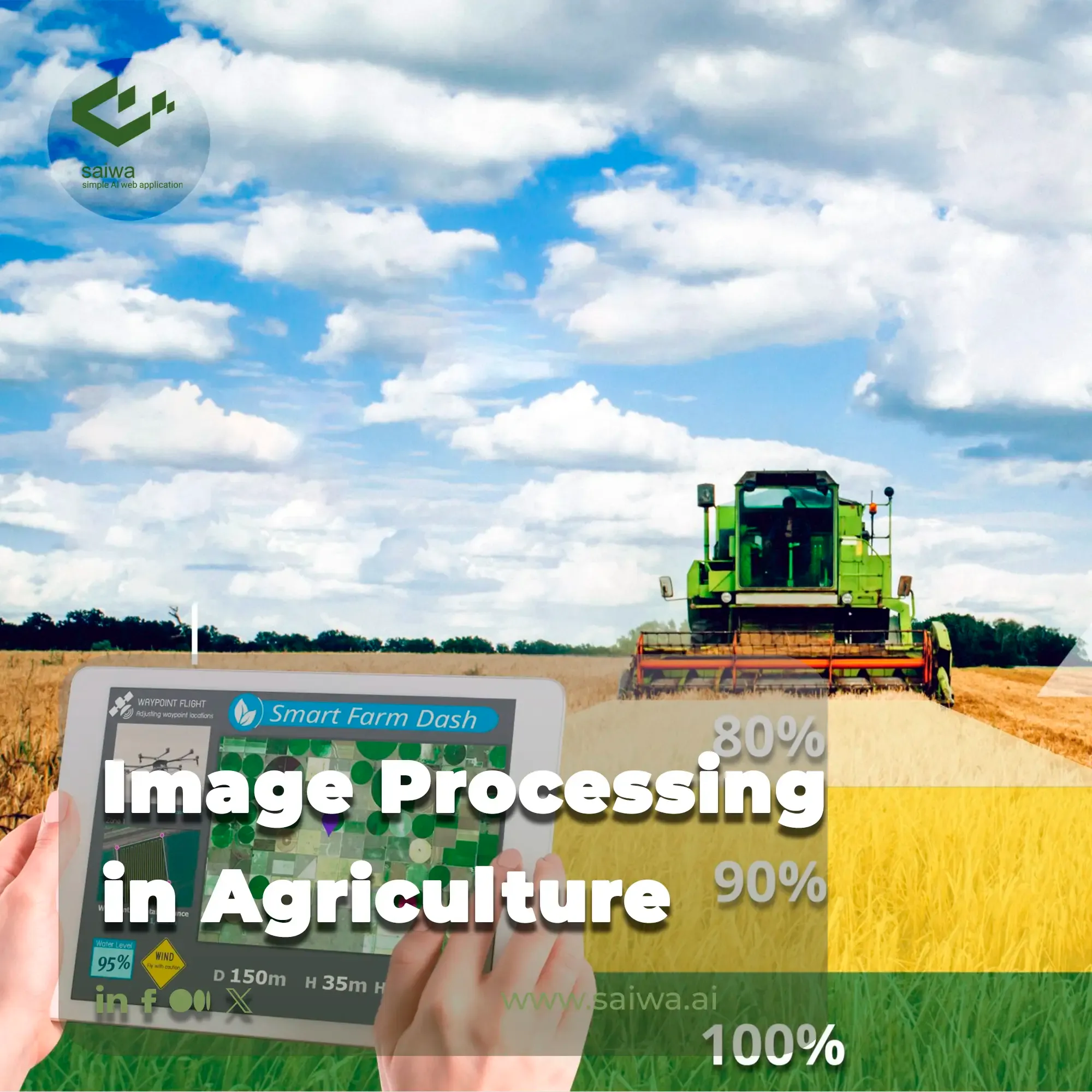
Agriculture is being transformed by advanced digital technologies, including the emerging field of image processing. Image processing involves analyzing visual data like photographs and videos to extract meaningful insights. By applying cutting-edge image processing techniques to agriculture, farmers can gain unprecedented real-time awareness of crop status, soil conditions, and overall farm health. When combined with machine learning algorithms, the agricultural insights unlocked from visual data analysis are poised to revolutionize crop management, disease prevention, yield forecasting and more.
