Smart Agriculture, One Flight at a Time
Sairone is an AI/ML-drone platform that makes agriculture more efficient, and environmental monitoring smarter.
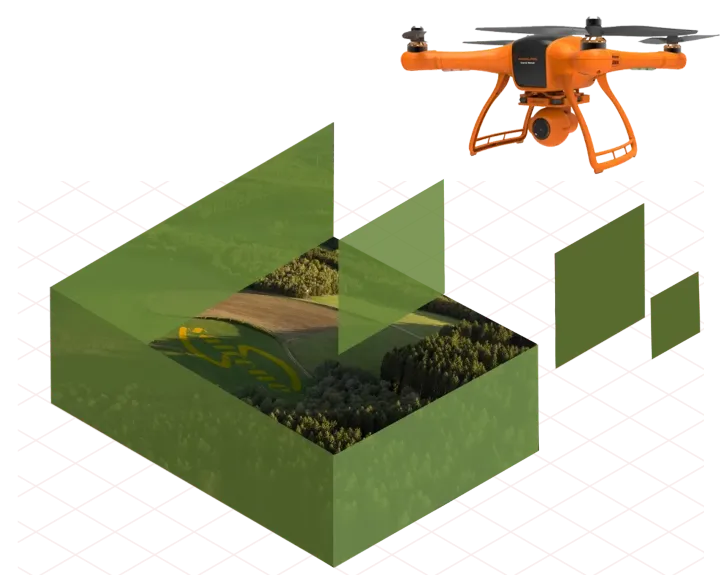
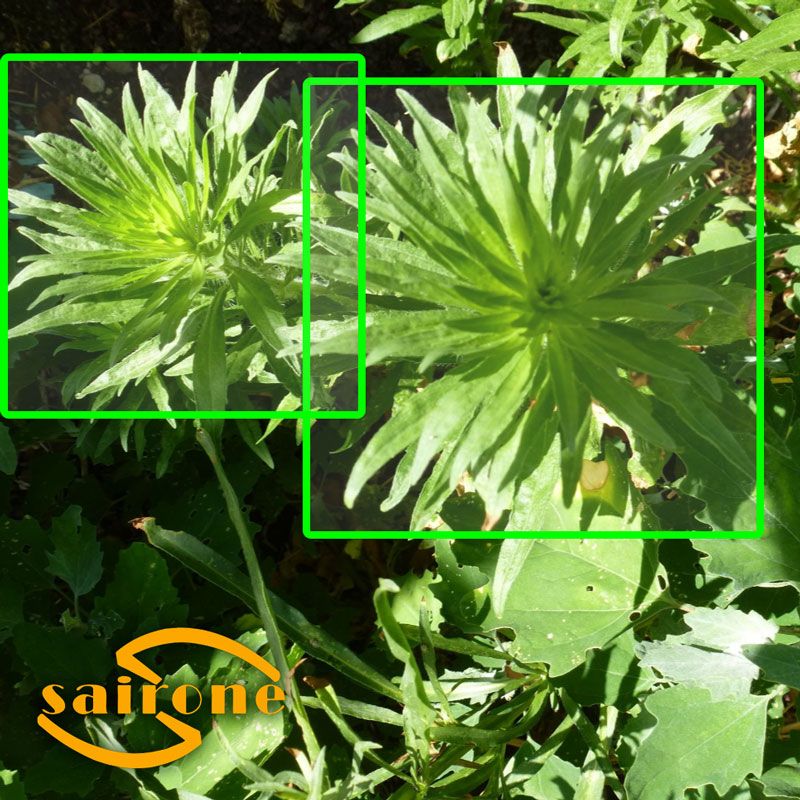
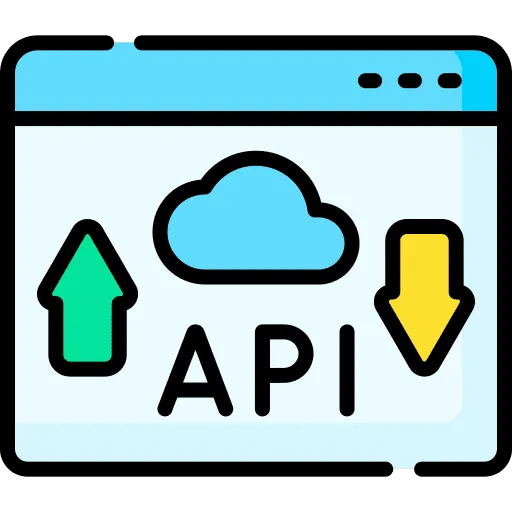
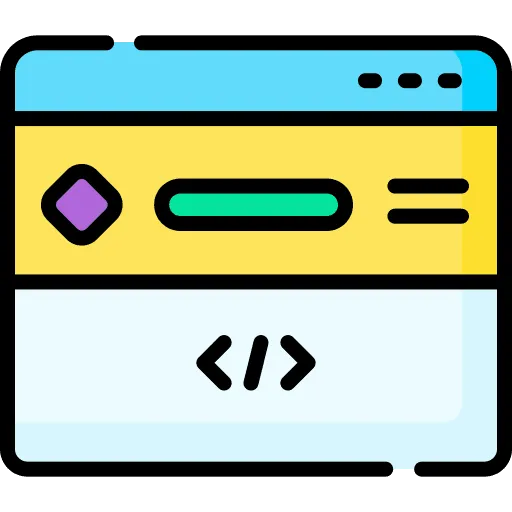
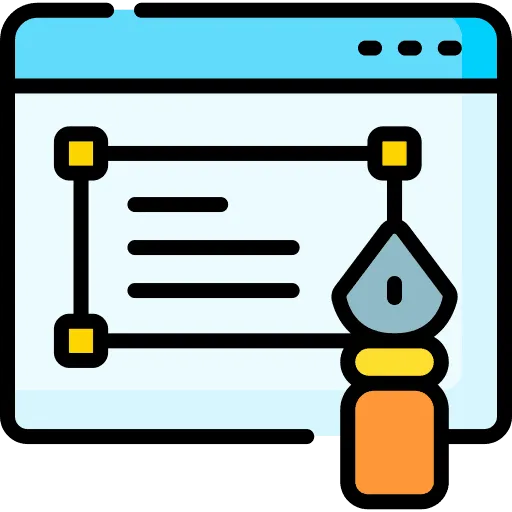
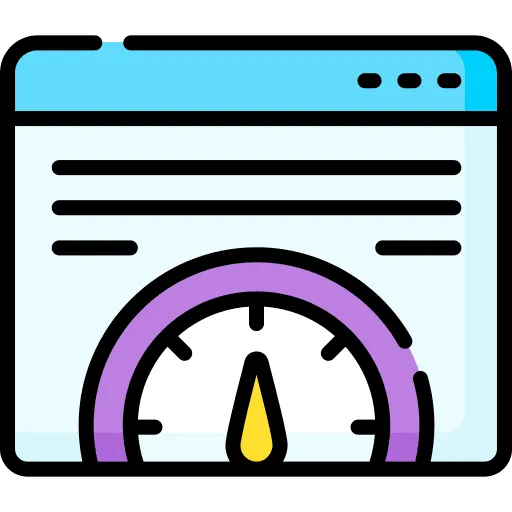
Save Time and Increase Precision
For Ducks Unlimited Canada, we developed a platform leveraging deep learning and GPS integration to enhance invasive species detection and protect aquatic ecosystems. By automating GPS and species identification using DUC's flight data, Sairone streamlined detection efforts, allowing field workers to focus on their priorities like pinpointing species, tracking trends, and safeguarding Canadian wetlands. Read more in this article.
