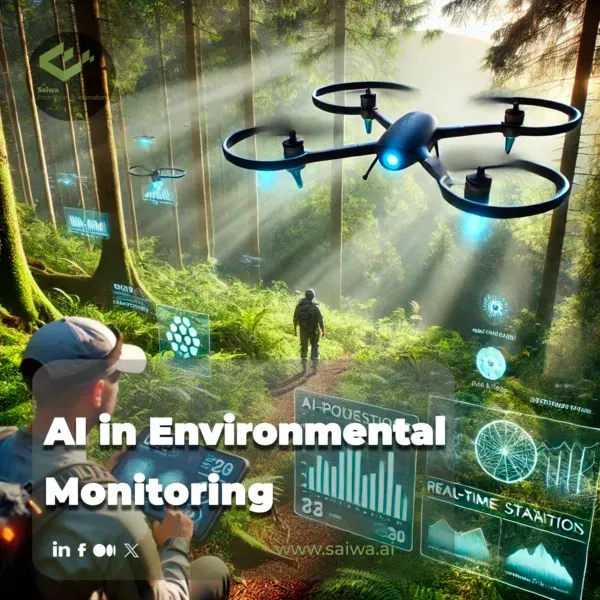
Environmental monitoring, defined as the systematic observation and assessment of environmental conditions, is critical to understanding and mitigating the impacts of human activities on the planet. Traditional methods of environmental monitoring often involve manual data collection and analysis, a process that can be time-consuming, expensive, and limited in scope. The advent of artificial intelligence (AI) has revolutionized various fields, including environmental monitoring, offering unprecedented opportunities to enhance our ability to observe, analyze, and protect the environment.
This article explores the importance, techniques, applications, benefits, and challenges of using AI in environmental monitoring.
Importance of AI in Environmental Monitoring
The increasing complexity and scale of environmental challenges, coupled with the growing availability of environmental data, necessitate more sophisticated and efficient monitoring approaches. AI offers several advantages over traditional methods:
Automation
AI can automate data collection, processing, and analysis, significantly reducing manual effort and improving efficiency. This automation frees up human resources for more complex tasks such as interpretation and decision-making. It also enables continuous monitoring, which is often impractical with manual methods.
Real-time Insights
AI algorithms can analyze data in real-time, providing timely alerts and insights into emerging environmental issues. This allows for rapid responses to environmental incidents, minimizing potential damage. Real-time data analysis also facilitates proactive management strategies.
Predictive Capabilities
AI models can be trained to predict future environmental conditions, enabling proactive interventions and mitigation strategies. Predictive models can forecast pollution levels, climate change impacts, and other environmental risks, allowing for preemptive action.
Pattern Recognition
AI can identify complex patterns and relationships in environmental data that may not be apparent through traditional analysis. This can reveal hidden connections between different environmental factors, leading to a deeper understanding of ecosystem dynamics.
Improved Accuracy
AI can enhance the accuracy of environmental monitoring by reducing human error and incorporating diverse data sources. AI algorithms can handle large and complex datasets, integrating information from various sensors, satellites, and other sources to provide a more comprehensive and accurate picture of environmental conditions.
AI Techniques in Environmental Monitoring
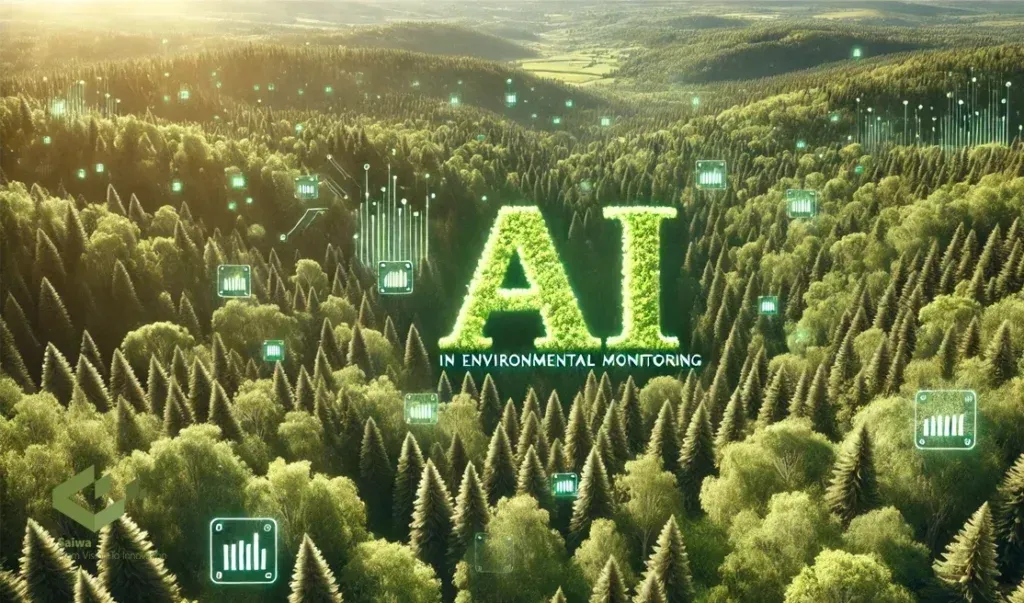
Several AI techniques are employed in environmental monitoring:
Machine learning, deep learning, and data analytics
Machine learning models, including deep learning models, are used to analyze large environmental datasets, identify patterns, and make predictions. Supervised learning techniques, such as regression and classification, can be used to predict environmental variables or classify different types of environmental events.
