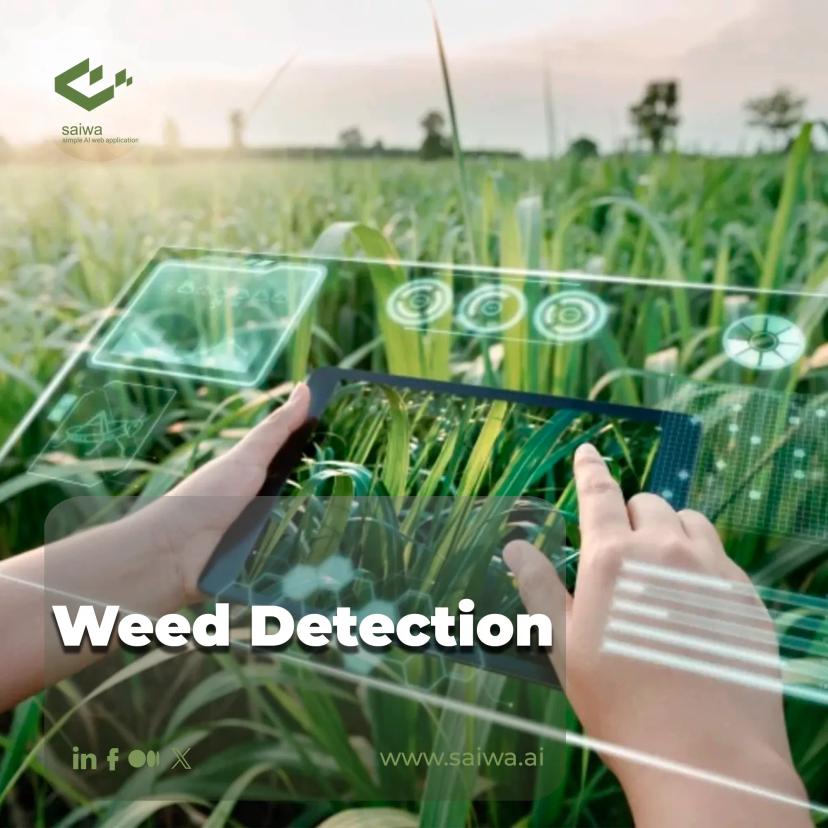
Weed Detection | Comprehensive Overview
Weeds are defined as unwanted plants that compete with desired crops for vital resources, including water, nutrients, and sunlight. Weeds can significantly reduce crop yields and quality, resulting in substantial economic losses for farmers. It is therefore evident that effective weed control is essential for maximizing agricultural productivity and ensuring food security. Traditional weed management practices have historically relied on manual removal, mechanical cultivation, and herbicide application. Nevertheless, these methods are often labor-intensive, time-consuming, and may have potential environmental drawbacks.
Traditional Weed Detection Methods
Manual Scouting: This traditional method involves visually inspecting fields to identify and locate weeds. While effective for small areas, it is labor-intensive and impractical for large-scale farms.
Mechanical Weed Control: Techniques like tilling and harrowing can disrupt weed establishment and growth. However, these methods can also disturb soil structure and increase erosion risks.
Herbicide Application: Herbicides are chemical agents that target and kill weeds. While effective, they can have unintended consequences, such as harming beneficial insects or contaminating water sources. The overuse of herbicides can also lead to the development of herbicide-resistant weed populations.
The limitations of traditional methods necessitate the exploration of more efficient, targeted, and sustainable approaches for weed detection and control. This is where advancements in artificial intelligence (AI) and image processing offer promising solutions.
Weed Detection using the Sairone Platform
Several commercially available platforms, like Sairone, leverage AI-powered weed detection for precision agriculture. These platforms typically provide users with a web interface or mobile application for uploading images of fields or specifying areas of interest. The platform utilizes pre-trained AI models to analyze the uploaded images and identify weeds within the crop canopy. The user receives a weed detection map or report highlighting the location and extent of weed infestation within the field. These platforms offer user-friendly interfaces and can be valuable tools for farmers seeking a convenient and objective method for weed detection.
