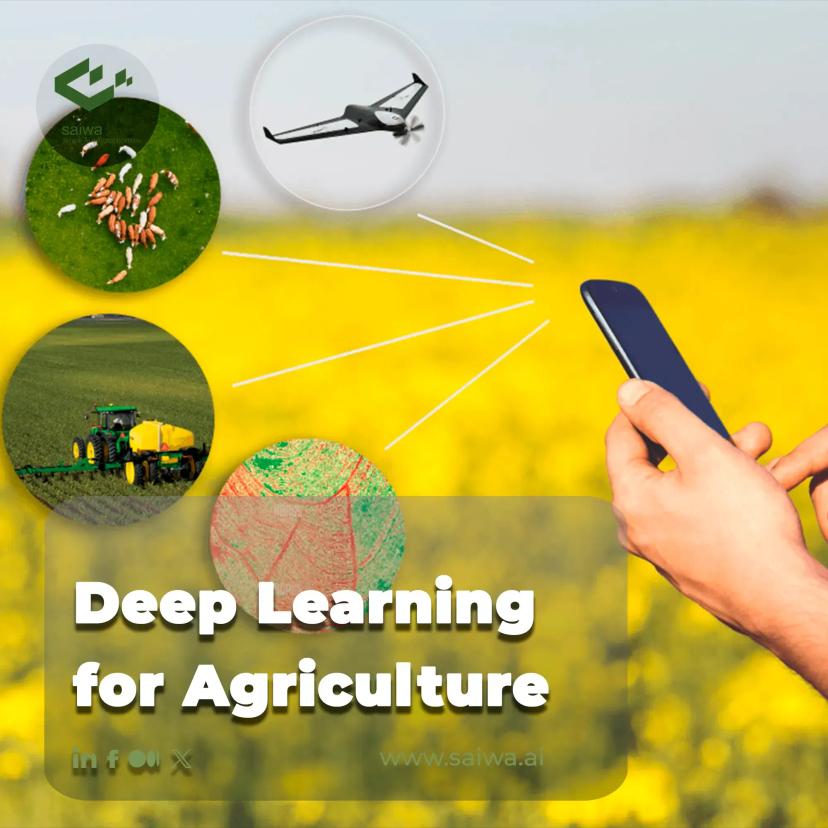
Deep Learning for Agriculture | Data-Driven Decisions and Increased Efficiency
The foundation of human civilization, agriculture, is facing significant challenges as the global population is projected to reach nearly 10 billion by 2050. This surge in population necessitates the development of new technologies to enhance agricultural productivity, efficiency, and sustainability. Technological advancements, particularly in the field of deep learning, which is a subfield of artificial intelligence, offer promising solutions. The analysis of extensive agricultural data, including satellite imagery, sensor readings, and weather information, enables the extraction of valuable insights and the automation of essential tasks, thereby transforming the agricultural sector.
Saiwa, an AI company, employs the power of deep learning to provide cutting-edge solutions in image processing, detection, and authentication services. Our significant product, Sairon, exemplifies this innovation with AI-powered drone applications that automate high-resolution aerial image processing. Sairon’s deep learning models analyze drone imagery for habitat monitoring, crop health assessment, and environmental conservation, generating precise and efficient reports. The integration of deep learning by Saiwa enhances the accuracy of crop disease detection, monitoring soil health, and optimizing resource use. By delivering actionable insights, Saiwa empowers farmers to improve yields and adopt sustainable farming practices. Our robust image processing and detection services address the complex challenges of modern agriculture, ensuring efficient and effective resource management.
Overview of deep learning and its potential applications in agriculture
Deep learning is a type of machine learning characterized by the use of artificial neural networks with multiple hidden layers. These neural networks are inspired by the structure and function of the human brain, allowing them to learn complex patterns and relationships from data. Deep learning models can be trained on large datasets to perform various tasks, such as image recognition, object detection, time series forecasting, and natural language processing.
In agriculture, deep learning holds immense potential for applications across the entire crop production cycle, from planting to harvest and beyond. Here are some potential areas of application:
Crop monitoring and yield prediction: Deep learning can analyze satellite imagery, drone data, and sensor readings from fields to monitor crop health, predict yields, and identify areas requiring attention.
Plant disease and pest detection: Deep learning models can be trained to automatically detect plant diseases and pests using images captured from drones or ground-based sensors. This enables early intervention and minimizes crop losses.