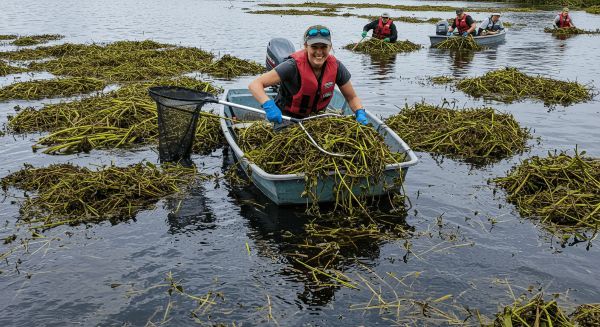
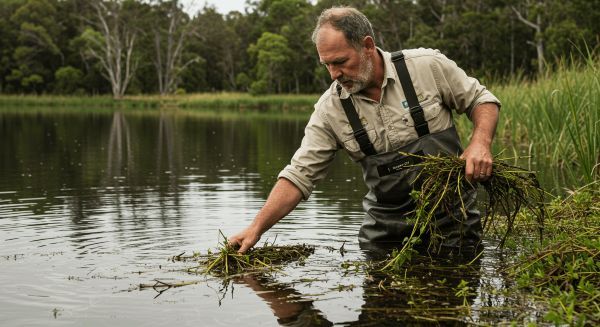
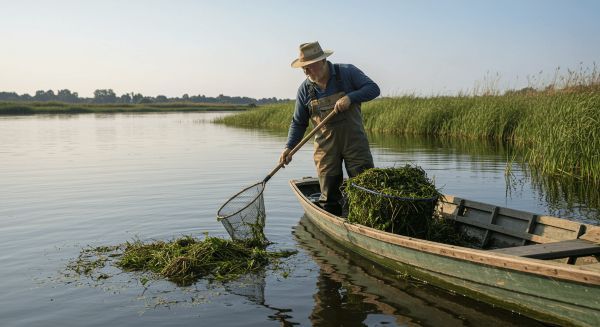
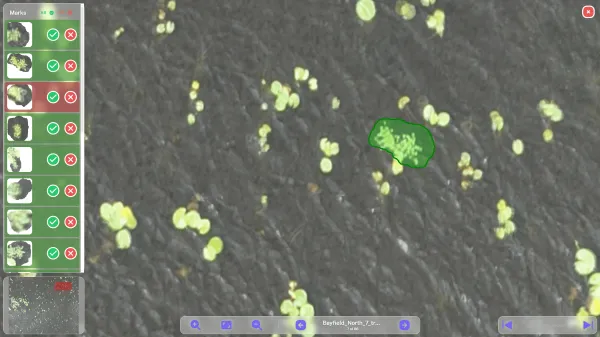
Weed and Invasive Plant Control Solution
✨ Automated plant identification
✨ Drone image processing
✨ Automatic clustering and polygon formation
✨ Offline capability
✨ Species-specific plant identification
✨ Orthomosaic processing
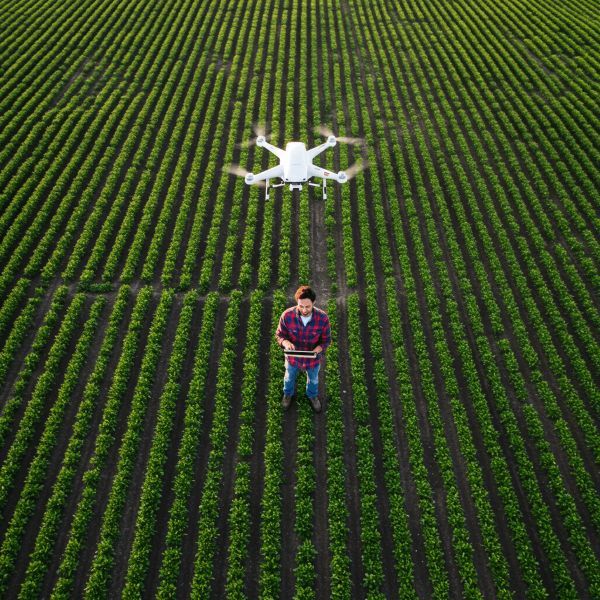
Wildlife Conservation
✨ Al-Powered Wildlife Monitoring
✨ Predictive Wildlife Detection & Risk Mitigation
✨ Animal Population Counting & Tracking
