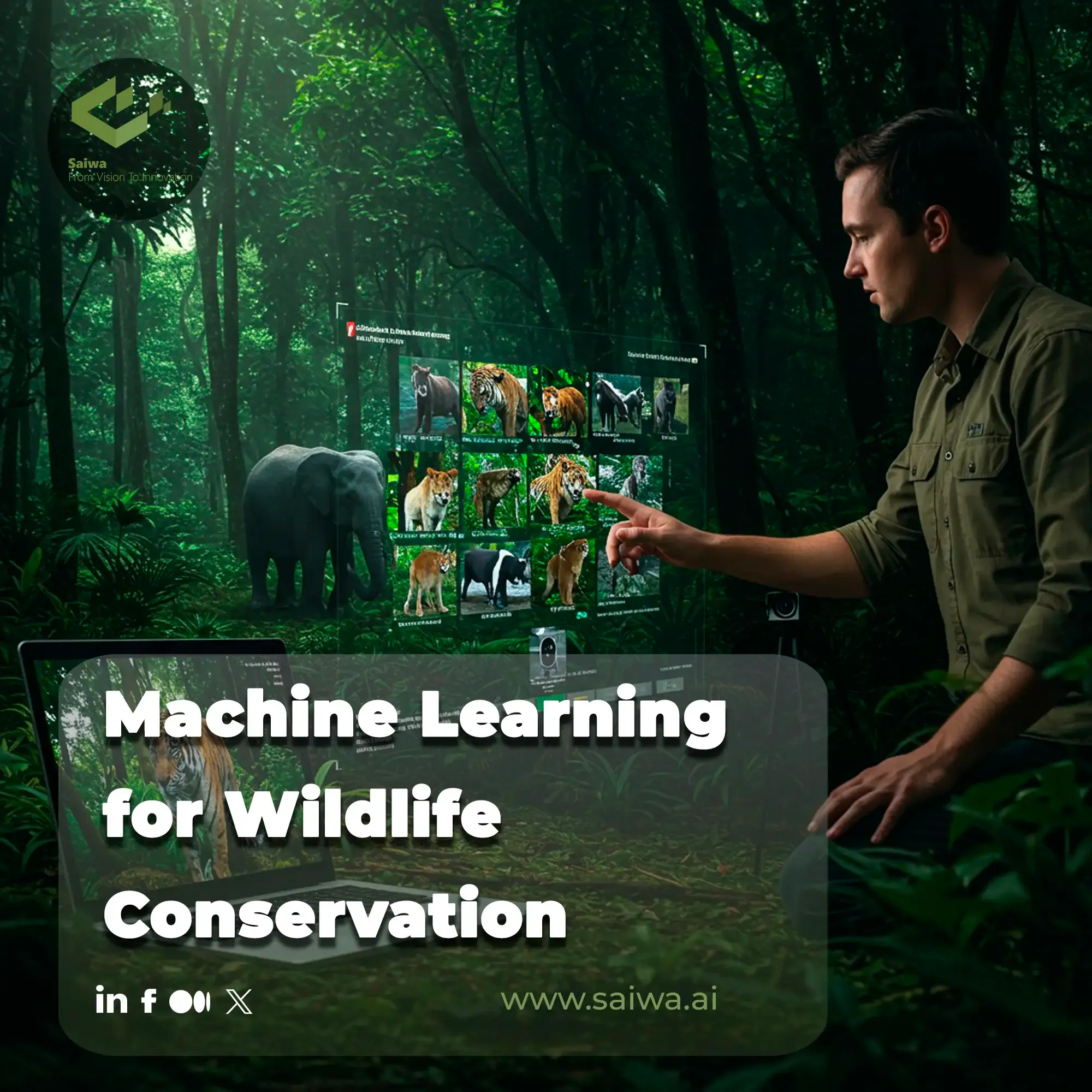
Wildlife conservation faces serious challenges, from habitat destruction and climate change to poaching and human-wildlife conflict. These threats are rapidly eroding biodiversity and destabilizing ecosystems around the world. While traditional conservation efforts are critical, they are often insufficient to address the scale and complexity of these issues. As the stakes rise, the need for innovative solutions has never been more urgent.
Sairone, developed by Saiwa, is changing the way wildlife conservation is approached. By combining drone technology with machine learning, Sairone provides a more efficient and non-intrusive way to monitor and protect wildlife. The platform provides real-time, data-driven insights that help conservationists and researchers make more informed decisions to protect endangered species and preserve vital ecosystems.
In this article, we'll explore how machine learning is revolutionizing wildlife conservation, discussing its key applications, real-world impact, and the challenges and ethical considerations that come with it.
