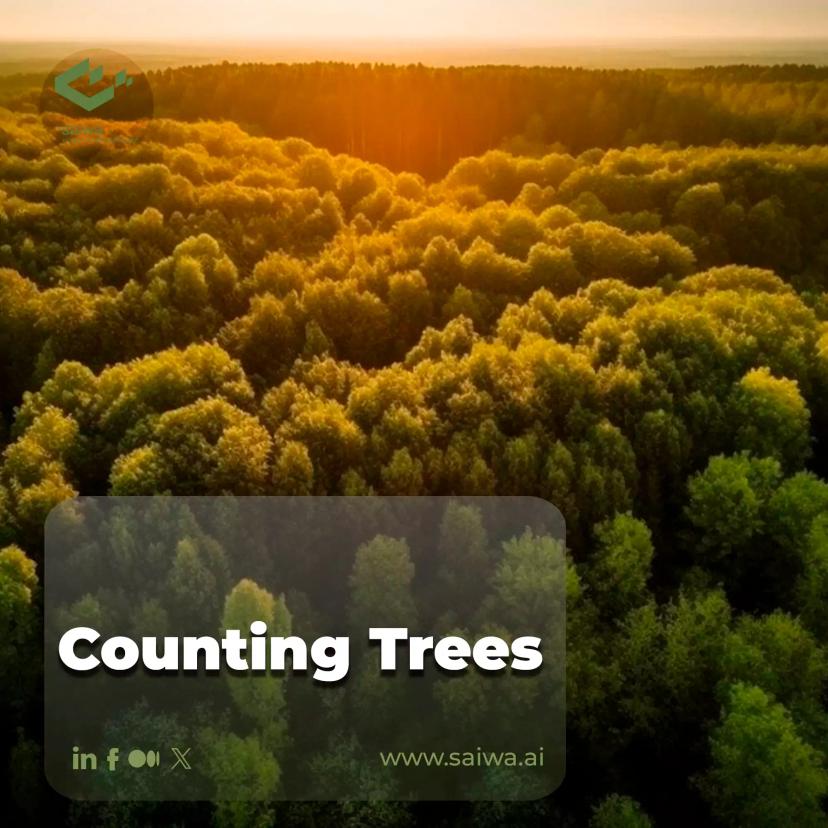
A Guide to Counting Trees | Quantifying Nature
Accurate estimates of tree cover are critical for forest resource assessment, timber inventory management, and carbon stock monitoring. However, traditional methods of counting trees, such as manual surveys or aerial photography, can be time-consuming, labor-intensive, and prone to error. Recent advances in artificial intelligence (AI) offer new opportunities to automate and improve tree counting in diverse forest ecosystems. This blog post provides an overview of AI techniques for tree counting and assesses their potential to expand forest monitoring efforts. Read more: The Surprising Value of Plant Counting
Counting trees is critical for quantifying aboveground biomass, modeling carbon budgets, and making informed forest management decisions. However, manual counting of individual trees over large areas is generally not feasible, while visual interpretation of aerial imagery is inefficient and subject to observer bias. Lidar and other active remote sensing technologies can estimate tree density, but remain costly at regional scales. Computer vision and machine learning algorithms offer promising new tools for analyzing forest structure from both passive and active remote sensing data sources.
Importance of Counting Trees
Accurate tree counts enable the calculation of key forest metrics such as stand density, basal area, and biomass. These underpin timber inventories, carbon accounting, fire modeling, and biodiversity monitoring. For example, combining tree count data with allometric equations and wood density allows estimation of carbon stored in aboveground woody biomass. This is critical for carbon market programs such as REDD+ that aim to mitigate climate change by avoiding forest loss and degradation.
At larger scales, repeated tree counts using statistical sampling can track changes in forest cover, productivity, and disturbance effects. By quantifying tree mortality, recruitment, and growth rates, managers can assess sustainability, plan harvest cycles, and evaluate silvicultural practices. Without accurate tree population data, sustainable forest management is virtually impossible.
Historically, ground-based surveys have provided estimates of the number of trees in local stands. However, complete systematic inventories over large regions are prohibitively time and resource intensive. Manual interpretation of aerial photographs also requires considerable expertise and is prone to subjective bias. These limitations motivate the development of automated AI techniques.