Unsupervised learning techniques, such as clustering and dimensionality reduction, can be used to identify patterns and relationships in environmental data. Deep learning models, with their ability to learn complex patterns from high-dimensional data, are particularly well-suited for analyzing complex environmental datasets, such as satellite imagery and sensor data.
Advanced data analytics techniques, including time series analysis and spatial analysis, are used to extract meaningful insights from environmental data.
Sensors and IoT integration in AI-driven systems
The Internet of Things (IoT) enables the deployment of a vast network of interconnected sensors that collect real-time environmental data. These sensors can measure various parameters, such as air quality, water quality, temperature, humidity, and soil moisture.
AI algorithms can then analyze the data from these sensors to identify anomalies, predict future conditions, and trigger alerts. The integration of sensors and IoT with AI-driven systems enables continuous and comprehensive environmental monitoring, providing a rich source of data for analysis and decision-making. Edge computing, where data processing occurs closer to the sensor, is becoming increasingly important for reducing latency and enabling real-time analysis.
The Applications of AI for Environmental Monitoring
AI is being applied to a wide range of environmental monitoring tasks:
Air Quality Monitoring
AI algorithms can analyze data from air quality sensors, meteorological data, and traffic patterns to predict air pollution levels, identify pollution sources, and assess the impact of air pollution on human health.
AI-powered platforms can provide real-time air quality information to the public and inform policy decisions aimed at improving air quality. This can lead to more targeted interventions and public health advisories. AI can also help optimize traffic flow to minimize emissions in urban areas.
Water Quality Monitoring
AI can analyze data from water quality sensors, remote sensing imagery, and hydrological models to monitor water quality parameters, detect pollution events, and assess the health of aquatic ecosystems.
AI can also be used to predict the spread of pollutants in water bodies and optimize water management strategies. This information can be used to protect drinking water sources and manage water resources more effectively. AI can also help identify the sources of pollution and track their impact on aquatic life.
Deforestation Detection
AI algorithms, particularly deep learning models trained on satellite imagery, can detect deforestation activities in real-time, enabling rapid response and enforcement actions. AI can also be used to monitor forest health, assess the impact of climate change on forests, and support sustainable forest management practices.
This can help prevent illegal logging and protect biodiversity. AI can also be used to monitor reforestation efforts and assess their effectiveness.
Wildlife Conservation
AI can analyze data from camera traps, acoustic sensors, and tracking devices to monitor wildlife populations, identify endangered species, and track animal movements. AI in wildlife conservation can also be used to detect poaching activities and support anti-poaching efforts.
This information can be used to develop targeted conservation strategies and protect endangered species from extinction. AI can also help understand animal behavior and migration patterns.
Climate Change Monitoring
AI can analyze climate data from various sources, including satellites, weather stations, and climate models, to monitor climate change trends, predict future climate scenarios, and assess the impacts of climate change on different ecosystems and human populations.
AI can also be used to optimize climate mitigation and adaptation strategies. This information can inform policy decisions and guide investments in climate resilience. AI can also help identify the most effective strategies for reducing greenhouse gas emissions.
