interventions to optimize resource use and improve overall productivity.
Variable rate technology (VRT)
Variable rate technology (VRT) utilizes yield maps and other spatial data to adjust the application of inputs like fertilizers, pesticides, and irrigation water across a field. By applying inputs only where necessary, VRT can optimize resource use, reduce costs, and minimize environmental impact.
Precision irrigation and nutrient management
GIS can integrate data on soil moisture, crop water requirements, and weather forecasts to optimize irrigation scheduling. Similarly, by analyzing soil nutrient content and crop needs, GIS can guide the application of fertilizers, ensuring optimal nutrient delivery and minimizing environmental risks associated with excess fertilizer use.
How Is GIS Used In Agriculture?
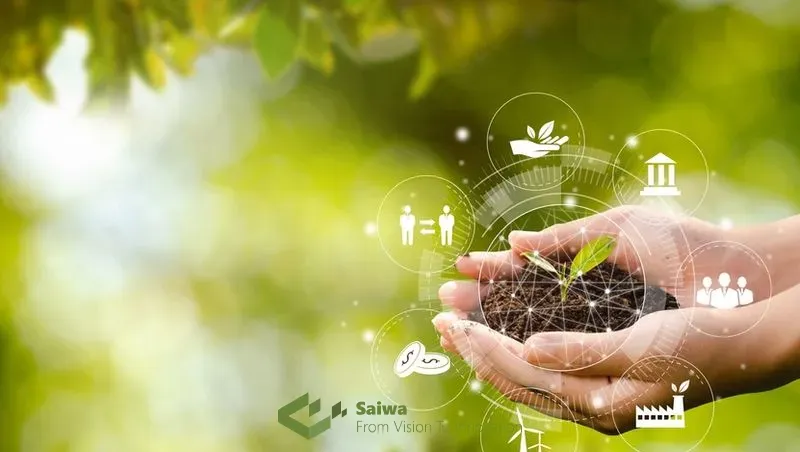
The applications of GIS in agriculture are vast and continuously evolving. Here's a closer look at some key areas where GIS plays a vital role:
Environmental and Resource Management
Water Resource Management
GIS is a powerful tool for water resource management in agriculture. It can be used to assess water availability, identify areas with water scarcity, and plan irrigation systems efficiently. Additionally, GIS can help in modeling water flow patterns, identifying potential sources of water pollution from agricultural activities, and developing strategies for sustainable water management practices.
Biodiversity and Ecosystem Services
Habitat mapping and conservation planning: GIS can be used to map and analyze the distribution of habitats for various plant and animal species. This information is crucial for conservation planning, identifying critical habitats, and developing strategies to mitigate the impact of agricultural activities on biodiversity.
Ecosystem service valuation: Ecosystem services encompass the benefits humans derive from nature, including pollination, water purification, and soil formation. GIS can be used to map and assess the value of these services within agricultural landscapes, informing decisions on land use practices that promote biodiversity and ecosystem health.
Wildlife corridors and connectivity analysis: Habitat fragmentation caused by agricultural expansion can disrupt wildlife movement patterns. GIS can be used to identify wildlife corridors, natural pathways that allow animals to move between fragmented habitats. This information is crucial for conservation planning and maintaining ecological connectivity within agricultural landscapes.
Climate Change and Risk Assessment
Climate modeling and scenario analysis: Climate change poses significant threats to agricultural production. GIS can integrate climate data and projections to model potential future scenarios and assess the vulnerability of agricultural landscapes to climate change impacts like drought, floods, and extreme temperatures.
Crop risk assessment and vulnerability mapping: By analyzing climate data, soil characteristics, and crop types, GIS can identify areas with high risk of crop failure due to climate change. Vulnerability maps can inform adaptation strategies, such as selecting more drought-resistant crop varieties or implementing improved water management practices.
Adaptation strategies and climate-smart agriculture: GIS plays a vital role in developing and implementing climate-smart agriculture practices. It can be used to identify areas suitable for specific climate-resilient crops, design efficient irrigation systems under changing water availability conditions, and monitor the effectiveness of adaptation strategies.