The History of Machine Learning in Wildlife Conservation
Machine learning's role in wildlife conservation is still relatively new, but it has quickly become a game changer. Its roots can be traced back to early efforts in pattern recognition and the automation of species identification. Initially, machine learning was used to analyze images and acoustic data to identify individual animals and classify species.
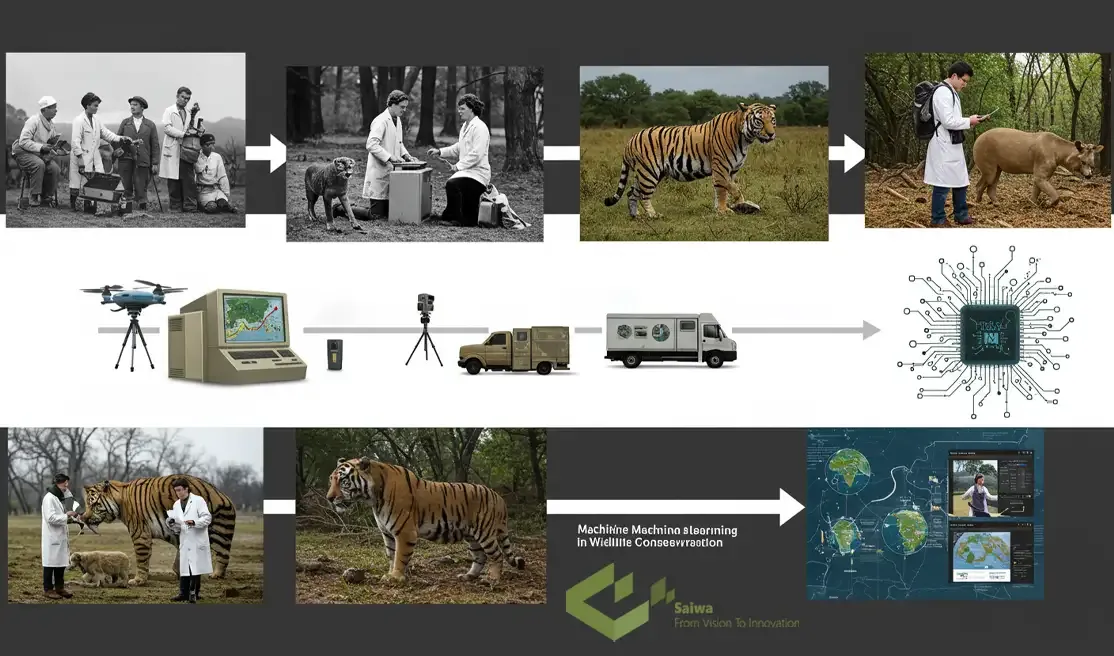
As computing power, data storage, and algorithms have evolved, machine learning has expanded into new areas of conservation. Today, it plays a key role in habitat mapping, poaching detection, disease surveillance, and understanding migration patterns. The availability of massive datasets combined with cutting-edge algorithms has opened up exciting possibilities, enabling smarter, data-driven conservation strategies that were once unimaginable.
Core Applications of ML in Conservation
Machine learning is being applied across a wide range of conservation domains, providing valuable insights and enhancing conservation strategies.
Image and Video Analysis
Machine learning algorithms can be trained to analyze images and videos of wildlife, automating species identification, counting individuals, and monitoring animal behavior. This technology significantly reduces the time and effort required for manual analysis, consequently enabling researchers to process large datasets efficiently and extract valuable insights into population dynamics, animal behavior, and ecological interactions. Furthermore, ML-powered image analysis can identify subtle patterns and anomalies that might be missed by human observers, providing deeper insights into wildlife ecology and behavior.
Acoustic Monitoring
Acoustic monitoring, combined with machine learning, provides a powerful tool for monitoring biodiversity, identifying species based on their vocalizations, and detecting poaching activities. ML algorithms can be trained to recognize the unique calls of different species, even in noisy environments, enabling researchers to monitor biodiversity, track the movement of animals, and detect the presence of endangered species.
Acoustic monitoring can also be used to detect gunshots, chainsaws, and other sounds associated with poaching, providing valuable information for law enforcement efforts.
Drone and Aerial Surveillance
Drones equipped with cameras and sensors, combined with machine learning algorithms, provide a cost-effective and efficient platform for monitoring; used in wildlife populations, mapping habitats, and detecting poaching activities.
ML algorithms can analyze drone imagery to identify individual animals, classify species, detect poaching activities, and monitor changes in habitat over time. This technology enables real-time monitoring of protected areas, providing valuable information for law enforcement and conservation management.
Geospatial and Satellite Analysis
Machine learning algorithms can analyze geospatial data from satellites and other sources to map habitats, monitor deforestation, assess the impact of climate change on ecosystems, and predict the spread of invasive species.