Fundamentals of Artificial Intelligence in Weed Detection
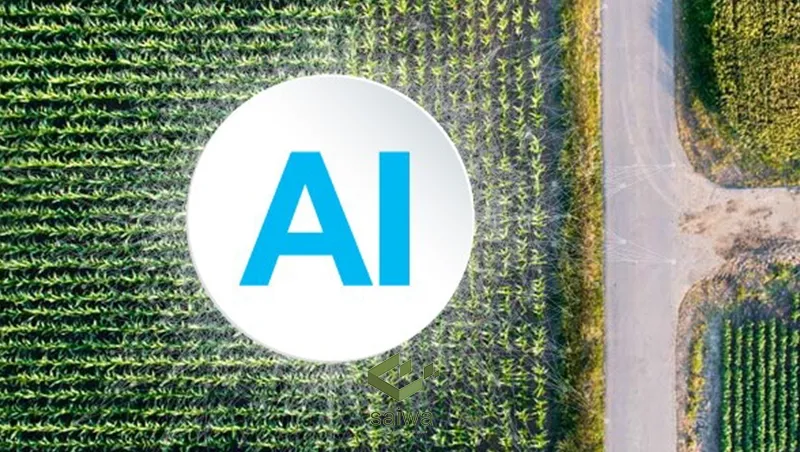
Artificial intelligence (AI) techniques, particularly machine learning and deep learning, play a pivotal role in automated weed detection systems. The following section presents a detailed analysis of the core concepts involved:
Weed Detection Using Machine Learning (ML): Machine learning (ML) algorithms are designed to learn from labeled training data, with the objective of identifying patterns and relationships. In the context of weed detection, machine learning (ML) algorithms are trained on datasets comprising images of various crops and weeds. The trained model is then able to analyze new images and classify pixels belonging to crops or weeds.
Weed Detection Using Deep Learning (DL): A subfield of machine learning, deep learning employs artificial neural networks with multiple layers to identify complex patterns within data. Convolutional neural networks (CNNs) represent a specific type of deep learning architecture that is particularly well-suited for image recognition tasks, such as weed detection. Convolutional neural networks (CNNs) are capable of automatically learning relevant features from images through a series of convolutional layers, which enables the accurate classification of weeds.
Image Processing and Computer Vision in Weed Detection: These techniques are of paramount importance in preparing images for analysis by artificial intelligence. The detection of weeds in images is achieved through the application of image processing techniques, which involve the reduction of noise, correction of color, and segmentation in order to enhance image quality and isolate regions of interest containing crops and weeds. Weed detection using computer vision algorithms then extracts relevant features from the images, including color, texture, and shape characteristics. These features are then used as input for the AI models for weed detection and classification.
Dataset Preparation and Annotation for Weed Detection Models: The efficacy of AI models is contingent upon the quality and diversity of the training data. Datasets for weed detection comprise images of a variety of crops and weed species captured under a range of lighting conditions, growth stages, and field backgrounds. It is imperative that these images be meticulously annotated by experts in order to identify and label weed pixels, thereby providing the ground truth for the training process.
Types of AI-Based Weed Detection Systems
AI-based weed detection systems can be categorized based on the platform used for image acquisition:
Drone-Based Systems: Unmanned Aerial Vehicles (UAVs) or drones equipped with high-resolution cameras can capture aerial images of large fields. These images are then fed into AI models to identify and map weed infestations across the entire field. Drone-based systems are particularly valuable for monitoring large-scale farms and identifying weed patches that may be difficult to detect from ground level.
Ground-Based Systems: These systems utilize cameras mounted on tractors, handheld devices, or autonomous robots that navigate through fields capturing images from a ground-level perspective. Ground-based systems offer high-resolution images suitable for detailed weed identification. They can be used for targeted weed control measures in specific areas of a field.
