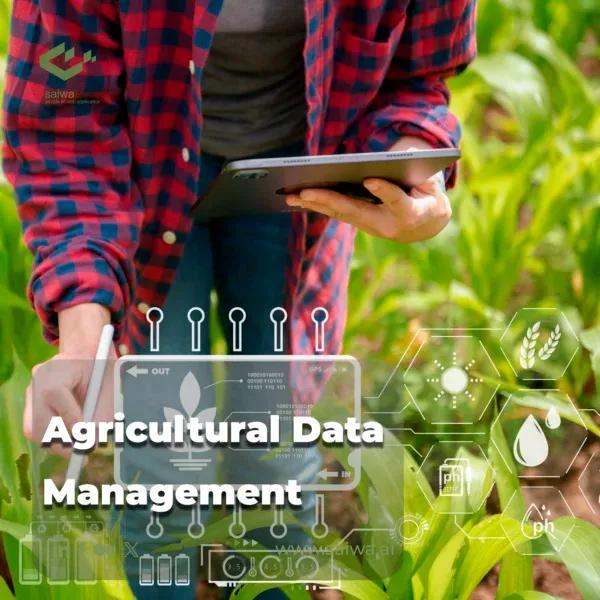
A Guide to Agricultural Data Management| Digital Fields
Modern agri-tech advancements in precision sensors, autonomous farm equipment, aerial imaging, and internet-connected infrastructures unlock immense potential for agricultural enterprises to harness data as a strategic asset guiding enhanced productivity, sustainability, and resource efficiency. As tractors, harvesting, and field hardware grow increasingly instrumented with interconnectivity for optimizing routines season after season vast datasets get generated containing hidden insights if skillfully collected, structured, and analyzed.
Yet without proactive data management foundations established early on, the promise of data-driven AI in agriculture quickly becomes overwhelmed by volumes of disorganized records offering minimal actual enterprise visibility. By implementing structured data harvesting pipelines coordinated with reliable storage solutions and analytics platforms, farm organizations can extract much greater value from precision agriculture programs over the long term.
This overview covers practical approaches to agricultural data management spanning intelligent data collection mechanisms, on-premise and cloud storage strategies, and analytics tools for deriving operational intelligence - all of which connect into a cohesive farm data utilization framework.
Importance of Agricultural Data Management
Without proper data harvesting, storage, and governance practices established early on, gaps occur losing pivotal context that could improve future decision analytics.
Uncovering Correlative Insights
Experts can pinpoint influential correlations between isolated environmental factors and yields through statistical analysis of diverse aggregated data sources combined holistically.
Planning Operations Judiciously
Complete data histories fuel precise forecast modeling capabilities that allow farmers to determine ideal crop selection, planting locations, harvest windows, and cultivation amendments custom-tailored to their unique land characteristics season over season.
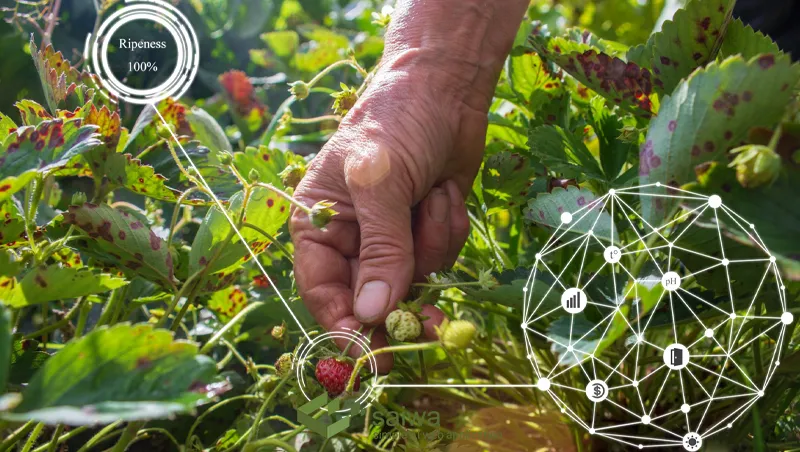
Types of Agricultural Data
Here are the main types of agricultural data management.
Farm Equipment Telemetry
Modern tractors, harvesters, and implements generate immense volumes of live usage statistics including engine load metrics, fluid levels, throttle application, hydraulic pressure, rotor speeds, and more. Location identifiers assign recordings to field zones.