This blog explores the current and potential future applications of image processing in agriculture. It delves into techniques like multispectral imaging, preprocessing steps for agricultural images, machine learning for automated crop analytics, and implementation challenges. With the exponential growth in agricultural imaging data from satellites, drones, and ground-level sensors, image processing will soon provide digital “eyes in the field” to guide data-driven decision making for optimal crop production globally.
What is Image Processing in Agriculture?
Image processing in agriculture refers to the analysis of images captured on farms to gather intelligence on crops, soil, pests, nutrition status and surrounding conditions. Data sources include aerial imagery from satellites and drones as well as ground-level photography from handheld devices and stationary systems mounted on tractors or infrastructure.
Visual data provides unique real-time insights not attainable from numeric soil probe measurements or moisture sensors alone. When processed through specialized algorithms, these images can reveal crop stress, tissue damage, drainage issues, emergence rates and a wealth of other actionable agricultural insights at the speed required for timely interventions. As camera and sensor resolution continue improving alongside advances in computer vision and machine learning, image processing is poised to become an indispensable tool for digital agriculture.
Read Also: Practical Application & Future of AI in Agriculture
Applications in Crop Monitoring and Management
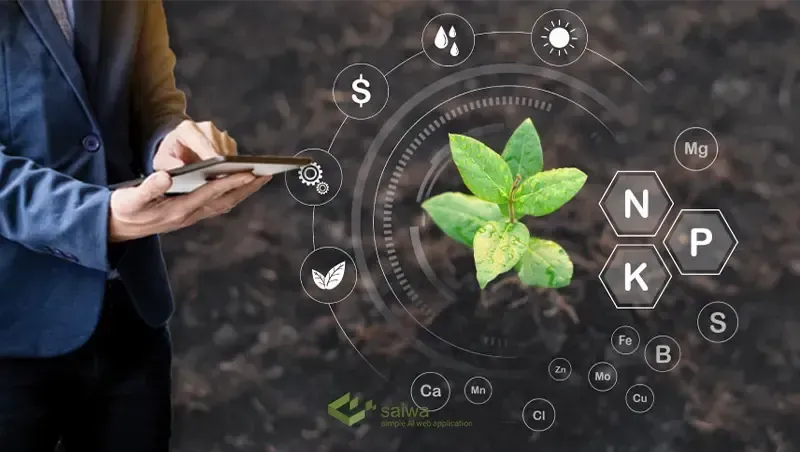
Image processing in agriculture enables several key applications to enhance crop monitoring and data-driven management:
Plant Health Assessment
Visual symptoms in imagery reveal problems like drought stress, pest damage, and nutritional deficiencies before they escalate. Subtle changes in leaf color, texture, size, and shape can be indicators. Measuring vegetation indices like NDVI shows overall plant vigor. Time series analysis detects progressive deterioration. This enables preventative treatment.
Disease and Pest Detection
Bacterial, fungal, and viral crop Disease Detection manifest visibly on leaves, stems, and fruits well before entering advanced stages. Identifying characteristic patterns in images allows early treatment. The same applies to the detection of insects and weeds.
Yield Prediction
As crops develop, algorithms can analyze a number of plants, flowering rates, fruit counts and vegetation density in images to forecast yields for the season. This enables optimized harvest planning and marketing.
Overall, image processing in agriculture generates granular insights to optimize crop management decisions and improve productivity.
Read Also: The Rise of Automated Fruit Sorting Using Image Processing
Image Data Capture
Multiple imaging modalities are used in agricultural image processing:
Satellite Imagery: Public and commercial satellite networks like Landsat provide frequent multi-spectral global image capture ideal for broad-scale crop monitoring, measuring vegetative indices, and identifying field issues.