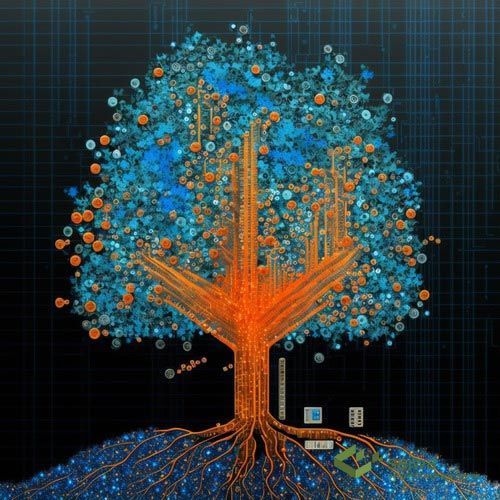
How Do You Count Trees?
There are several standard approaches to quantifying tree populations, with trade-offs between precision, cost, and feasibility at different scales. Field sampling using fixed-area plots is the basis of most forest inventory programs. Statistical methods such as distance sampling are then used to scale plot data to larger areas. However, ground-based techniques remain impractical for continuous regional monitoring.
Aerial photography enables efficient sampling across broader extents. Stereoscopic photo interpretation facilitates tree detection based on height and crown dimensions. However, occlusion and interpreter bias limit accuracy. Photogrammetric techniques can estimate tree heights but not necessarily distinct stems. Lidar provides more direct 3D structure measurements via ranging laser pulses. However, airborne acquisition costs currently constrain Lidar's scalability.
Satellite imagery facilitates wall-to-wall regional mapping but lacks fine details for distinguishing individuals. Pixel sizes of 1-5 meters are typical for commercial systems. No single existing method satisfies all data needs across scales from stands to national inventories. This motivates the development of automated AI techniques that can synergistically integrate diverse data streams.
Manual Tree Counting Methods
Early tree census methodologies relied exclusively on hands-on human direct observation without assistance from aerial perspectives or statistical enhancements. Small acre woodlots allow reasonably accurate manual tallying up to few hundred specimen groupings but consistency and fatigue degrade fidelity in surveying denser old growth areas spanning thousands which technology now ameliorates.
Visual Ground Counts and Tally Sheets
Small well well-defined one acre plots or occasional isolated satellite trees are distributed within eyesight enabling surveyors to continuously meander while incrementing mechanical clicker counters or annotating paper sheets tree-by-tree avoiding double registrations. Beyond small woodlots, density overwhelms manual spot-checking missing obscured subsurface root spreads for incomplete sampling.
Drones And UAVs As Tree Counting Methods
Small quadcopter drones equip various downward facing cameras or sensor arrays producing aerial perspective datasets customizable around flight paths specific to study goals whether prioritizing real-time streamlined enumeration through dedicated optical bark pattern recognition or maximum dimensional capture via LiDAR that intensifies back-end offline processing complexity but delivering enhanced individual tree characteristic accuracy once parsed.
Drones in agriculture counting methodology face challenges around consistency when attempting census spanning different days and locations that variable lighting conditions influence causing data patching difficulties analysts must reconcile manually by tuning batch image adjustments relative to biomarker color or brightness signal continuity so that software algorithm interpretations remain coherent detecting the same wood attributes across separate data capture periods. Some semi-automated reconciliation exists but heavy manual oversight ensures quality control in most pipelines.
Particular flight planning patterns that optimize coverage efficiency pose learning curves for operators balancing mission duration limitations imposed by battery constraints against altitude sweet spots discovering ideal above-ground vantage perspectives revealing unobscured specimens clear enough for software recognition without mixing indistinguishable treetop collages when viewed too remotely.
Read Also: AI-Based Drone Operation| AI in Drones use cases
Counting Trees using AI
A range of AI techniques leveraging both passive optical data and active lidar show promise for automated counting trees. Here we highlight some leading examples:
Machine Learning Image Classification
Recent studies have applied convolutional neural networks (CNNs) to classify overhead imagery into forest/non-forest or estimate tree density and crown size distributions. Data scientists trained a CNN on National Agriculture Imagery Program (NAIP) data across multiple biomes. They found it significantly improved tree crown delineation compared to traditional classifiers. Such semantic segmentation facilitates tree counting but depends on very high-resolution imagery.