Crop breeding and genetics: Deep learning can be used to analyze plant phenotypes (observable characteristics) and genomic data to accelerate breeding programs and develop new crop varieties with desired traits like disease resistance or improved yield.
Precision agriculture and farm management: Deep learning can help farmers optimize resource use by analyzing soil data, weather patterns, and historical yield data. This allows for variable-rate application of fertilizers, pesticides, and irrigation water based on specific field conditions.
Supply chain and traceability: Deep learning can improve the efficiency and transparency of agricultural supply chains by tracking the movement of food products from farm to fork. This helps ensure food safety, prevent contamination, and identify potential fraud.
Sustainable and regenerative agriculture: Deep learning can be used to assess the environmental impact of agricultural practices and develop strategies for sustainable farming.
Read Also: Practical Application & Future of AI in Agriculture
Why machine learning in agriculture is important
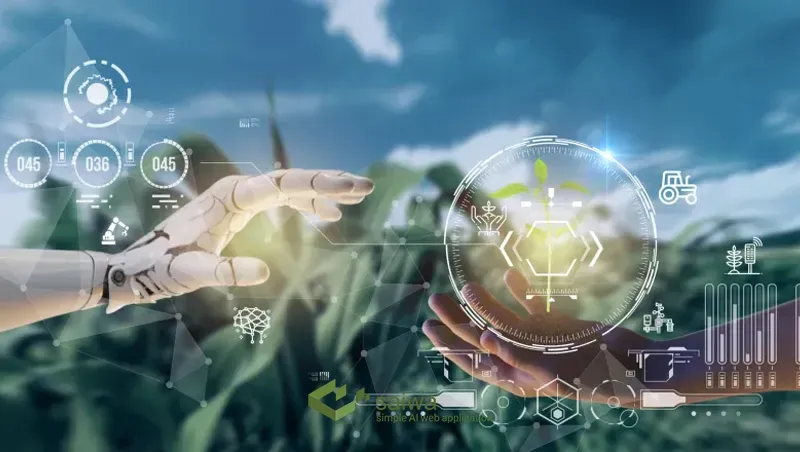
Traditional agricultural practices often rely on manual labor and human intuition. However, deep learning offers several advantages:
Data-driven decision making: Deep learning algorithms can analyze vast amounts of data to identify patterns and trends that might be missed by humans. This allows farmers to make data-driven decisions that optimize yields and resource use.
Automation of repetitive tasks: Deep learning can automate tasks such as disease detection, weed detection, and yield estimation, freeing up farmers' time to focus on other critical aspects of their operations.
Improved accuracy and efficiency: Deep learning models can achieve high levels of accuracy in tasks like crop classification, pest detection, and yield prediction. This leads to improved efficiency and reduced waste in agricultural practices.
Scalability and adaptability: Deep learning models can be easily scaled to analyze data from large farms or entire regions. Additionally, they can be adapted to different crops, environmental conditions, and farming practices.
Read More: The Impact of Machine Learning in Agriculture
Crop Monitoring and Yield Prediction
Remote sensing and satellite imagery analysis
Remote sensing technologies like satellites and aerial photography provide valuable data for monitoring crop health and predicting yields. Deep learning can analyze this data for various purposes:
Crop classification and mapping: Deep learning models can classify different types of crops (e.g., wheat, corn, soybeans) within satellite images. This information is crucial for creating accurate crop maps and tracking changes in land use over time.
Vegetation indices and crop health monitoring: Vegetation indices derived from satellite imagery can be used to assess crop health and identify potential problems like nutrient deficiencies or water stress. Deep learning algorithms can analyze these indices to generate real-time maps of crop health across large areas.
Yield forecasting and estimation: By analyzing historical yield data, weather patterns, and satellite imagery, deep learning models can forecast crop yields before harvest. This information helps farmers make informed decisions about resource allocation, pricing strategies, and risk management.
Unmanned Aerial Vehicle (UAV) and drone-based monitoring
UAVs (drones) equipped with high-resolution cameras and multispectral sensors are increasingly used for crop monitoring. Deep learning can be applied to analyze data collected UAVs (drones) equipped with high-resolution cameras and multispectral sensors are increasingly used for crop monitoring. Deep learning can be applied to analyze data collected by drones for various purposes:
Hyperspectral and multispectral imaging: Drones can capture data beyond the visible spectrum, providing valuable information about plant health and stress. Deep learning models can analyze these multispectral images to detect subtle changes in plant physiology that might indicate disease, nutrient deficiencies, or water stress.
