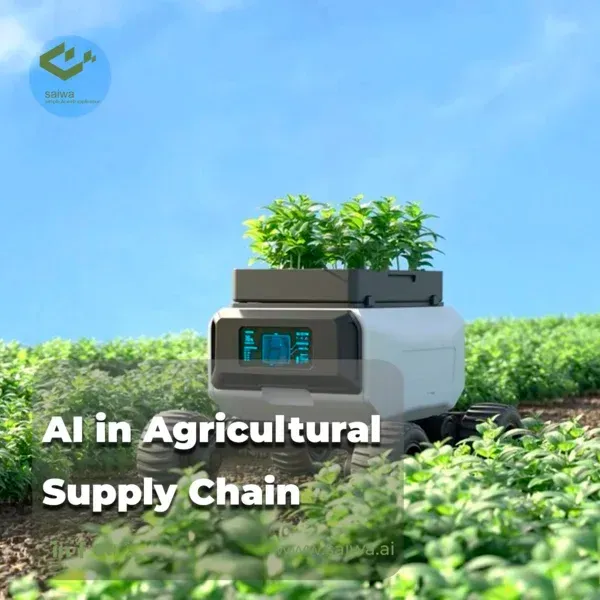
Over the coming decades, the global agricultural industry faces immense challenges to cost-effectively boost production volumes to nourish swelling population numbers in the face of dwindling arable land availability and climate change pressures. Agricultural producers must find ways to grow more resilient crop varieties while majorly enhancing yields within tightly constrained water resource budgets. Downstream along intricate farm-to-table supply chains, players contend with substantial food spoilage losses while consumer expectations around sustainability sourcing and food safety vigilance intensify from retailers down to end shoppers selecting grocery items.
Advanced AI capabilities stand ready to transform decision automation and optimization across the agricultural ecosystem from initial crop selection and cultivating decisions made on the farm through intermediate storage, processing, and distribution when delivering nutrition to neighborhood store shelves. When deployed skillfully, machine learning algorithms and AI in agricultural supply chain can guide more informed planting and harvesting protocols, detect risk factors compromising crop health earlier, route produce shipments more astutely, and ensure compliance with expanding regulatory demands around safety and transparency.
Crop Planning and Farming Operations
Long before harvest trucks get loaded for the very first time each season, AI in agricultural supply chain plays a major role in informing delicate decisions around precisely which high-value crops represent the ideal candidates for working farmland areas under management based on hyperlocal soil composition, historic regional precipitation patterns, and weather forecasts for the coming crop cycle. Agricultural data science specialists input soil nutrition panels, past seasonal rainfall metrics, and climate forecasts into crop planning models to run iterative simulations calculating projected yield potentials measured against different candidate seed varieties and plant spacing layouts. AI determines optimal acreage allocation per crop to satisfy demand forecasts and maximize farm income within sustainable boundaries.
Precision Farming with Computer Vision
Once crops get planted, computer vision integrated into autonomous tractor rigs takes over to cultivate and treat emerging crops with precision. As plants mature, aerial drones and on-the-ground robots equipped with spectral imaging sensors constantly survey crop health characteristics, alerting growers to any signs of deterioration or disease identified early before reaching devastating levels. The continual monitoring protects yields as algorithms classify crop issues and prescribe targeted corrective measures for technicians to review and approve treatment action.
Read Also: An Over View Applications of Computer Vision in Agriculture
Intelligent Harvesting and Yield Optimization
Additional sensors track indicators of crop ripeness, at which point AI in agricultural supply chain guides autonomous picking rigs on exact harvest timing and collection routes to gather produce at peak freshness.