✨ Anti-Poaching & Security Surveillance
✨ Wildlife Translocation & Ecosystem Restoration
✨ Machine Learning for Wildlife Insights
✨ Non-Invasive Monitoring
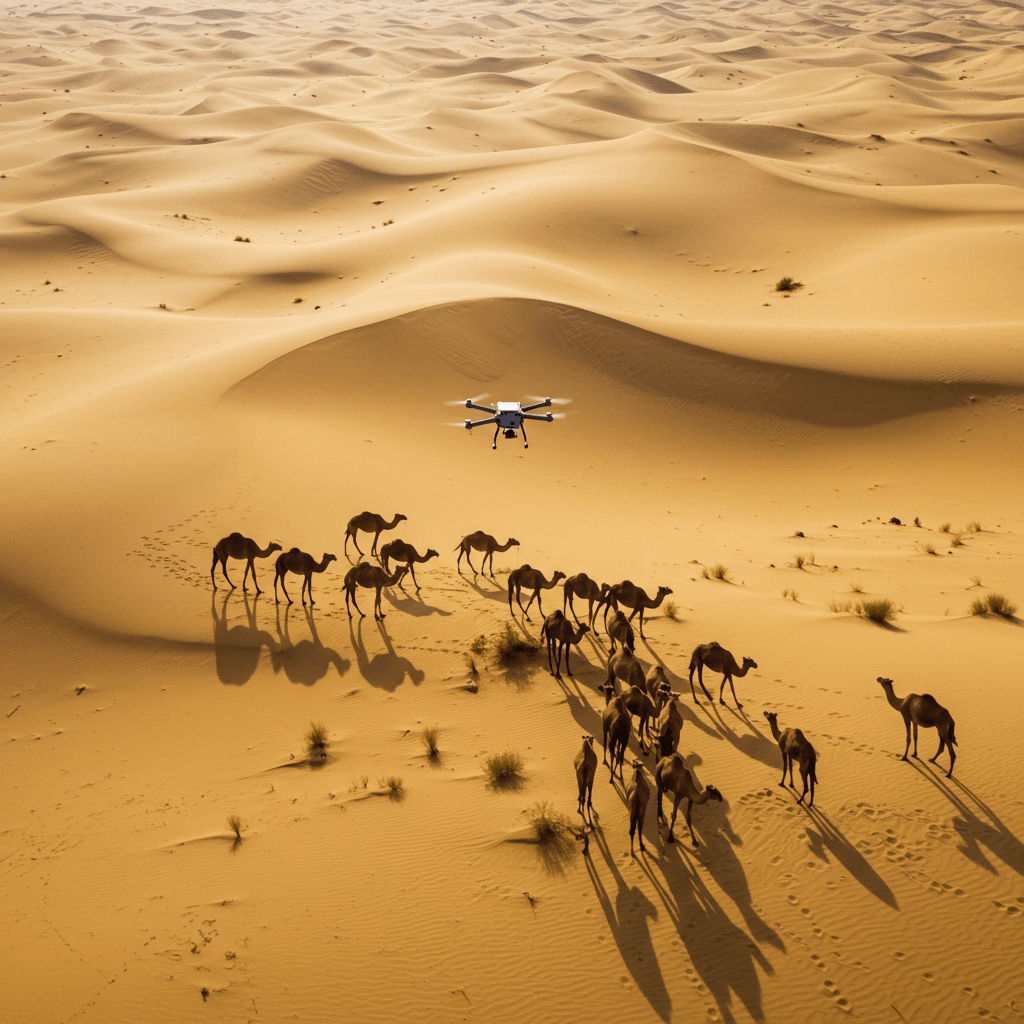
Fleabane Detector
✨ Real-Time Weed Detection
✨ Drone & Camera Image Support
✨ Customizable Detection Settings
✨ Visual Results Dashboard
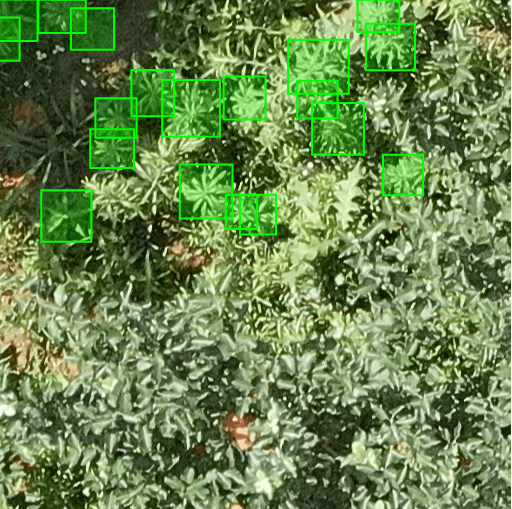
Crop Yield Estimation (Coming Soon)
✨ Crop detection and localization
✨ Blossom Counting
✨ Crop counting
✨ Nitrogen content estimation
✨ Weather and soil analysis
✨ Stand counting
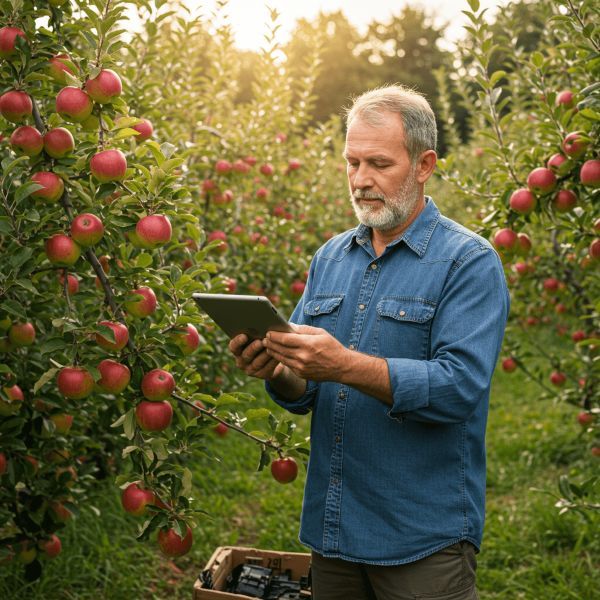
Need your own solution?