Plant phenotyping and growth monitoring: Deep learning can be used to analyze drone imagery for automated plant phenotyping, which involves measuring various plant traits like leaf area, canopy cover, and plant height. This data provides insights into plant growth patterns and allows for early identification of potential problems.
Precision agriculture and variable rate application: By analyzing high-resolution drone imagery and combining it with other data sources like soil maps, deep learning can help create detailed field maps for variable rate application (VRA) of fertilizers, pesticides, and irrigation water. VRA optimizes resource use by applying inputs only where they are needed, reducing waste and environmental impact.
Read Also: AI-Based Drone Operation| AI in Drones Use Cases
Plant Disease and Pest Detection
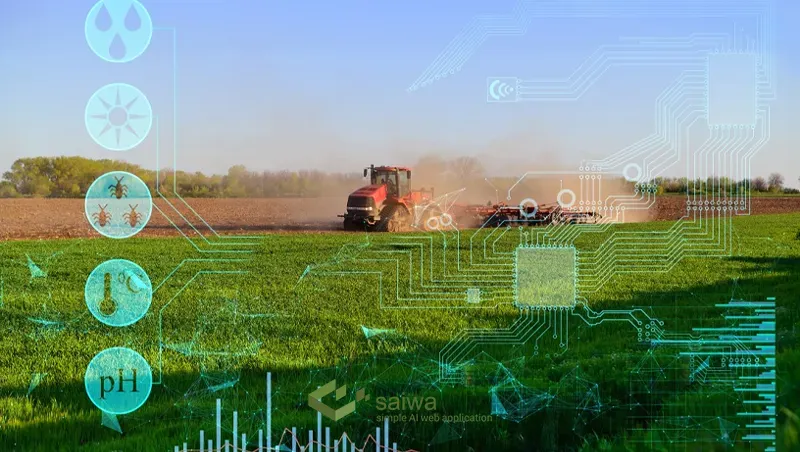
Image-based plant disease diagnosis
Early detection of plant diseases is crucial for minimizing crop losses. Deep learning can be a powerful tool for automated disease diagnosis using images captured from various sources:
Leaf and fruit disease detection: Deep learning models trained on large datasets of labeled images can identify various plant diseases based on visual symptoms on leaves, fruits, and stems. This allows for early intervention and targeted application of fungicides or other control measures.
Early detection and monitoring of disease spread: Deep learning can analyze time-series images captured by drones or ground-based sensors to track the spread of plant diseases across a field. This allows farmers to take timely action to contain outbreaks and prevent further losses.
Transfer learning and domain adaptation techniques: Transfer learning allows deep learning models trained on a large dataset of general-purpose images to be adapted for specific tasks like disease detection in a new dataset with limited labeled images for agricultural diseases. This is particularly useful for resource-constrained settings where acquiring large amounts of labeled agricultural data can be challenging.
Automated pest detection and monitoring
Pests like insects and weeds can significantly reduce crop yields. Deep learning can be used for automated pest detection and monitoring:
Insect and weed identification: Deep learning models can be trained to identify different types of insects and weeds based on images captured by drones or ground-based cameras. This allows for targeted pest control measures and reduces unnecessary use of broad-spectrum insecticides and herbicides.
Pest behavior analysis and population dynamics: By analyzing time-series images and video data, deep learning can help track pest movement and population dynamics. This information can be used to develop more effective pest management strategies.
Integrated pest management strategies: Deep learning can be integrated with other technologies like weather monitoring and pheromone traps to create comprehensive integrated pest management (IPM) systems. IPM strategies aim to control pests by combining various methods, minimizing reliance on chemical pesticides, and promoting sustainable agriculture.
Crop Breeding and Genetics
High-throughput plant phenotyping
Traditional plant breeding relies on manual observation and selection of desirable traits. Deep learning can accelerate breeding programs through:
Image-based trait analysis: Deep learning models can analyze images captured from drones or ground-based sensors to automatically measure various plant traits like leaf area, plant height, and biomass. This high-throughput phenotyping allows breeders to rapidly evaluate large numbers of plants and identify those with desired qualities.
Automated phenotyping platforms and facilities: Automated phenotyping platforms equipped with high-resolution cameras, controlled lighting conditions, and conveyor belts can be used to image large numbers of plants in a standardized manner. Deep learning algorithms can then analyze these images to extract relevant phenotypic data.
Genomic selection and breeding value prediction: Deep learning can be used to analyze both phenotypic and genotypic data (plant DNA information) to predict the breeding value of individual plants. This allows breeders to select plants with desirable traits more efficiently and accelerate the development of new crop varieties.