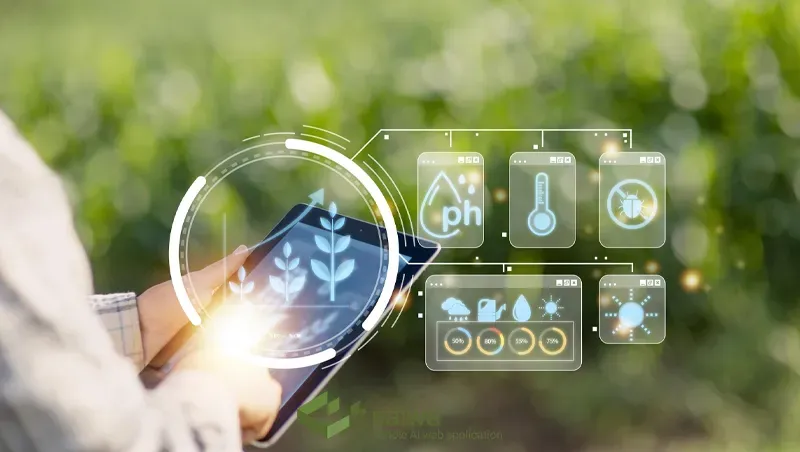
Predictive Analytics for Crop Yield and Quality
Growers and subsequent supply chain participants downstream stand to gain immense advantages from machine learning algorithms converting historical agricultural datasets paired with incoming real-time IoT sensor telemetry into actionable intelligence forecasting ahead:
Yield Volume Prediction for Crops
Agri-tech companies developed advanced Agri-tech sensing and computer vision for crops as they grow giving mid-season perspectives into size, density, and potential harvesting estimates for crop fields weeks before picking allowing operations executives to set early expectations around quantities of raw materials anticipated for procurement budgeting and storage capacity planning ahead of time.
Crop Quality Grading Guidance
Predictive analytics around eventual crop quality grades and classifications weeks out based on indicators monitored through the growth lifecycle empowers preemptive decisions allocating downstream sorting, processing, and storage infrastructure to avoid grade differentiation value leakage or penalties assessed by buyers.
Crop Variety Identification
When raw agricultural goods lack clear indicators of their precise cultivar lineage and properties, AI powered by spectroscopy and sample property analysis delivers probabilities around likely crop subtype identification assisting decisions on ideal pairings for recipes, processing methodology selections, and even projected finished output compositions.
Read Also: Predictive Analytics in Agriculture | Fields of Insight
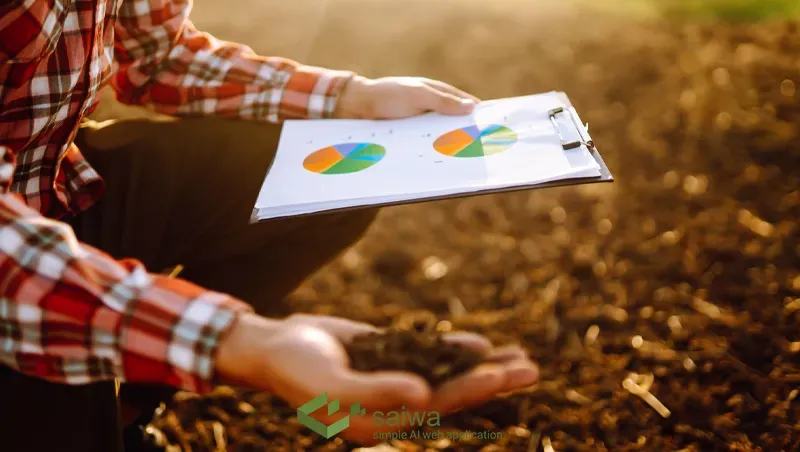
Supply Chain Transportation and Storage
Post-harvest, maintaining food safety and quality involves careful handling across processing, storage, and logistics links before reaching retailers. Harsh vibrations, big swings in humidity, and temperature exposure quickly accelerate food decay if left unmitigated. Telematics sensors attached to fresh goods monitor product environments throughout transit, triggering alerts when adverse conditions threaten shelf life. Connected analytics detect patterns correlated with higher levels of waste to dial in optimized transport protocols.
Weather-Informed Routine
Routing systems constantly ingest weather data to choose routes less prone to delays getting perishables to their next destinations promptly. Predictive model analysis on inbound supply volumes also informs processing plant teams to align downstream packaging, storage, and staffing needs avoiding bottleneck pileups.