Crop Analysis Feeds
Multispectral aerial imagery, drone footage, and scanner readings on crop health, maturity states, moisture stress, and growth phases build data pillars for predictive modeling.
Animal Biometrics
Ingestible health monitoring sensors, wearables on cattle, video tracking in hen houses, and milk output meters create information streams around livestock conditions optimizing welfare.
Read Also: A Guide to AI in Livestock Monitoring |Smart Farms
Weather Station Sensor Grids
Arrays of weather sensors distributed across acreages measuring hyperlocal variations in temperature, precipitation, dew point, soil temperature, and evapotranspiration rates at 5–15-minute sampling frequencies inform irrigation, fertilization, and harvest timing decisively.
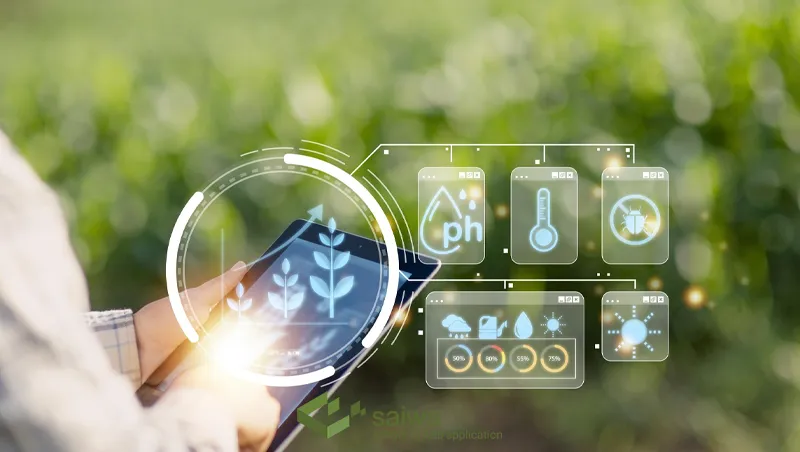
Data Collection and Instrumentation
The starting point for leveraging agricultural data management begins with instrumenting equipment, vehicles, livestock, and environmental sensors measuring details occurring across farm operations. Data harvesting requires more than just capturing isolated siloed metrics in bulk but thoughtfully tying recordings to location and timing context.
As tractors pass through fields performing planting, spraying, and harvesting routines for example, IoT modules can encode geo-coordinates documenting exactly where machinery operates matched to runtime timestamps plus tied operational statistics like fluid levels and diesel consumption to link usage factors back to field zones.
Meanwhile, dense sensor grid layouts scattered across acreage measuring hyperlocal weather conditions every few minutes fueled by on-site solar power can feed into data pools providing microclimate insights impossible from relying on isolated broader regional weather reports alone.
Appending additional sensors onto livestock animals to assess movements, feed intake, and biometrics during grazing, transportation, and sheltering provides invaluable health monitoring indicators tied back to groups using edge data processing.
Structured data gathering requires intentional instrumentation using appropriate fieldbus protocols and APIs capable of handling agriculture working conditions and interfaces rather than just generic IT equipment. Ruggedized IoT devices survive prolonged outdoor placement across seasons while integrating adequately with existing equipment.
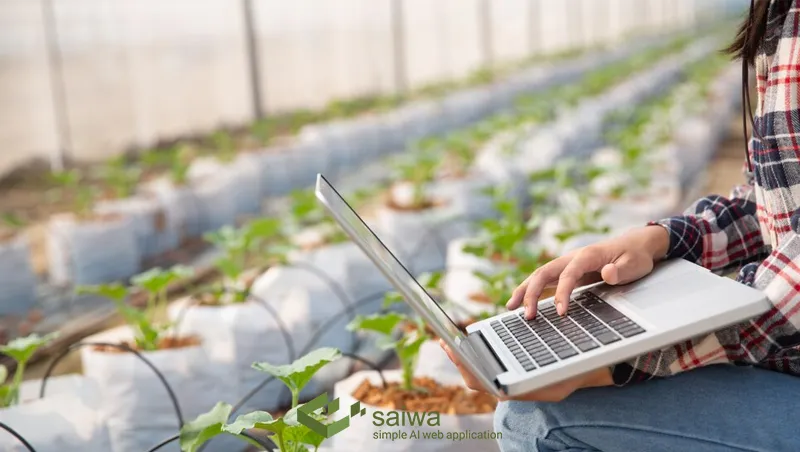
Data Storage Infrastructure
The mass-scale machine-generated recording volumes amassing daily from scattered agricultural data sources require planning sufficient backend infrastructure for securely aggregating and storing the continual flows for processing. A hybrid approach blending on-premise solutions with public cloud platforms balances affordability, scalability, and analytics readiness:
Locally managed data warehouses and servers offer inexpensive starting capacity for smaller operators to retain recent seasons of aggregated data if sized adequately. However backup power protection is essential to maintain uptime through grid outages to avoid gaps in data flows which would impede complete harvesting records.