This information is crucial for identifying critical habitats, prioritizing conservation efforts, and developing effective management strategies. ML-powered geospatial analysis can also be used to predict the future distribution of species under different climate change scenarios, informing proactive conservation planning.
Genomic and Health Data Analysis
Machine learning offers powerful tools for analyzing genomic and health data in wildlife. It can help to better understand animal health, track disease spread, and even predict potential outbreaks. By examining genetic information, ML algorithms can identify individual animals, measure genetic diversity, and uncover the evolutionary history of species.
These insights are essential for guiding captive breeding programs, protecting endangered populations, and assessing how environmental changes affect wildlife health.
Combating Wildlife Poaching and Illegal Trade
Machine learning is being used to develop intelligent systems for detecting and combating wildlife poaching and illegal trade. ML algorithms can analyze data from various sources, including social media, online marketplaces, and law enforcement databases.
This data aid in identifying patterns associated with illegal wildlife trade, predicting poaching hotspots, and tracking the movement of illegal wildlife products. This information can ultimately be used to inform law enforcement efforts, disrupt trafficking networks, and reduce the demand for illegal wildlife products.
Migration Pattern Analysis
Machine learning can analyze data from GPS trackers, satellite tags, and other sources to understand animal migration patterns, identify critical habitats, and assess the impact of environmental change on animal movements.
This information is not only crucial for designing protected areas, but also managing migratory corridors, and mitigating human-wildlife conflict. ML-powered migration pattern analysis can also be used to predict the future movements of animals under different climate change scenarios, informing proactive conservation planning.
Real-World Impact of Machine Learning for Wildlife Conservation
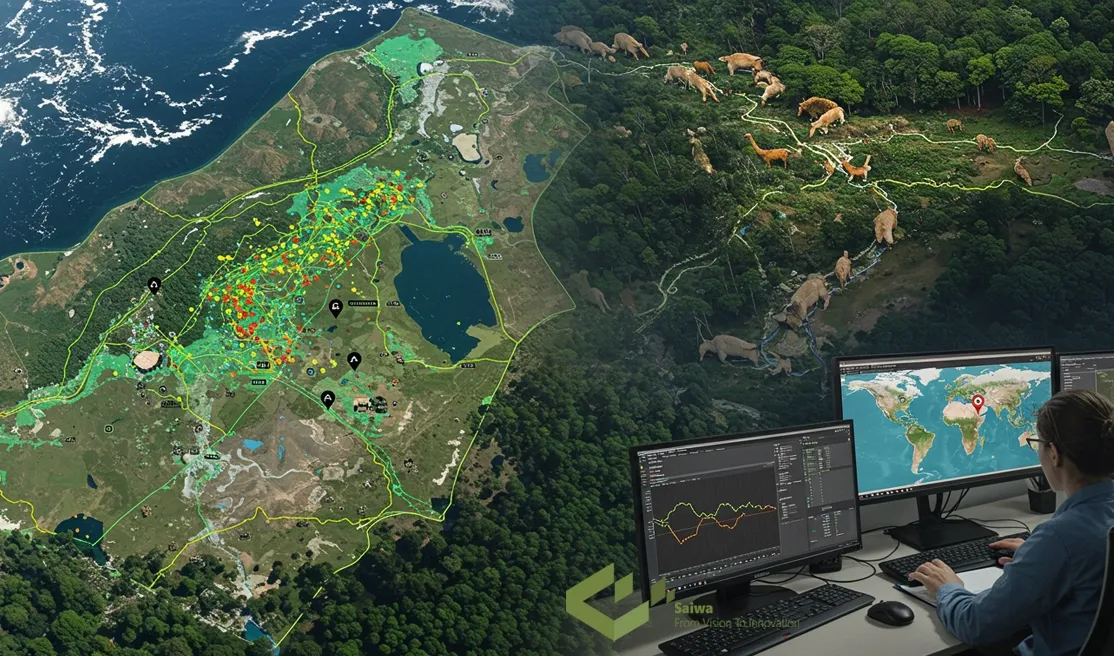
Several successful projects demonstrate the real-world impact of machine learning in wildlife conservation.
Nesher Bari Project
The Nesher Bari project utilizes machine learning to predict vulture mortality risks in Israel. Therefore, enabling proactive interventions to prevent poisoning incidents and protect these critically endangered birds from accidental or intentional poisoning. By analyzing data on vulture movements, foraging behavior, environmental factors, and known poisoning locations, the project can identify areas where vultures are at high risk of poisoning and implement targeted mitigation measures.