Genomic Data Analysis and Bioinformatics
The field of genomics is rapidly advancing, providing a wealth of data about plant genes and their functions. Deep learning can be applied to analyze this data for various purposes:
Sequence analysis and variant calling
Deep learning can be used to analyze DNA sequencing data to identify genetic variations (mutations) associated with specific traits. This information can be used for marker-assisted selection in breeding programs and to understand the genetic basis of complex traits like disease resistance or drought tolerance.
Gene expression profiling and regulatory network inference
Deep learning can analyze gene expression data (which genes are active in different tissues or under different conditions) to identify genes involved in important plant processes. This information can help us understand how genes interact with each other to regulate plant growth, development, and stress responses.
Genomic prediction and genome-wide association studies (GWAS)
Deep learning can be used to analyze large datasets of genotypic and phenotypic data to identify genetic markers associated with complex traits. This approach, known as genome-wide association studies (GWAS), can help breeders develop new crop varieties with desired characteristics more efficiently.
Precision Agriculture and Farm Management
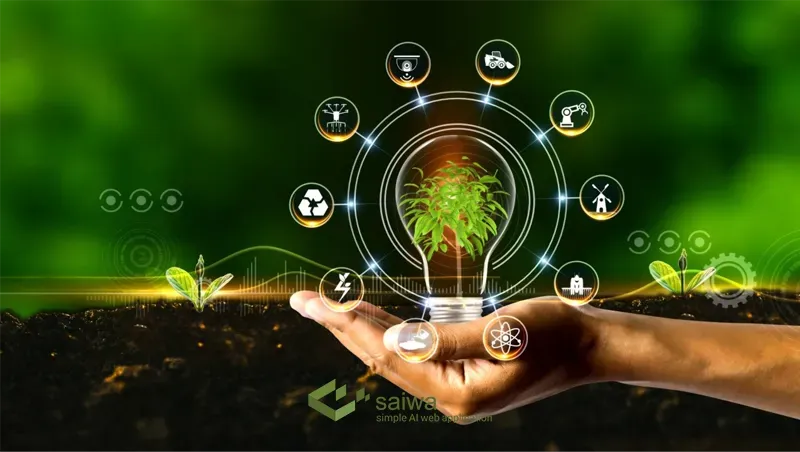
Soil and environmental data analysis
Soil quality and environmental conditions play a crucial role in crop growth and yield. Deep learning can be used to analyze various types of data for precision agriculture:
Soil property mapping and nutrient management: Deep learning models can analyze data from soil sensors and satellite imagery to create detailed maps of soil properties like nutrient content, organic matter, and pH. This information allows farmers to apply fertilizers and other amendments more precisely based on the specific needs of different areas within a field.
Weather and climate data analysis: Deep learning can analyze historical weather data and climate forecasts to predict future weather patterns and potential risks like droughts, floods, or extreme temperatures. This information helps farmers make informed decisions about planting dates, irrigation practices, and crop selection.
Irrigation scheduling and water resource management: By analyzing soil moisture data, weather forecasts, and crop water requirements, deep learning can help farmers optimize irrigation scheduling to ensure optimal water use efficiency. This is particularly important in regions facing water scarcity.
Read More : An Over View Applications of Computer Vision in Agriculture
Precision farming and decision support systems
Deep learning can be integrated with other technologies to create comprehensive decision support systems for precision agriculture:
Variable rate technology and site-specific management: Variable rate technology (VRT) allows farmers to apply inputs like fertilizers, pesticides, and irrigation water at different rates based on specific field conditions. Deep learning models can analyze various data sources to generate VRT prescriptions that optimize resource use and maximize yields.
Yield mapping and field zoning: Deep learning can be used to analyze data from yield monitors on agricultural machinery to create detailed yield maps. These maps can be used to identify areas of the field with consistently lower yields and investigate potential causes like soil fertility issues or drainage problems. Field zoning allows farmers to manage different areas within a field more effectively.
Farm operations optimization and resource allocation: Deep learning can be used to analyze various farm data sources to optimize farm operations and resource allocation. This might include tasks like scheduling labor, planning machinery use, and managing inventory.
Supply Chain and Traceability
Crop and livestock supply chain management
Deep learning can improve transparency and efficiency throughout the agricultural supply chain:
Logistics and transportation optimization: Deep learning algorithms can analyze historical data and real-time traffic information to optimize the logistics and transportation of agricultural products. This reduces transportation costs, minimizes spoilage, and ensures timely delivery to consumers.