Decision Support Systems and Farm Management
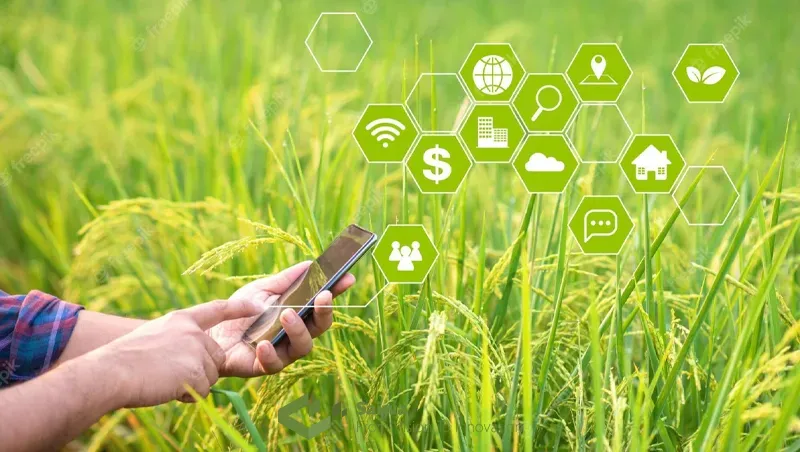
Crop Monitoring and Yield Forecasting
GIS, coupled with satellite imagery and crop growth models, can be used for real-time crop monitoring. This allows farmers to identify potential problems like pest outbreaks or nutrient deficiencies early on, enabling timely interventions to minimize yield losses. Additionally, GIS-based crop forecasting models can utilize historical yield data, weather patterns, and crop health information derived from satellite imagery to predict potential crop yields. This information can be used for informed decisions on harvesting schedules, storage requirements, and marketing strategies.
GIS, coupled with satellite imagery and crop growth models, empowers farmers with real-time insights into their crops. This section explores the various applications of GIS in crop monitoring and yield forecasting:
Crop Growth Modeling and Simulation
Crop growth models are computer simulations that predict crop growth and development based on factors like weather, soil conditions, and crop genetics. GIS integrates these models with spatial data to simulate crop growth across a field, accounting for variations in soil properties, topography, and microclimates. This allows farmers to identify areas that may be lagging in growth and investigate potential causes.
Yield Prediction and Estimation
GIS can be used to estimate potential crop yields throughout the growing season. By analyzing satellite imagery that captures vegetation health and growth stages, along with historical yield data and weather patterns, GIS models can predict potential yield variations within a field. This information enables farmers to make informed decisions on resource allocation, harvesting schedules, and marketing strategies.
Early Warning Systems and Disease Monitoring
Early detection of crop stress or potential outbreaks is crucial for minimizing yield losses. GIS can be used to develop real-time monitoring systems that integrate satellite imagery, sensor data, and weather forecasts. By analyzing changes in vegetation health indicators derived from satellite imagery, GIS can identify areas with potential stress or disease outbreaks. This allows for timely interventions, such as targeted pesticide application, to minimize damage and safeguard crop yields
Farm Planning and Resource Optimization
Farm boundary delineation and field zoning: GIS can be used to accurately map farm boundaries, define field sizes and shapes, and create detailed zoning plans for different agricultural activities. This information is crucial for farm management tasks like resource allocation, planning crop rotations, and optimizing machinery use.
Machinery and logistics planning: GIS can assist in planning efficient routes for agricultural machinery, considering factors like field locations, terrain variations, and access points. This can optimize logistics, minimize fuel consumption, and reduce operational costs.
Optimal route planning and scheduling: Optimizing routes for farm machinery and deliveries can significantly improve operational efficiency. GIS can analyze road networks, field locations, and traffic patterns to generate optimal routes, saving time and resources.
GIS-based Decision Support Systems (DSS)
Web-based and mobile GIS applications: Web-based and mobile GIS applications provide farmers with real-time access to their farm data and GIS tools on the go. This allows for on-site data collection, monitoring of field conditions, and informed decision-making at the point of need.
Integrated farm management systems: Integrated farm management systems (IFMS) combine GIS capabilities with other farm management software functionalities like recordkeeping, financial management, and resource planning. These systems provide a centralized platform for managing all aspects of a farm operation, fostering data-driven decision making.