Object Recognition
Object recognition with deep learning models like You Only Look Once (YOLO) can pinpoint individual trees in aerial or satellite photos. YOLO trained on plantation images, achieving over 90% accuracy. However, performance declined on novel test sites, indicating challenges generalizing across environments. Data augmentation via rotations and added noise can enhance model transferability.
Active Machine Learning
Lidar provides key structural parameters like canopy height and density, even under vegetation. This active 3D information powerfully complements passive optical data. For example, in 2015, Lidar and aerial imagery combined to segment individual trees with 85% accuracy. The fusion of lidar, optical, and radar data is a promising area of research.
Recent studies also apply unsupervised learning to cluster Lidar returns into individual crowns based on tree architecture. Supervised lidar analysis can also classify returns as tree vs. non-tree points and inform optical image analysis.
3D Deep Learning
New 3D convolutional networks process aerial LiDAR scans and stereo imagery to reconstruct detailed 3D models of trees for trait analysis beyond just crown dimensions.
Multispectral Analysis
Combining optical imagery with infrared, LiDAR, and radar data provides a rich spectrum profile of vegetation for robust tree sensing under variable conditions.
Counting Trees with Machine Learning
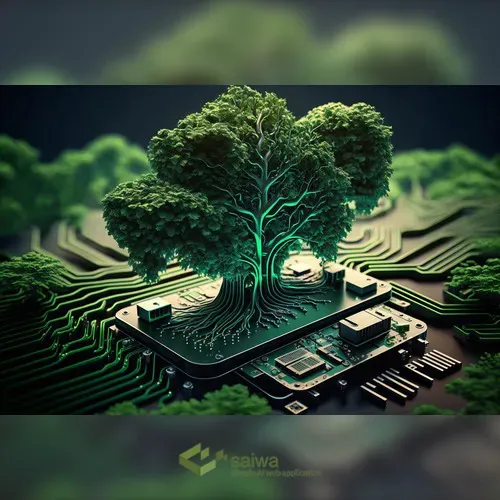
Recent computer vision and machine learning breakthroughs offer new tools to revolutionize tree counting from overhead imagery. Machine learning uses statistical algorithms and large labeled datasets to build models that can analyze complex patterns in data. These powerful predictive models are ideal for tackling challenges like identifying tree crowns against diverse background landscapes.
Two main approaches exist: supervised learning uses input data labeled by humans to train models, while unsupervised learning finds hidden patterns in unlabeled data. In supervised learning, the model learns relationships between input image features and output labels indicating the presence or location of a tree. After sufficient training, the model can classify new unlabeled images.
For tree counting, common supervised tasks include image classification to predict if an image patch contains a tree, object detection in agriculture to pinpoint tree locations within an image, and semantic segmentation to delineate tree crown boundaries at the pixel level. Deep learning neural networks now tackle these challenges more accurately than previous techniques.
Read Also: An Over View Applications of Computer Vision in Agriculture
Data Collection
Remote sensing provides essential data to feed counting tree models. Satellite multispectral imagery offers regional coverage but lacks fine details. Very high-resolution systems like GeoEye provide about 0.5m pixels capable of capturing some crowns. However, frequent revisit cycles to refresh imagery remain costly over large areas.
Low-altitude aerial imagery from drones, planes, or helicopters delivers sub-meter resolution ideal for identifying individuals. However, the limited field of view constrains coverage. Laser scanning yields 3D structures. Lidar penetration capabilities help overcome occlusion. However, airborne acquisition costs are still prohibitive for systematic regional monitoring.
Ground-based Lidar, camera arrays, and terrestrial laser scanning provide high-precision references to calibrate airborne estimates and provide training data. However, in situ collection is sparsely distributed. Fusing these diverse sampling techniques allows for leveraging their complementary strengths.
Integration of Counting Trees with Forest Inventory and Management Systems
The integration of AI-based counting trees methods with existing forest inventory and management systems is crucial for maximizing the benefits of this technology. By seamlessly incorporating the data and insights generated from AI-powered counting trees, forestry professionals can make more informed decisions and streamline their operations.
Forest inventory management systems (FIMS) are essential tools for tracking and maintaining records of forest resources, including tree species, sizes, and locations. By integrating AI-based counting trees techniques, FIMS can be updated with accurate and up-to-date information on tree populations, enabling more precise estimations of biomass, carbon stocks, and overall forest health.