These measures include removing carcasses laced with poison or educating local communities about the dangers of poisoning wildlife. This proactive approach is helping to reduce vulture mortality and contribute to the recovery of these vital scavengers.
Wildbook
Wildbook utilizes machine learning algorithms to identify individual animals based on their unique coat patterns, whisker spot patterns, and other distinguishing features, providing a non-invasive method for tracking individuals over time.
This technology not only enables researchers to track the movement of animals, but also helps monitor population dynamics, study animal behavior, and assess the effectiveness of conservation interventions without the need for invasive tagging or capture.
This method minimizes stress on animals and provides valuable long-term data. Wildbook has been successfully applied to a variety of species, including whales, dolphins, zebras, giraffes, and other species with unique markings.
AI Drones for Rhino and Big Cat Protection
AI-powered drones are being used to protect rhinos and big cats from poachers in Africa, enhancing surveillance capabilities and enabling rapid response to poaching threats. These drones are equipped with thermal cameras and machine learning algorithms that can detect poachers in real-time, even in dense vegetation or at night, alerting rangers to their presence and enabling rapid response.
This technology is significantly improving the effectiveness of anti-poaching efforts, helping to protect these iconic species from extinction, deterring poaching activities, and increasing the chances of apprehending poachers.
Challenges and Ethical Considerations of Machine Learning for Wildlife Conservation
While machine learning offers immense potential for wildlife conservation, it also presents several challenges and ethical considerations.
Data Limitations
Machine learning algorithms require large amounts of high-quality, labeled data for training, which can be a significant bottleneck in conservation applications. Collecting and labeling this data can be time-consuming, expensive, and logistically challenging, particularly in remote or difficult-to-access areas, Therefore, requiring specialized expertise and resources.
The lack of sufficient data can limit the accuracy and effectiveness of machine learning models, highlighting the need for innovative data collection strategies and data sharing initiatives.
Technical and Financial Barriers
Implementing machine learning in wildlife conservation requires specialized technical expertise, access to computational resources, and adequate funding. Many conservation organizations lack the necessary expertise and resources to develop and deploy machine learning models, limiting the widespread adoption of this technology, particularly in resource-constrained environments.
Bridging this gap requires capacity building initiatives, technology transfer programs, and increased funding for machine learning in conservation.
Ethical Implications
The use of machine learning in wildlife conservation raises several ethical considerations, including data privacy, algorithmic bias, and the potential for misuse of technology. It is essential to ensure that machine learning is used responsibly and ethically, protecting the privacy of wildlife, respecting the rights of local communities, and avoiding unintended consequences. Developing ethical guidelines and best practices for the use of machine learning in conservation is crucial for ensuring its long-term benefits.
The Future of ML in Wildlife Conservation
The future of machine learning in wildlife conservation is bright, with ongoing advancements in technology, increasing data availability, and growing awareness of its potential.
Community and Citizen Science
Citizen science initiatives can play a crucial role in collecting and labeling data for machine learning models, expanding data availability, reducing costs, and engaging the public in conservation efforts.
Mobile apps, online platforms, and community-based monitoring programs can empower citizen scientists to contribute data on wildlife sightings, animal behavior, and environmental conditions. These apps provide valuable information for training machine learning models and enhancing the scale and scope of conservation monitoring.
Policy and Collaboration
Effective policy frameworks and collaborative partnerships are essential for ensuring the responsible and ethical use of machine learning in wildlife conservation, maximizing its benefits while minimizing potential risks. International collaborations, data sharing agreements, ethical guidelines, and supportive policies can promote best practices, address data privacy concerns, facilitate technology transfer, and maximize the benefits of machine learning for conservation, creating a supportive environment for innovation and collaboration.
Technological Advancements
Ongoing advancements in machine learning technology, including deep learning, computer vision, cloud computing, and edge computing, are creating new possibilities for wildlife conservation. These advancements are enabling the development of more sophisticated and accurate machine learning models, expanding the applications of this technology, improving real-time data analysis capabilities; Enhancing its impact on conservation efforts, paving the way for more effective and data-driven conservation strategies.
Conclusion
Machine learning is becoming an essential tool in wildlife conservation, offering innovative solutions to the many challenges facing endangered species and ecosystems. By analyzing vast amounts of data and uncovering patterns that might go unnoticed by the human eye, it's helping conservationists make smarter, more informed decisions. These insights enable faster, more effective action, whether it's preventing poaching or protecting habitats.
While there are still challenges to overcome, such as ethical considerations and data gaps, the future of machine learning in conservation is promising. With advances in technology and increased access to data, we have the opportunity to make a significant, lasting impact. By working together, using technology responsibly, and staying open to new approaches, we can ensure a more sustainable future for wildlife and their habitats.