Let Us Know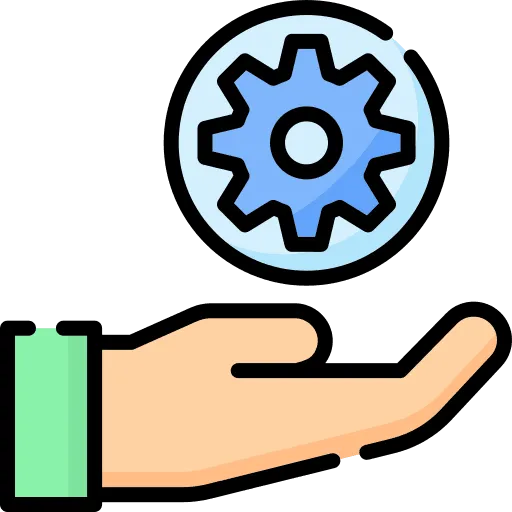
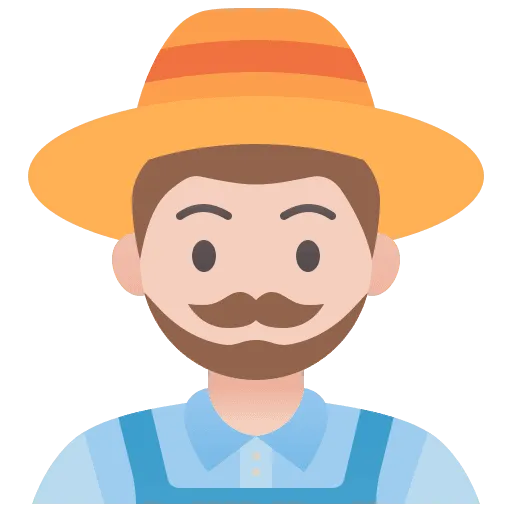
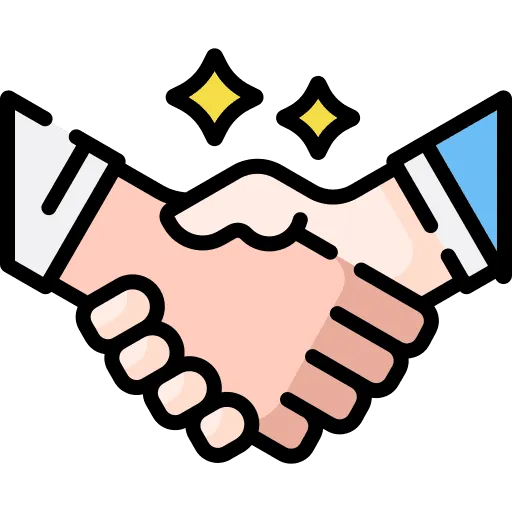
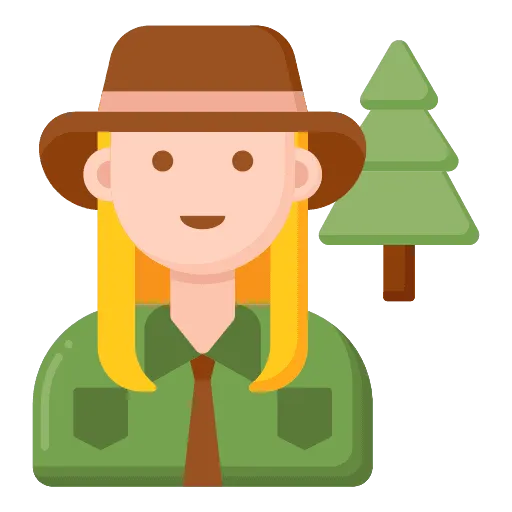
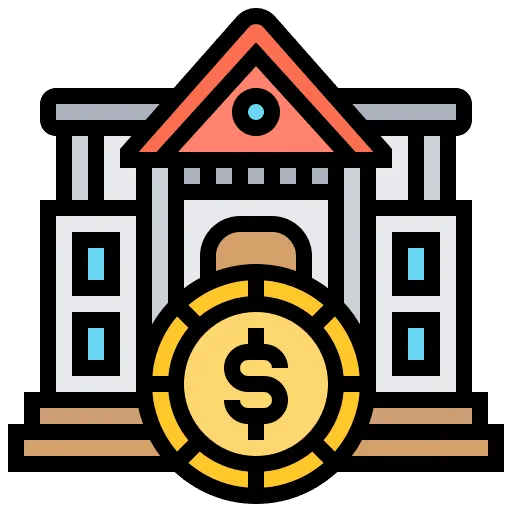
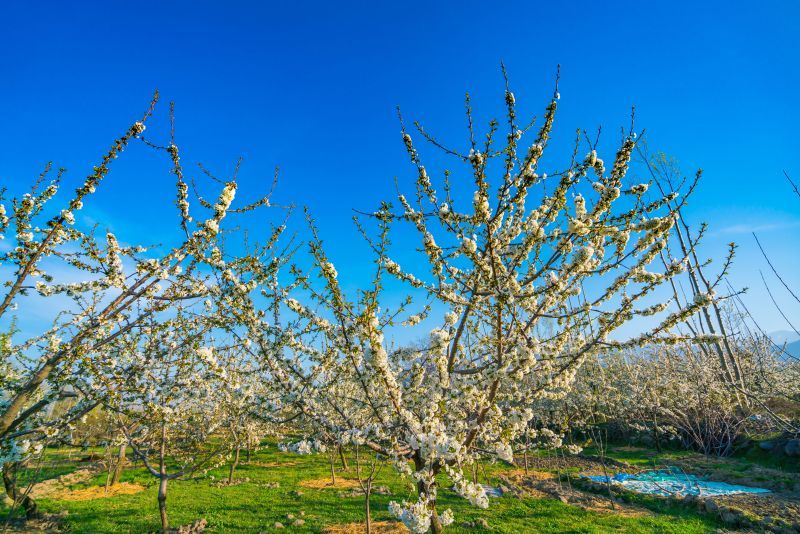
Enhancing Apple Blossom Detection with Sairone
In 2024, the Cropmind team partnered with Sairone to accurately detect and count apple blossoms per tree. Using data collected by Cropmind, our platform provided precise blossom detection, empowering their team with actionable insights for better orchard management.
Additionally, we developed a white-labeled version of our crop detection service, customized for their use from January to September 2024. This seamless integration helped streamline their workflow and optimize their decision-making process. Sairone’s advanced AI capabilities proved instrumental in transforming their approach to crop monitoring.
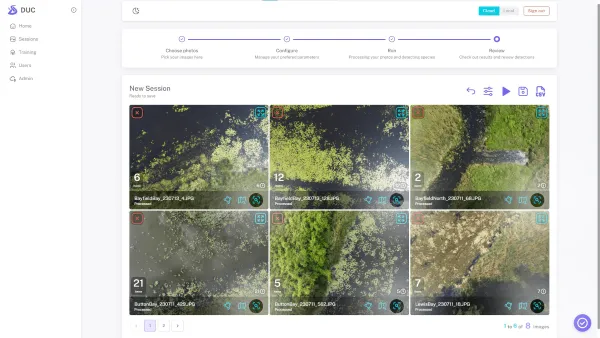
Enhancing UAV-Based Surveillance for European Water Chestnut Detection
In collaboration with Ducks Unlimited Canada (DUC), Saiwa has been instrumental in advancing the detection and surveillance of European Water Chestnut through cutting-edge machine learning solutions. Building on the success of our first project, where we delivered the initial version of the EWC Detector software, this initiative focuses on upgrading product features, enhancing interfaces, and streamlining workflows for DUC’s UAV-based surveillance efforts.
The project includes three phases: implementing incremental learning methods for improved detection accuracy, developing universal coordination features for precise mapping, and designing an intuitive user interface. By delivering these advanced functionalities, Saiwa continues to empower DUC with state-of-the-art tools to monitor and manage invasive species effectively.
We offer a number of AI powered drone applications to automate the processing of high-resolution aerial images in agriculture, greenhouse, ecology, habitat monitoring and environmental conservation. Our applications compile the drone imagery, generating customer-requested reports with high precision and efficiency, ultimately accelerating knowledge synthesis by removing the tedious aspects of dealing with large and complex data.