Furthermore, the integration of counting trees data with Geographic Information Systems (GIS) can provide a comprehensive spatial representation of forest resources as mentioned before in the blog. This spatial context is invaluable for effective forest management practices, such as harvest planning, conservation efforts, and wildfire risk assessment.
Automated data pipelines and interoperability between AI-based counting trees systems and existing forest management software are crucial for ensuring seamless integration. This may require the development of standardized data formats, application programming interfaces (APIs), and data exchange protocols in counting trees to facilitate the flow of information between different systems.
Advantages of AI Tree Counting
Automated AI analysis offers numerous potential advantages over manual interpretation for counting trees, including:
Much faster processing of huge volumes of overhead imagery
Lower costs by reducing or eliminating manual labor
Higher consistency without subjective human bias
Scalability across regions and repeat sampling over time
Increased accuracy via data fusion and integration
By improving counting efficiency, AI can support higher-resolution monitoring to inform carbon accounting, biodiversity assessment, and sustainable forest management. However, some key challenges remain.
Industry and Market Insights on Tree Counting Software
Tree counting software leverages advanced technologies like artificial intelligence, machine learning, and remote sensing to automate the traditionally labor-intensive process of counting trees. These software solutions analyze aerial imagery, LiDAR data, and other relevant datasets to generate precise tree counts, enabling forest managers to make informed decisions
The market for tree counting software is characterized by a diverse range of products and services. From standalone software applications to cloud-based platforms, the industry offers solutions tailored to different user needs and budget constraints. As the technology continues to evolve, we can expect to see even more sophisticated tree counting software with enhanced capabilities, such as real-time data processing, integration with other forestry tools, and improved accuracy.
To invest on the growing demand for tree counting software, companies must focus on developing user-friendly interfaces, robust data management features, and reliable customer support. Additionally, staying abreast of the latest advancements in remote sensing and machine learning is crucial for maintaining a competitive edge in this dynamic market.
The potential applications of tree counting software extend beyond traditional forestry. For instance, the software can be used in agriculture, urban planning, and AI and natural disaster prediction. As the technology continues to evolve, we can expect to see even more innovative applications emerge.
Limitations and Future Work for Counting Trees
While AI clearly presents new opportunities for counting trees, several limitations and areas for improvement remain. Many recent studies have focused on simplistic plantation settings. Performance can degrade in complex natural forests with high biodiversity, dense understory vegetation, or uneven age structure. Interpretability continues to be a challenge for deep neural networks. Diagnosing and correcting model errors is difficult.
Data availability and quality remain primary constraints. Well-annotated optical, LiDAR, and field data across diverse biomes is essential for training robust models. However, accumulating sufficient reference data through manual image labeling and field sampling requires major time and resource investments. Data fusion techniques help maximize information yield from limited samples. However, airflow distortion in LiDAR and sun-sensor geometry effects for optical data can introduce artifacts that complicate analysis.
Future priorities include aggregating larger diverse training datasets, testing transferability across sites and conditions, and exploring optimal sensor and data fusion strategies. Ensemble approaches that integrate outputs from multiple models and data sources could improve consistency and accuracy. Canopy penetrating LiDAR may have advantages for occlusion prone regions. Fully automated workflows from data pre-processing through analysis will be needed for operational implementation. Continued benchmarking on field data will be critical for quantifying uncertainties.
How to Count Trees in a Forest | The Economic Impact of Counting Trees Using AI
Traditionally, "how to count trees in a forest" has been a major hurdle in forestry management. It's a laborious and expensive task that requires physical surveys and manual counting trees in a forest. However, Artificial Intelligence is changing the game, revolutionizing how to count trees in a forest and impacting the economic landscape of forestry.
Firstly, AI automates a significant portion of the "how to count trees in a forest" problem. Instead of relying on manual surveys, AI algorithms analyze aerial imagery or LiDAR data. This allows for efficient and cost-effective counting trees in a forest across vast areas. Imagine the economic benefits of skipping tedious manual counting in a forest and letting AI handle it!
Secondly, AI enhances the accuracy of how to count trees in a forest. Traditional methods are prone to human error, but AI algorithms can achieve near-perfect precision. This leads to more reliable data for economic decisions. Precise forest counts ensure accurate timber volume estimates, a critical factor for the forestry industry. Knowing the exact number of trees in a forest allows for confident calculations and economic planning related to timber harvesting.