Benefits of AI for Environmental Monitoring
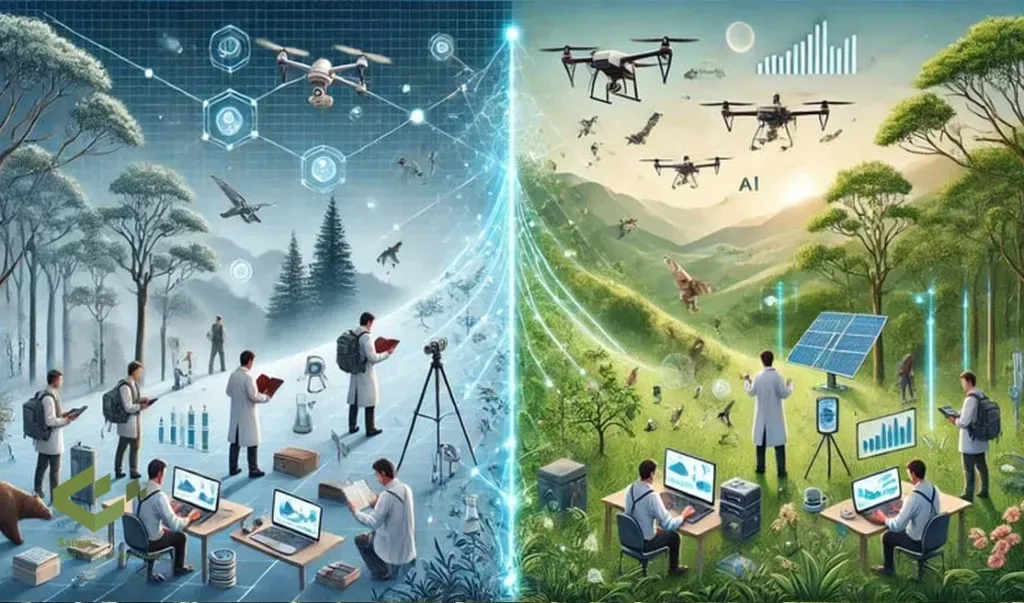
The application of AI in environmental monitoring offers numerous benefits:
Real-Time Data Analysis
AI enables real-time analysis of environmental data, providing immediate insights into emerging issues and enabling timely interventions. This rapid analysis can be crucial for responding to pollution events, natural disasters, and other time-sensitive environmental changes. Real-time data also allows for continuous monitoring and adaptive management strategies.
Enhanced Accuracy
AI algorithms can improve the accuracy of environmental monitoring by reducing human error and incorporating diverse data sources. AI can handle complex datasets and identify subtle patterns that might be missed by manual analysis. This improved accuracy leads to better informed decisions and more effective environmental management.
Cost-Effectiveness
AI can automate many aspects of environmental monitoring, reducing the need for manual data collection and analysis, thus lowering costs. Automation minimizes labor-intensive tasks, freeing up resources for other important activities. The long-term cost savings can be substantial.
Scalability
AI-powered systems can be easily scaled to monitor large geographical areas and diverse environmental parameters. This scalability is essential for addressing global environmental challenges like climate change and biodiversity loss.
AI can analyze data from a vast network of sensors and satellites, providing a comprehensive view of environmental conditions across large regions.
Challenges of AI in Environmental Monitoring
Despite the significant potential of AI in environmental monitoring, several challenges need to be addressed:
Data privacy and security concerns
The increasing use of AI in environmental monitoring raises concerns about data privacy and security. Sensitive environmental data needs to be protected from unauthorized access and misuse.
Robust data governance frameworks and security protocols are essential for ensuring the responsible use of AI in environmental monitoring. Data anonymization and encryption techniques can help protect sensitive information. Clear guidelines on data ownership and access are also needed.
Access to quality data and the need for large datasets
AI algorithms, particularly deep learning models, require large amounts of high-quality data for training. Access to reliable and representative environmental data can be a challenge, especially in developing countries. Efforts to improve data collection, standardization, and sharing are crucial for maximizing the potential of AI in environmental monitoring.
Data gaps can limit the effectiveness of AI models, and biases in existing datasets can perpetuate inequalities. Open data initiatives and collaborative data sharing platforms can help address these challenges.
Computational power and resource limitations
Training and deploying complex AI models can require significant computational resources, which may not be readily available in all settings. Cloud computing and edge computing can help address these limitations, but access to these technologies can be a barrier in some regions.
The energy consumption of AI models is also a concern, and research into more energy-efficient algorithms is needed. Optimizing models for deployment on resource-constrained devices is another important area of development.
Ethical considerations in AI applications for the environment
The use of AI in environmental monitoring raises ethical considerations, such as the potential for bias in algorithms, the impact on human jobs, and the responsibility for decisions made by AI systems. Careful consideration of these ethical implications is essential for ensuring the responsible and beneficial use of AI in environmental monitoring.
Transparency and explainability of AI models are crucial for building trust and accountability. Societal discussions and ethical guidelines are needed to navigate the complex ethical landscape of AI in environmental applications.
Conclusion
The field of environmental monitoring is being transformed by artificial intelligence (AI), which is providing powerful tools for observing, analyzing, and protecting our planet. AI-powered systems can automate data collection and analysis, provide real-time insights, predict future conditions, and identify complex patterns in environmental data. While challenges remain, the continued development and application of AI in environmental monitoring holds immense promise for addressing critical environmental challenges and building a more sustainable future.
By leveraging the potential of AI, we can improve our ability to understand and manage the complex interactions between human activities and the environment, paving the way for a healthier and more resilient planet.