Aerial Drones: Drones with specialized cameras are used at regular intervals to capture detailed crop imagery for analyzing plant health patterns, growth, and yield outlook across fields.
Proximal Sensing: Mounted systems on tractors or irrigation booms using RGB and multi-spectral cameras gather high-resolution imagery of each crop row to detect localized issues and growth variability.
Handheld/Portable: Agronomists use smartphones and near-range cameras for portable imaging to quickly scout fields for problems, document conditions, and monitor individual plants.
Multispectral: Sensors capturing wavelengths beyond visible light like near-infrared provide specialized imagery revealing plant problems before visible symptoms emerge based on light interaction with foliage.
Thermal: Infrared thermal imaging indicates water stress and can identify over-watering.
Fluorescence: Chlorophyll fluorescence imaging measures photosynthetic efficiency.
Hyper-spectral: Hundreds of narrow bands reveal detailed chemical and biological composition to plant disease identification and nutrition status.
Advances in sensor technology and imaging from multiple perspectives provide rich, complementary visual data on crops for computer analysis.
Preprocessing Steps
To read agricultural images for effective analysis and algorithm training, key preprocessing steps are required:
Geo-registration
Geo-registration accurately maps images to real-world coordinate positions through optical camera models or by aligning to referenced landmarks. This allows integrating multiple images over time or from diverse platforms to track precise field locations. Geo-registration also underpins prescription maps guiding agricultural equipment.
Challenges include distortion from camera angles and lenses, changes in lighting conditions across images, and platform localization errors. Advanced computer vision techniques that match image features or textures enable robust geo-registration.
Noise Filtering, Super-Resolution and Deblurring
Raw agricultural images frequently contain noise from sensor defects, low light levels, and platform vibrations. Image processing in agriculture algorithms filters statistically anomalous pixels to reduce artifacts.
Super-resolution methods combine multiple low-resolution shots to reconstruct higher-fidelity images for finer analysis. Motion deblurring compensates for camera platform instabilities and scene dynamics like the wind to sharpen imagery.
Such corrections ensure optimum quality for subsequent analysis steps. Adapting techniques from medical imaging and satellite platforms continue advancing agricultural image restoration.
Normalizing Multi-seasonal Images
Variability in illumination, weather conditions, soil properties, and phenology differences over multiple growing seasons make comparing agricultural images across years challenging.
Image normalization and domain adaptation methods use color and texture analytics to convert images from diverse seasons into a common representation. This facilitates consistent feature extraction and classification for multi-seasonal time series analysis to distinguish meaningful crop changes from environmental variance.
Ongoing research in multi-modal simulations, style transfers, and adversarial techniques is further overcoming dataset shifts over time.
Image Analysis Applications in Agriculture
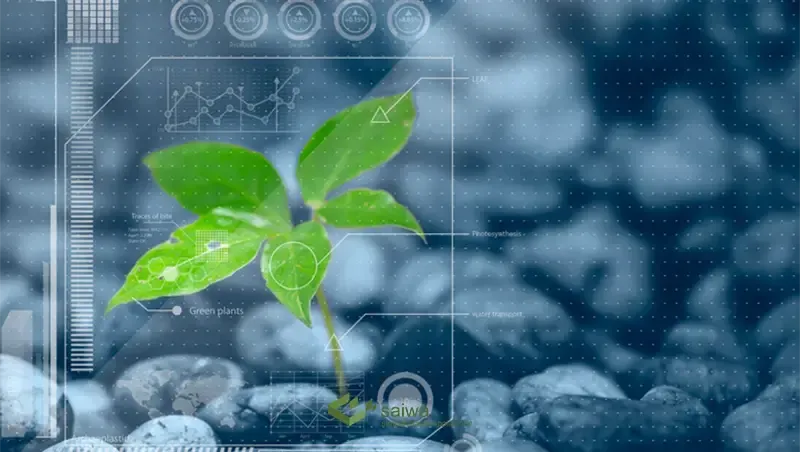
Key applications of agricultural image analysis include:
Detecting Diseased Leaf Patterns for Early Intervention
Advanced computer vision techniques enable automated, large-scale disease detection in crops through image recognition of visual symptoms on leaves, stems, and fruit. Machine learning algorithms can be trained on labeled datasets of imagery containing healthy plants vs various diseases. The models learn subtle visual cues like leaf spots, wilting, chlorosis, or patterns of decay to accurately classify crop health status.