Quality control and food safety monitoring: Deep learning models can be used to analyze images captured at different stages of the supply chain to assess the quality of agricultural products and identify potential contamination. This helps ensure food safety and prevent outbreaks of foodborne illnesses.
Blockchain and distributed ledger technologies for traceability: Blockchain technology can be used to create a secure and transparent record of the movement of agricultural products from farm to fork. Deep learning can be integrated with blockchain to analyze sensor data and automate traceability processes, providing consumers with information about the origin, quality, and safety of their food.
Market analysis and price forecasting
Deep learning can be used to analyze market data and weather patterns to forecast future agricultural commodity prices. This information helps farmers make informed decisions about planting decisions, storage strategies, and marketing their products.
Sustainable and Regenerative Agriculture
Environmental impact assessment and carbon footprint analysis
Deep learning can be used to assess the environmental impact of agricultural practices:
Greenhouse gas emission monitoring and mitigation strategies: Deep learning can analyze data from various sources like soil sensors and satellite and drone imagery to estimate greenhouse gas emissions from agricultural activities. This information can be used to develop mitigation strategies and promote sustainable farming practices.
Soil health and conservation practices: Deep learning models can analyze soil data and satellite imagery to assess soil health and identify areas at risk of degradation. This information can help farmers implement conservation practices like cover cropping, reduced tillage, and improved crop rotations to maintain soil health and fertility.
Ecosystem service valuation and biodiversity monitoring: Deep learning can be used to analyze data from drones and remote sensing platforms to monitor biodiversity and ecosystem services provided by agricultural landscapes. This information is crucial for developing sustainable farming practices that promote biodiversity and ecosystem health.
Circular economy and waste management
Deep learning can contribute to a more circular economy in agriculture by:
Agricultural waste valorization and byproduct utilization: Deep learning can analyze data on agricultural waste streams to identify opportunities for valorization (conversion into valuable products). This might involve using deep learning to optimize composting processes, develop biofuels from agricultural residues, or extract valuable nutrients from waste streams for reuse as fertilizers.
Nutrient recycling and closed-loop systems: Deep learning can be used to model nutrient flows within agricultural systems and identify opportunities for nutrient recycling. This could involve optimizing manure management, developing closed-loop hydroponic systems, or using deep learning to design more efficient irrigation practices that minimize nutrient leaching.
Life cycle assessment and product sustainability labeling: Deep learning can be used to analyze data throughout the agricultural life cycle to assess the environmental impact of different products. This information can be used to develop sustainable farming practices and create life cycle assessment (LCA) models that inform product sustainability labeling initiatives.
Data Management, Privacy, and Ethics
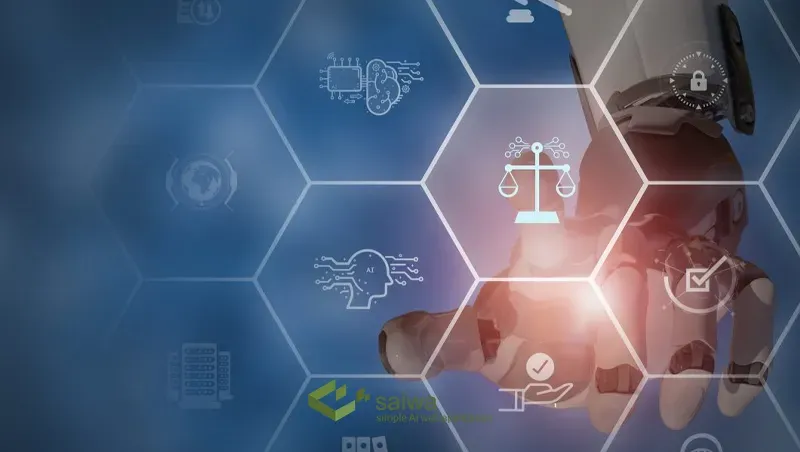
The successful application of deep learning in agriculture relies heavily on data. However, several data management, privacy, and ethical considerations need to be addressed:
Agricultural data governance and privacy concerns: Data collected from farms can be sensitive and may contain information about soil quality, crop yields, and farm management practices. Robust data governance frameworks are needed to ensure data privacy, security, and ownership. Additionally, farmers should have control over how their data is collected, used, and shared.
Privacy-preserving data analytics and federated learning: Techniques like federated learning allow deep learning models to be trained on data distributed across multiple devices or farms without sharing the raw data itself. This can help address privacy concerns and encourage wider participation in data-sharing initiatives.