Ensuring Quality in Storage and Warehousing
During temporary warehousing periods, continuous air quality readings allow facilities teams to proactively tune climate control setpoints to best preserve harvested materials correctly. Advanced scanning technology further ensures storage containers meet stringent cleanliness requirements before loading to prevent contamination. As shipments get dispatched to distributors, sensors enable remote troubleshooting of mechanical issues with transport refrigeration units to minimize potential temperature fluctuations and food safety risks if equipment were to malfunction unattended.
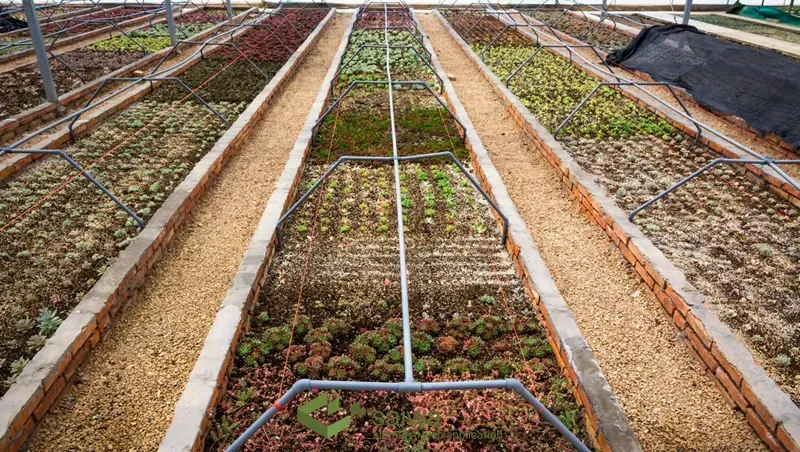
Supply Chain Optimization with AI
Integrating AI in agricultural supply chain more pervasively into planning and execution enables transformative opportunities to economize operations from field outcomes downstream through final delivery:
Prescriptive Replenishment Optimization
AI-powered demand forecasting refines supply planning, processing & packaging schedules, and finished goods inventory buffers kept at distribution centers to help ensure product availability meets sales velocity changes regionally while maximizing inventory turns and mitigating waste buildups.
Dynamic Distribution Network Reconfiguration
As customer density, seasonal purchase variability, channel shifts, and outlet expansions occur geospatially, AI mapping based on SKU-level projected demand volumes aids strategic optimization of distribution center footprint, selection of 3PL carriers by lanes, and fine-tuned last mile delivery tactics fulfilling orders profitably.
Predictive Maintenance Modeling
Drawing correlations between telemetry sensor histories monitoring equipment vibration, temperatures, and hours of utilization with eventual failure occurrences allows AI engines to recommend maintenance interventions ahead of time before disruptions instead of reactive approaches once issues emerge and lead to lost productivity around the processing line or during transportation.
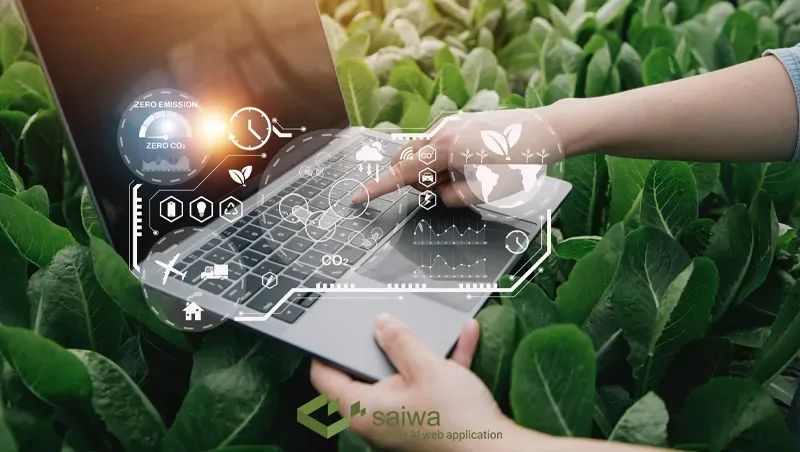
AI in Agri Supply Chain Management Fights Food Fraud
Food fraud, the intentional misrepresentation of food products, is a growing concern threatening consumer safety and fair competition. Traditional methods for ensuring food authenticity and traceability often rely on paper trails and manual inspections, leaving vulnerabilities for exploitation. However, AI in Agri supply chain management offers a powerful weapon in the fight against food fraud.