Finally, AI used in agriculture unlocks new economic opportunities through improved methods for the main question “how to count trees in a forest”. With faster and more accurate forest counts, companies can explore sustainable harvesting practices and participate in carbon offset markets. By knowing exactly how to count trees in a forest and how many trees are present, businesses can confidently measure and manage their carbon footprint, creating a new economic avenue.
In conclusion, AI-powered counting trees in a forest is transforming the forestry industry. By streamlining processes, improving accuracy, and unlocking new economic avenues, AI is poised to create a more efficient and sustainable future for our forests.
Harnessing GIS for Tree Counting Methods in Forestry
In the realm of forestry, Geographic Information System (GIS) applications have revolutionized the way we approach tree counting methods. The integration of GIS technology not only streamlines the process but also enhances the accuracy and efficiency of tree counting initiatives.
GIS allows foresters and environmentalists to employ spatial analysis tools, creating a robust framework for tree counting methods. One of the key advantages of GIS in forestry lies in its ability to combine various data sources, such as satellite imagery, LiDAR, and field surveys, to generate comprehensive and accurate tree inventories.
The first step in employing GIS for tree counting involves the acquisition of high-resolution satellite imagery. These images serve as a foundational layer, aiding in the identification and delineation of tree canopies. Through sophisticated image processing techniques, GIS facilitates the automation of tree counting methods, reducing manual efforts and enhancing precision.
Moreover, GIS extends its utility by incorporating LiDAR data into the analysis. LiDAR provides detailed three-dimensional information about the forest canopy structure, allowing for a more nuanced understanding of tree distribution. This integration significantly improves the reliability of tree counting methods, especially in areas with complex topography or dense vegetation.
GIS-driven tree counting methods offer a dynamic approach to forestry management. The technology enables real-time monitoring and updates, allowing for adaptive strategies based on evolving environmental conditions. This continuous monitoring not only aids in accurate tree counting but also provides valuable insights for sustainable forest management practices.
Community engagement is another aspect where GIS applications shine in the context of tree counting. By incorporating citizen science initiatives and crowd-sourced data into GIS platforms, local communities become integral contributors to the tree-counting process. This collaborative approach fosters a sense of shared responsibility for environmental stewardship.
Read Also : Application of Image Processing in Agriculture
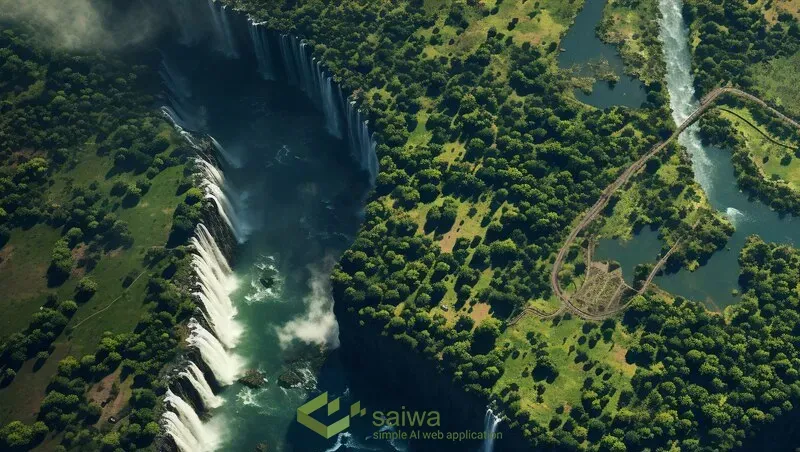
GIS applications play a pivotal role in advancing tree counting methods within the forestry domain. The integration of GIS technology enhances accuracy, efficiency, and community involvement, making it a cornerstone for modern forestry practices. As we delve deeper into the era of smart environmental management, the repeated emphasis on "tree counting methods" underscores its central importance in maintaining and preserving our invaluable forest ecosystems.
Conclusion
Advanced AI techniques show tremendous promise to reinvent counting trees but are not yet ready for full operational deployment. Priorities for unlocking their potential include expanding labeled training data resources across diverse forest ecosystems, improving model transferability, and sustained calibration and validation using field measurements. Combined with new satellite systems and drone data acquisition, AI-based counting trees could transform twenty-first-century forest monitoring. Realizing this future requires cross-disciplinary collaboration between remote sensing experts, ecologists, and AI researchers to develop robust integrated solutions.