Expert systems and knowledge-based modeling: Expert systems leverage GIS with artificial intelligence (AI) to incorporate expert knowledge and best practices into decision-support tools. These systems can guide farmers in making informed decisions on crop selection, pest management, and fertilizer application based on specific field conditions and historical data.
Read Also: An Over View Applications of Computer Vision in Agriculture
Data Visualization and Communication
Effective communication of agricultural data is crucial for knowledge sharing, collaboration, and policy development. GIS offers powerful tools for data visualization:
Cartography and Map Design
Thematic mapping and data visualization: GIS allows for the creation of thematic maps that visually represent various agricultural data layers, such as soil types, crop yields, or areas with high water stress. Effective thematic map design principles ensure clear communication of complex spatial information to a wider audience.
Map projections and coordinate systems: The Earth is a three-dimensional sphere, but maps are two-dimensional representations. Map projections are used to flatten the Earth's surface onto a map, and choosing the appropriate projection is crucial for accurate representation of spatial data in GIS applications related to agriculture.
Web mapping and interactive maps: Web mapping platforms allow for the creation of interactive maps that users can explore and query. This enables stakeholders to dynamically visualize and analyze agricultural data, fostering informed decision-making and collaboration.
Spatial Data Sharing and Collaboration
Geospatial data standards and interoperability: Standardized data formats and protocols are essential for sharing spatial data between different GIS platforms and organizations. This ensures data compatibility and facilitates collaboration among stakeholders in the agricultural sector.
Spatial data infrastructures (SDIs): Spatial Data Infrastructures (SDIs) provide a framework for managing, sharing, and accessing spatial data across various organizations and government agencies. SDIs play a vital role in promoting data accessibility and collaboration in the agricultural domain.
Participatory GIS and community mapping: Participatory GIS (PGIS) approaches involve local communities in collecting and analyzing spatial data related to their agricultural practices and knowledge. This fosters collaboration, empowers communities, and integrates local knowledge into decision-making processes.
Policy and Governance
GIS plays a crucial role in informing agricultural policies and supporting good governance practices:
Agricultural Policy and Subsidy Management
GIS can be used to identify areas most in need of agricultural subsidies or support programs. By analyzing factors like soil quality, land use patterns, and farm income data, policymakers can design targeted interventions to promote sustainable agricultural practices and support smallholder farmers.
Land Use Planning and Zoning
GIS is a valuable tool for land-use planning and zoning in agricultural landscapes. It can be used to identify areas suitable for agricultural expansion, while considering factors like environmental constraints, protected areas, and proximity to infrastructure.
Emerging Technologies and Future Directions
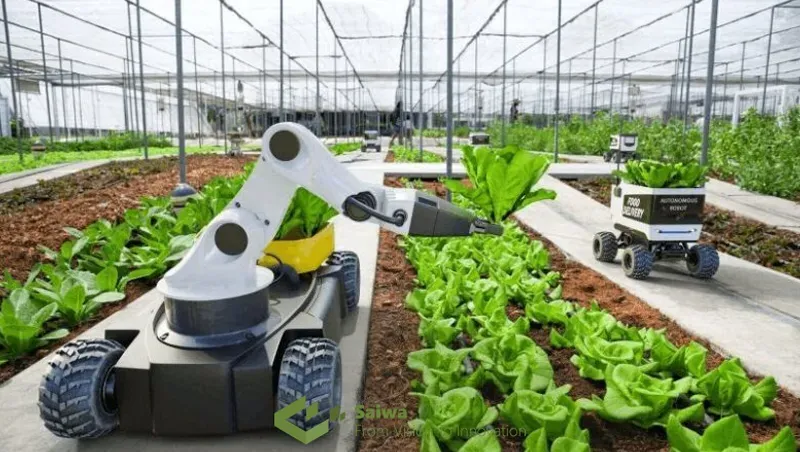
The field of GIS in agriculture is constantly evolving, driven by advancements in technology:
Big Data and Spatiotemporal Analytics
Time-series analysis and change detection: The increasing availability of satellite imagery and other sensor data allows for time-series analysis and change detection in agricultural landscapes. By analyzing trends over time, researchers and agricultural stakeholders can monitor changes in land cover, crop health, and environmental conditions, informing proactive management strategies.