Weed Detection Techniques Using AI
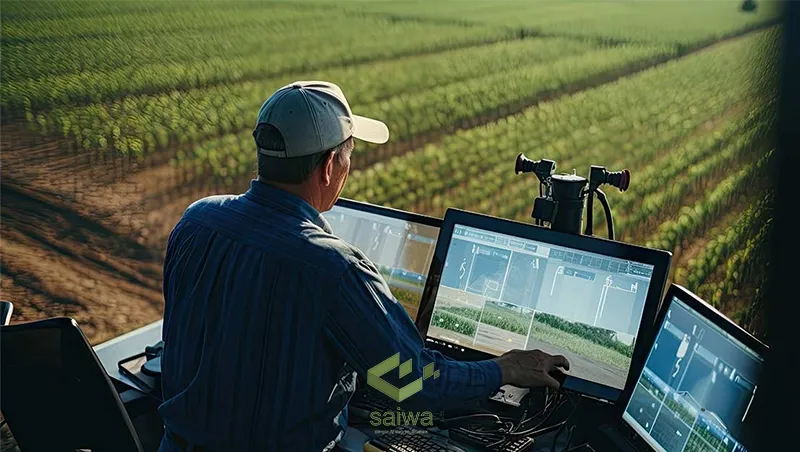
Several AI techniques are employed for weed detection, each offering distinct advantages and limitations:
Object Detection and Segmentation: These techniques aim to identify individual weed plants within an image and differentiate them from crops. Object detection models predict bounding boxes around weeds, indicating their location within the image. Segmentation techniques go a step further, classifying each pixel in the image as belonging to a crop, weed, or background. This provides a more detailed picture of the weed infestation.
YOLOv3 (You Only Look Once v3): A well-known deep learning model for real-time object detection. YOLOv3 offers an optimal balance between accuracy and speed, rendering it an appropriate choice for weed detection in field conditions.
YOLOv5 (You Only Look Once v5): This is an enhanced version of YOLOv3 that offers accelerated processing speeds and the potential for greater accuracy in weed detection tasks.
Saiwa Object Detection Service: The Saiwa Object Detection Service sets the standard in precision agriculture, using AI to transform weed management. Its intuitive interface provides access to state-of-the-art networks such as Detectron2, YOLOv7, and YOLOv5, all trained on the COCO dataset. This enables seamless identification of various objects, including weeds, across agricultural landscapes. In addition, Saiwa offers customization beyond the out-of-the-box, with customized re-training services on specific datasets for maximum accuracy. Users can effortlessly detect weed infestations, enabling targeted intervention to improve crop health and yield. By integrating advanced object detection into agricultural practices, Saiwa provides actionable insights that facilitate sustainable weed control strategies. From early weed detection to precise mapping, Saiwa empowers growers with efficient, productive and environmentally responsible solutions. In addition, Saiwa's cloud-based service streamlines weed detection by requiring users to upload images for analysis using pre-trained models.
Convolutional Neural Networks (CNNs): CNNs are a type of deep learning architecture particularly well-suited for image recognition tasks. They consist of multiple convolutional layers that learn to extract relevant features from images, such as edges, shapes, and textures, which are then used to classify weeds within the image.
Region-based Convolutional Neural Networks (RCNNs): These models build upon CNNs by first generating potential regions of interest (ROIs) within an image that might contain objects. Then, CNNs are applied within each ROI to classify the object present (e.g., weed or crop). While RCNNs offer high accuracy, they can be computationally expensive due to the need to generate ROIs for the entire image.
Fully Convolutional Networks (FCNs): These deep learning architectures perform segmentation tasks by directly predicting the class (weed or crop) for each pixel in the image. FCNs offer advantages over traditional segmentation approaches by being fully convolutional, allowing for efficient processing of images of varying sizes.
Transfer Learning and Pre-trained Models: Deep learning models typically require vast amounts of training data to achieve optimal performance. Transfer learning leverages pre-trained models developed for other image recognition tasks and fine-tunes them for weed detection. This approach significantly reduces training time and computational resources required to develop effective weed detection using machine learning models.
Read Also : Practical Application & Future of AI in Agriculture
AI-based Weed Detection Systems and Applications
The integration of AI into weed detection systems unlocks numerous applications for precision agriculture:
Autonomous Robotic Weed Control Systems
These systems utilize AI weed detection combined with robotic platforms to automate weed removal. Robots equipped with cameras and mechanical weed removal tools can autonomously navigate through fields, identify weeds, and perform targeted removal, minimizing herbicide use and labor costs.