Real-time disease detection from live image feeds or high-throughput processing of batches of field images provides an early warning to growers. This permits applying timely targeted fungicide or bacterial treatments before widespread infection occurs. Geolocating visual detections also facilitates the generating of disease distribution maps to analyze spread patterns. Such precision application saves costs and limits environmental impacts versus broad spectrum spraying of entire fields.
Assessing Crop Density, Spacing, and Size Distributions
Analyzing overhead crop images with smoothing, filtering, semantic segmentation, and object counting algorithms generates key plant morphological and population data. This includes stand density (plants per area), spacing variability, and relative size distributions.
Tracking growth over time can indicate gaps requiring replanting or reseeding for optimal yields. Size variability across plants may reflect non-uniform germination, nutrient levels, or moisture. This enables corrective actions like tailored water, fertilizer, or pesticides.
Identification of Nutrient Deficiencies
Characterizing color changes in foliage with computer vision reveals the onset of macro and micronutrient deficiencies before visible stunting or wilting. Machine learning classification models distinguish healthy spectral signatures from deviations indicating low nitrogen (pale greens), iron (yellows) or sulfur (purples).
Connecting color anomalies with soil properties, fertilization regimes, and yield impacts allows precision nutrient prescriptions. Targeted in-season fertilizer applications or modified programs for successive years then address deficiencies observed in imagery before yields suffer.
Species Recognition Capabilities for Weed Suppression
Automated weed detection leverages computer vision techniques to distinguish crops from interspersed weeds based on textural, structural, and color differences in images. Deep neural networks can classify a wide variety of weed species once trained on representative visual samples.
By pinpointing locations of infestations, chemical or mechanical weed control interventions can be targeted precisely rather than applied broadly across entire fields. Furthermore, identifying spread patterns enables proactive management to limit proliferation. Combining species recognition with growth stage estimation also facilitates optimal timing of weed suppression.
Read Also: An Over View Applications of Computer Vision in Agriculture
The Challenges of Using Image Processing for Agriculture
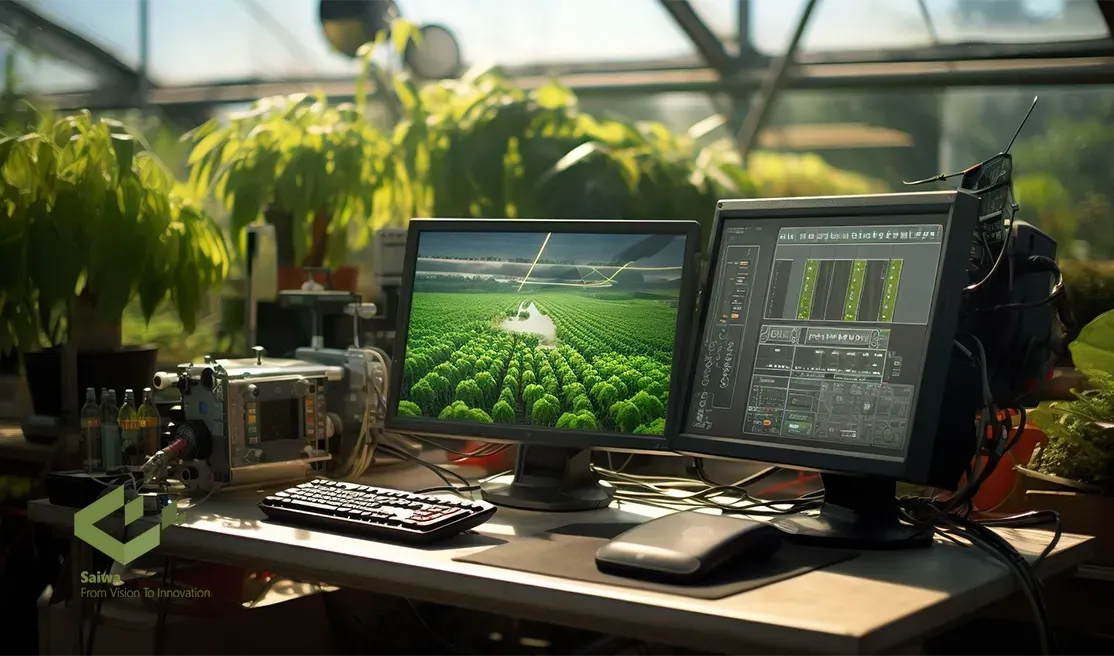
Image processing for agriculture can revolutionize crop management. However, effective implementation of such technology comes with its own difficulties. Let us now look at some of the main problems that are related to the use of image processing for agriculture:
Data Quality Demands