AI-powered solutions within the Agri supply chain management system can track food products from farm to fork with unparalleled precision. Image recognition technology can analyze photographs taken at various stages of production, identifying inconsistencies in product appearance that might signal fraud. Meanwhile, AI can analyze vast datasets encompassing sensor readings, logistics records, and even satellite imagery of crops. This allows for the creation of a detailed digital footprint for each product, highlighting any deviations from the established path.
The benefits of this increased transparency extend across the food system. Consumers gain much-needed confidence in the authenticity and safety of the food they purchase. Farmers and legitimate businesses are protected from unfair competition by fraudulent products. Regulatory bodies can leverage AI in Agri supply chain management to identify and swiftly address food fraud attempts. By harnessing the power of AI in Agri supply chain management, the food industry can move towards a future where transparency reigns supreme, fostering trust and ensuring the safety of our food supply.
AI Use Cases for Agricultural Supply Chains
When exploring practical AI solution entry points across agribusiness verticals, a few high-impact application areas immediately stand out as providing substantial value potential:
Livestock Health Monitoring
Ingestible sensors and computer vision-enabled health tracking wearables onboard cattle can continuously assess indicators of sickness, lameness, or reactions to dietary changes in near real-time without intensive manual inspections required. Herd management analytics reveals insights leading to earlier interventions maximizing herd health outcomes.
Read Also: A Guide to AI in Livestock Monitoring |Smart Farms
Soil Composition Analytics
Applying hyperspectral imagery from satellite feeds and proximal soil sensors creates rich mapped visualizations indicating locations with nutrient deficits and helps determine optimal remedies of supplements required using precision release methods preventing overapplication.
Weather Forecasting Analytics
Ingesting feeds from adjacent weather stations, thermometers, hygrometers, and leaf wetness sensors distributed across acreage assists AI in agricultural supply chain engines to model location-specific microclimate forecasts over the weeks ahead aiding growers to align planting, irrigation, fertilization, and harvesting actions with greater certainty.
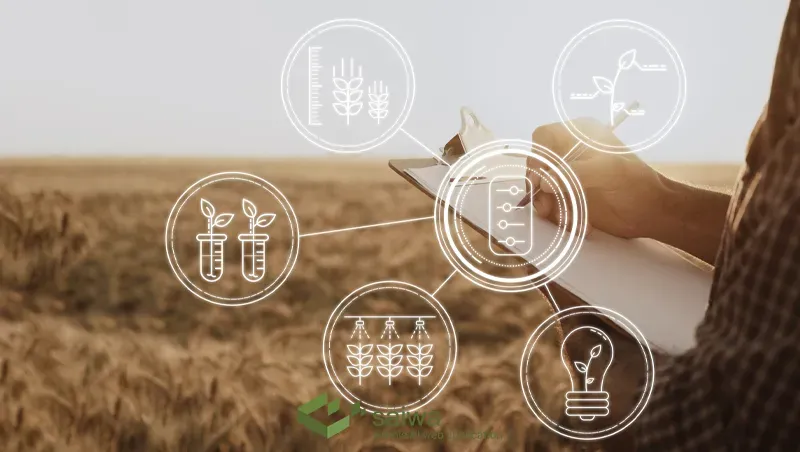
Conclusion
The integration of artificial intelligence capabilities across major links within agricultural ecosystems enables transformative advancements in efficiency and responsible resource utilization critical to feeding expanding populations sustainably while addressing brand safety vulnerabilities. AI in agricultural supply chain unlocks superior visibility into field conditions, crop development, and downstream food integrity as goods traverse from farms to grocery aisles. When core AI applications come together in harmony driving smarter decisions unified by data, rather than disjointed siloed optimization tactics, the result can satisfy consumer expectations for quality, safety, and affordability simultaneously across integrated agricultural networks.