Monitoring and Mapping of Weed Infestations
AI-based systems can be used to generate detailed weed maps of fields. These maps provide valuable insights into the location, extent, and specific weed species present within a field. This information can be used to guide targeted herbicide application or to develop preventative weed management strategies.
Weed Control Optimization
By combining weed detection with variable-rate technology, herbicide application can be optimized to target only areas with weed infestation. This approach minimizes herbicide waste, reduces environmental impact, and promotes sustainable weed management practices.
Early Detection of Invasive Species
AI models can be trained to identify specific invasive weed species. Early detection of these fast-spreading weeds allows for timely intervention and control measures, minimizing their potential to disrupt ecosystems and harm native plant communities.
Real-Life Use Cases of AI in Weed Detection
A large-scale farming operation utilizes a drone-based AI system to monitor weed infestations across thousands of acres of land. The system generates detailed weed maps, allowing for targeted herbicide application and reducing overall herbicide use by 20%.
A vegetable farm integrates a ground-based robotic weed control system into its operations. The robot equipped with AI-powered weed detection autonomously navigates rows of vegetables, identifying and removing weeds with minimal disruption to the desired crop.
A research institution develops a smartphone application that utilizes AI for weed identification. Farmers can upload images of weeds in their fields, and the app provides real-time identification assistance, enabling them to implement appropriate weed control strategies.
Challenges in AI-Based Weed Detection
Despite its significant advantages, AI-based weed detection faces certain challenges:
Data Quality and Variability: The accuracy of AI models heavily relies on the quality and diversity of training data. Variations in lighting conditions, crop varieties, weed species, and growth stages necessitate comprehensive training datasets to ensure robust performance.
Computational Requirements: Developing and running complex deep learning models can require significant computational resources. This can be a constraint for resource-limited farms or applications requiring real-time weed detection in field conditions. Optimizing AI models for efficient processing on edge devices is an ongoing area of research.
Environmental Factors and Field Conditions: Real-world field environments present challenges like dust, shadows, and variations in soil moisture content. AI models need to be robust enough to account for these factors and minimize false positives or negatives in weed detection.
Evolving Weed Resistance: Similar to herbicide resistance, weeds may develop resistance to specific detection algorithms over time. Continuous retraining and adaptation of AI models are crucial to maintain their effectiveness against evolving weed populations.
Integration with Weed Control Systems: Developing seamless integration between AI-based weed detection and automated weed removal systems like robotic platforms presents engineering challenges. Communication protocols and reliable real-time data exchange are essential for effective weed control operations.
Weed Detection based on Position and Edge Features
While AI-based techniques offer significant advantages, it's important to acknowledge traditional methods that may still hold value in specific contexts. One such approach is weed detection based on position and edge features. This method relies on the following principles:
Planned Crop Row Spacing: Crops are typically planted in rows with a predetermined spacing. This allows for the identification of potential weed presence in areas between established crop rows.
Edge Detection: Image processing techniques can be used to detect edges within an image. In the context of weed detection, weeds may appear as distinct edges interrupting the continuity of crop rows.
Pixel Histogram Analysis: Analyzing the distribution of pixel intensities across an image can reveal patterns associated with crop rows. Deviations from these patterns, such as areas with higher intensity variations, could indicate potential weed presence.
While this method may not be as sophisticated as AI-based techniques, it can be a simpler and less resource-intensive approach for weed detection in certain scenarios. For example, it may be suitable for small farms with limited resources or for situations where identifying broad weed patches between crop rows is sufficient for initial weed management purposes.
Conclusion
AI-powered weed detection represents a transformative approach to sustainable weed management in agriculture. AI models offer capabilities for early detection, accurate weed mapping, and optimized herbicide application, leading to improved weed management practices. However, overcoming challenges related to data quality, computational requirements, and environmental variability remains critical for widespread adoption. As AI technology continues to evolve and become more accessible, its integration into agricultural practices holds immense potential for maximizing crop yields, minimizing environmental impacts, and ensuring a sustainable future for our food production systems.