Data ownership and intellectual property rights: Clear ownership rights for agricultural data need to be established. This includes data collected by sensors, drones, and other farm technologies. Farmers should have ownership of their data and be able to benefit from its use.
Explainable AI and the Path to Transparency
Deep learning for agriculture is a transformative force, but its black-box nature can leave farmers in the dark about how these AI models arrive at decisions. This is where Explainable AI (XAI) steps in, bridging the gap between deep learning's power and human understanding in agriculture.
Here's how XAI integration is revolutionizing deep learning for agriculture:
Trust and Transparency
Unexplained outputs from deep learning models can create a sense of unease for farmers relying on these models. XAI bridges this gap by allowing farmers to understand the reasoning behind recommendations from deep learning for agriculture. Visual explanations like heatmaps highlight areas in images that influence the model's decision, making it easier for farmers to grasp the "why" behind disease detection or yield predictions from deep learning for agriculture.
Informed Decision-Making
XAI doesn't just explain results from deep learning for agriculture; it helps farmers make informed choices. By understanding how factors like soil moisture or weather patterns contribute to a deep learning model's prediction, farmers can refine their agricultural practices. This knowledge allows them to adapt strategies based on the model's insights, leading to more effective resource allocation and improved outcomes in deep learning for agriculture.
Collaboration and Innovation
XAI fosters collaboration between farmers and AI developers in deep learning for agriculture. By understanding how models arrive at conclusions from these models, farmers can provide valuable feedback to developers. This two-way communication loop helps refine deep learning for agriculture models, ensuring they address the real-world needs of farmers and contribute to continuous innovation in the field of deep learning for agriculture.
Future Trends and Research Directions
Deep learning for agriculture is a rapidly evolving field with several exciting future trends and research directions:
Multimodal and multi-sensor data fusion: Deep learning models are increasingly being designed to integrate data from multiple sources, such as satellite imagery, drone sensors, soil sensors, and weather data. This allows for a more comprehensive understanding of agricultural systems and enables more accurate predictions.
Edge computing and on-farm data processing: Advancements in edge computing allow for real-time processing of data collected from farm sensors and drones. This reduces reliance on cloud computing and enables faster decision-making for farmers.
Reinforcement learning and autonomous systems: Reinforcement learning algorithms can be used to train autonomous robots for tasks like weed identification, crop thinning, and targeted pesticide application. This could revolutionize farm labor practices and improve efficiency.
Robotic automation and intelligent agricultural machinery: Deep learning can be used to develop intelligent agricultural machinery capable of performing tasks like autonomous navigation, obstacle detection, and precise application of inputs. This could significantly reduce labor costs and improve farm productivity.
Autonomous crop monitoring and intervention systems: Deep learning-powered drones and robots could be used for autonomous crop monitoring, allowing for real-time detection of problems and automated intervention measures like targeted irrigation or pest control.
Case Studies and Real-world Applications
Several companies and research institutions are already applying deep learning for agriculture. Here are some real-world examples:
Sairone by Saiwa: Sairone is an AI/ML-driven drone platform designed to optimize agricultural operations. It employs deep learning to analyze high-resolution aerial imagery, providing insights on habitat monitoring, crop health, and environmental conservation. Sairone assists farmers in detecting crop diseases at an early stage, monitoring soil health, and assessing water stress, thereby enabling timely interventions and improved yields.
PlantVillage: A mobile app that uses deep learning to diagnose plant diseases in real-time based on user-uploaded images.
The Climate Corporation: A company that uses deep learning to analyze weather data and satellite imagery to provide farmers with crop yield forecasts and risk management tools.
Blue River Technology: A company developing autonomous weeding robots that use deep learning to identify weeds and eliminate them precisely using targeted lasers.
DroneDeploy: A platform that allows farmers to use drones for aerial imagery capture and utilizes deep learning for automated crop health analysis and yield estimation.
These are just a few examples, and as deep learning technology continues to evolve, we can expect even more innovative applications to emerge in the coming years.
Conclusion
Deep learning has the potential to revolutionize agriculture by enabling data-driven decision making, automating repetitive tasks, and improving resource use efficiency. By applying deep learning across the entire agricultural production cycle, from planting to harvest and beyond, we can work towards a more sustainable, productive, and resilient food system for the future. However, addressing data management challenges, ensuring ethical practices, and fostering collaboration between researchers, farmers, and technology companies will be crucial for realizing the full potential of deep learning in agriculture.