High-quality data is crucial for precise image processing for agriculture. Factors like weather variations, pests, and diseases can significantly impact the visual characteristics of crops, making it difficult for image processing for agriculture algorithms to deliver consistent results. This requires strong image capture methods, correct data pre-processing procedures, and possibly even the implementation of multispectral imaging to address these differences and guarantee accurate crop evaluation.
Limited Training Data Availability
Limited training data availability for specific crops, diseases, or environmental conditions in agriculture can further restrict the generalizability of image processing for agriculture models. This necessitates the development of strategies for data augmentation and transfer learning techniques to overcome this hurdle and ensure image processing for agriculture delivers its full potential.
Integration with Existing Farm Management Systems
Integrating image processing data seamlessly with existing farm management systems is essential for optimizing workflows and decision-making. Challenges arise in ensuring compatibility and efficient data exchange between these systems. Additionally, standardizing data formats across different platforms can further streamline the integration process and unlock the full potential of image processing for agriculture.
Advanced Machine Learning Techniques
Recent advances in artificial intelligence and data science have opened up new possibilities for more sophisticated agricultural image processing. Deep learning neural network models like CNNs can be trained on large annotated image datasets to automatically identify and classify a wide range of crop species and cultivars from visual appearance. Recurrent neural networks analyze multi-temporal images to identify changes in crops over time and predict future states. Deep learning algorithms classify every pixel in images into categories like foliage, fruits, or background to provide richer details.
Combining image analysis data like stand counts and vegetation indices with climate records in machine learning models leads to improved yield prediction accuracy. Algorithms learn normal healthy crop appearance and then automatically flag anomalies in new images that may indicate disease, weeds, or nutrient issues.
AI can extract information on relationships between visual crop symptoms, location, soil conditions, treatments applied, and outcomes to gain agronomic insights. Expert systems can recommend management interventions to farmers based on image analysis and broader field data. Computer vision guides agricultural robots to perform tasks like weed removal and selective harvest based on crop image analysis.
Read Also: The Impact of Machine Learning in Agriculture
Security and Privacy in Agriculture Image Processing
Agriculture image processing is revolutionizing the way farmers manage their fields. This data is crucial for optimizing farm management, but it's also sensitive.
Security Threats
Imagine someone hacking into this system and stealing your data. They could use it for financial gain, like targeting you with scams, or disrupt your operations by messing with planting schedules or resource allocation. That's why robust security measures are crucial throughout the entire process, from capturing the images to analyzing them.