As analytic needs develop, expanding into public cloud infrastructure delivers vastly greater storage capacity for developing more complete multi-year agricultural data lakes at reduced incremental costs. Cloud analytics engines input cleansed datasets while applying machine learning techniques against historical benchmarks pursuing advanced modeling. AWS, Google Cloud, and Azure provide services tailored for ag data workloads.
Based on growth expectations down the road, hybrid architectures maintain selective core data like sensor readings on local storage infrastructures while funneling processed derivative datasets into cloud repositories cost-effectively. This facilitates analyzing manageable recent data batches on-premise while offloading cold long-term archives externally.
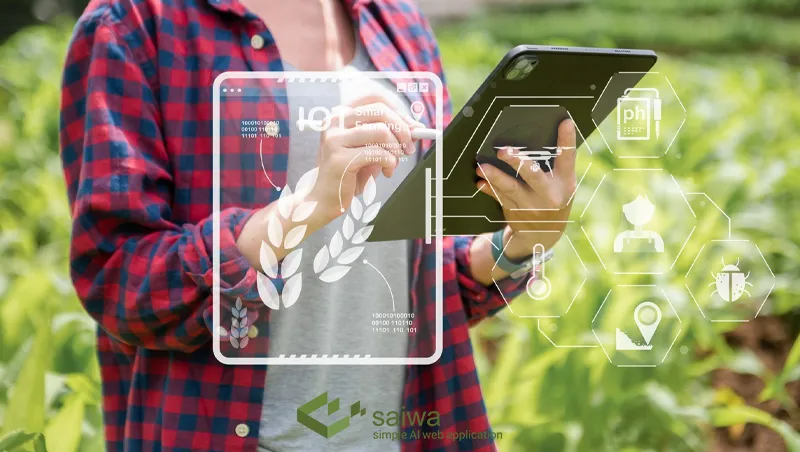
Case Studies
Examining successful case studies provides insights into the real-world impact of agricultural data management. Farms that have embraced data-driven practices showcase tangible benefits, from increased productivity to improved resource efficiency. These examples serve as inspiration for others looking to adopt similar approaches.
Predictive Crop Planning with Satellite Data
By ingesting satellite imagery data documenting multiple prior growing years showing pivot irrigation patterns and subsequent yield results differentiated by crop varieties, AI models can optimally determine the highest value cultivation planting recommendations for each zone maximizing producer profits.
Earlier Harvesting from Maturity Monitoring
Ingesting regular aerial NDVI crop scans tracking maturation phases across mid-season growth followed by geo-tagged early harvester route completion rates layered over yield accuracy logging enables tighter protocols for dispatching autonomous picking fleet routes daily based on crops reaching maximal ripeness first.
Variable Rate Amendment Adjustments
After clustering soil composition scans, drainage profiles, and yield results logged geo-spatially over years, prescription map layers get generated indicating optimal variable application rates for irrigation, fertilizers, pesticides, and liming tuned at a sub-acre level personalized to each zone’s potential.
Conclusion
Agriculture organizations risk significant missed efficiency opportunities and competitive advantage by not actively governing field data early on. Intelligently instrumenting equipment while implementing structured data platforms, both on-premise and cloud-based, unlocks game-changing analytics around crop management, livestock health improvements, and accurate yield forecasting over seasons. As data analytics in agricultural data management guides more judicious operational changes, farmers gain capabilities to enhance margins through earlier issue intervention and resources allocated based on advanced predictive science.