Machine learning and predictive analytics: Machine learning algorithms can be applied to vast agricultural datasets to identify patterns, predict crop yields, and assess potential risks. For instance, machine learning models can analyze historical yield data, weather patterns, and soil properties to predict potential crop failures and inform preventive measures.
Cloud computing and distributed GIS: Cloud computing platforms offer scalable and cost-effective solutions for storing, processing, and analyzing large agricultural datasets. Distributed GIS architectures enable data processing across multiple servers, handling the computational demands of big data analytics in agriculture.
Internet of Things (IoT) and Smart Agriculture
Precision agriculture and smart farming: The Internet of Things (IoT) is a technological paradigm that integrates sensors, actuators, and internet connectivity to facilitate the collection of real-time data from agricultural fields. Such data can be employed in the implementation of precision agriculture practices, including the monitoring of soil moisture and the targeted application of irrigation water. The integration of the Internet of Things (IoT) and geographic information systems (GIS) enables the automation of agricultural operations and the optimization of resource utilization in a process known as "smart farming."
Sensor networks and real-time monitoring: Sensor networks deployed across agricultural fields can collect real-time data on soil moisture, temperature, and crop health. This data can be integrated with GIS for real-time monitoring and informed decision-making. For instance, farmers can receive alerts about potential pest outbreaks or irrigation needs based on sensor data analysis.
Integration of GIS with IoT platforms: Integrating GIS with IoT and agriculture allows for the visualization and analysis of real-time sensor data within a spatial context. This empowers farmers to identify spatial patterns and trends in crop health, soil conditions, and other parameters, enabling them to make data-driven decisions for improved farm management.
Artificial Intelligence (AI) and Automation
Computer vision and aerial/satellite image analysis: AI-powered computer vision techniques can be applied to analyze aerial and satellite imagery for automated crop identification, weed detection, and disease monitoring. This can significantly improve efficiency and accuracy in field scouting tasks.
Autonomous systems and robotics in agriculture: Autonomous agricultural robots, equipped with sensors and artificial intelligence capabilities, are capable of performing a range of tasks, including weed control, crop harvesting, and soil sampling. Such technology can reduce labor costs, improve operational efficiency, and minimize human exposure to hazardous agricultural chemicals.
AI-driven decision support systems: The integration of AI with GIS and agricultural data enables the development of intelligent decision support systems. Such systems are capable of analyzing extensive datasets, identifying patterns, and suggesting optimal management practices for farmers based on specific field conditions and real-time data.
Read More: Practical Application & Future of AI in Agriculture
Data Quality, Standards, and Interoperability
The quality, accessibility, and interoperability of spatial data are crucial for the successful application of GIS in agriculture:
Spatial Data Quality and Uncertainty
Spatial data can be subject to errors and uncertainties. Understanding the quality and limitations of data is essential for interpreting results and making informed decisions. GIS tools can be used to assess data quality and propagate uncertainty through analyses.
Geospatial Data Standards and Interoperability
Standardized data formats and protocols ensure seamless data exchange between different GIS platforms and organizations. Promoting data standards and interoperability is essential for facilitating collaboration and knowledge sharing in the agricultural sector.
Legal, Ethical, and Social Considerations
The application of GIS in agriculture raises several legal, ethical, and social considerations:
Privacy and Data Protection
The collection and use of agricultural data raise concerns about privacy and data protection. It is crucial to implement robust data security measures and obtain informed consent from farmers before collecting and using their data.
Intellectual Property Rights and Data Ownership
Clear ownership rights and access controls regarding agricultural data are essential. Farmers should have control over their data and be able to benefit from its use.
Digital Divide and Access to Technology
The digital divide can limit access to GIS and related technologies for some farmers. Efforts are needed to bridge the digital divide and ensure equitable access to these tools for all agricultural stakeholders.
Conclusion
Geographic information systems (GIS) have emerged as a powerful tool that transforms agricultural data into actionable insights, empowering stakeholders to make informed decisions at various scales. From optimizing the utilization of resources and enhancing farm management to informing policy development and promoting sustainable agricultural practices, GIS plays a pivotal role in addressing the challenges confronting the agricultural sector in the 21st century. As technology continues to evolve, with advancements in big data analytics, artificial intelligence, and the Internet of Things, GIS will undoubtedly play an even more transformative role in shaping the future of agriculture.