Privacy Concerns
Data privacy is another concern in agriculture image processing. The detailed imagery might reveal information about specific crops, planting patterns, and even farm layouts. No one wants to feel like their data is being exposed. To solve this problem, there are ways to anonymize the data by removing any information that could identify you. Additionally, user access controls ensure that only authorized people can see the data. Farmers deserve to know how their data is used and have a say in who sees it. Clear communication and building trust are key to wider adoption of this technology.
Building a Secure Future
Security and privacy aren't just technical issues; they're essential for gaining farmers' trust and encouraging them to use this technology. By implementing strong security protocols and clear data privacy policies, stakeholders show their commitment to protecting valuable farm data. This transparency builds trust and creates a secure environment for farmers to leverage the power of Agriculture image processing. Ultimately, prioritizing data security and privacy paves the way for the sustainable and ethical growth of this technology. By safeguarding the "eyes in the field," agriculture image processing can empower farmers with valuable insights while protecting their critical data.
Post-Harvest Quality Assessment: Ensuring Excellence with Image Processing
The journey of leveraging image processing in agriculture doesn't conclude at harvest; it continues to add significant value in post-harvest operations, particularly in the critical task of fruit and vegetable grading. Maintaining high quality standards for the market demands consistent and accurate assessment, an area where automated visual inspection systems are making remarkable strides.
Moving beyond the limitations of subjective and labor-intensive manual sorting, these systems employ sophisticated cameras and computer vision algorithms. As produce travels along processing lines, image processing in agriculture techniques analyze various quality attributes with speed and precision. This includes:
Objective Grading: Assessing characteristics like size, shape, and volume far more consistently than human inspectors.
Color and Ripeness Analysis: Utilizing spectral data within images to accurately determine maturity levels, crucial for shelf-life and consumer appeal.
Defect Detection: The identification of surface imperfections, including blemishes, bruises, cuts, and signs of disease that might affect marketability.
By integrating image processing in agriculture into grading lines, agribusinesses can ensure uniform quality, significantly reduce sorting errors, minimize waste, and optimize packing efficiency. This application underscores how intelligent visual analysis is enhancing quality control and efficiency throughout the entire agricultural value chain, from field to final product.
Combining Image Processing with Internet of Things (IoT) for Smarter Farms
The integration of image processing in agriculture with the Internet of Things (IoT) is paving the way for the development of more intelligent and efficient farming practices. By combining these technologies, farmers can enhance their decision-making processes, improve crop yields, and reduce waste. Here’s how this synergy benefits modern farming:
Real-time Data Collection: IoT devices, such as sensors and drones, are capable of collecting vast quantities of data, including environmental conditions and crop health. When integrated with image processing in agriculture, this data can be analyzed to detect crop stress, pest infestations, and nutrient deficiencies instantly.
Automated Monitoring: Internet of Things (IoT) sensors placed throughout the farm, alongside cameras and drones, provide continuous monitoring. Image processing algorithms analyze these images, giving farmers a real-time view of their fields. This helps in early detection of potential problems, reducing the need for manual checks.
Data-Driven Decisions: The integration of the Internet of Things (IoT) and image processing in agriculture enables farmers to make data-driven decisions based on accurate, up-to-date information. This enables optimized irrigation, pest control, and fertilization schedules, which can significantly boost crop production and sustainability.
Predictive Analytics: The integration of these technologies can also enable predictive analytics. By analyzing patterns in the visual data collected over time, farmers can forecast crop yields and plan resources more effectively.
Conclusion
Image processing in agriculture is reaching an inflection point, transitioning from isolated proofs of concept to widespread adoption. With advanced sensors, aerial platforms, ground-level imaging, and advances in computer vision, this rapidly evolving digital toolkit can provide farmers with timely insights on crop status, diseases, nutrient deficiencies, and management needs.
To fully harness the potential of image-driven agriculture, continued focus must be placed on generating labeled training data, standardizing image capture, refining models, integrating insights with other farm data streams, and strengthening grower decision tools. Like the agricultural innovations of the past century, image processing is set to profoundly augment human capacities in managing land and nature sustainably.