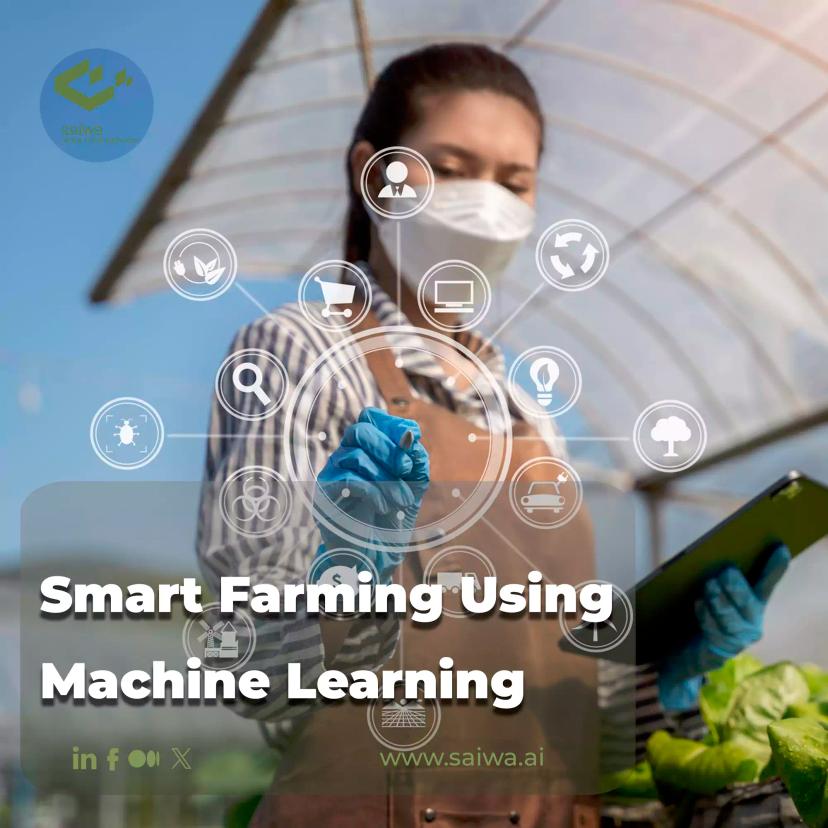
Unlocking the Potential of Smart Farming Using Machine Learning
Smart farming using machine learning refers to data-driven agricultural practices that leverage technology to optimize efficiency, sustainability, and crop yields. Machine learning, a subset of artificial intelligence, is playing a growing role in smart agriculture through its ability to uncover hidden insights from vast datasets.
Machine learning algorithms apply statistical techniques to iteratively "learn" from data and improve at making predictions or optimizing outcomes over time without explicit programming. In agriculture, machine learning enables the processing of immense amounts of farm data to guide everything from irrigation schedules to disease prevention programs. Its flexible predictive capabilities facilitate more precise interventions tailored to localized conditions in the field.
Overall, integrating machine learning into agricultural management through predictive analytics and automation holds immense potential to increase food production and economic livelihoods for farmers while minimizing ecological impacts. However, thoughtfully addressing concerns around data security, accessibility, and technical integration remains imperative to ensure responsible and ethical machine learning deployment.
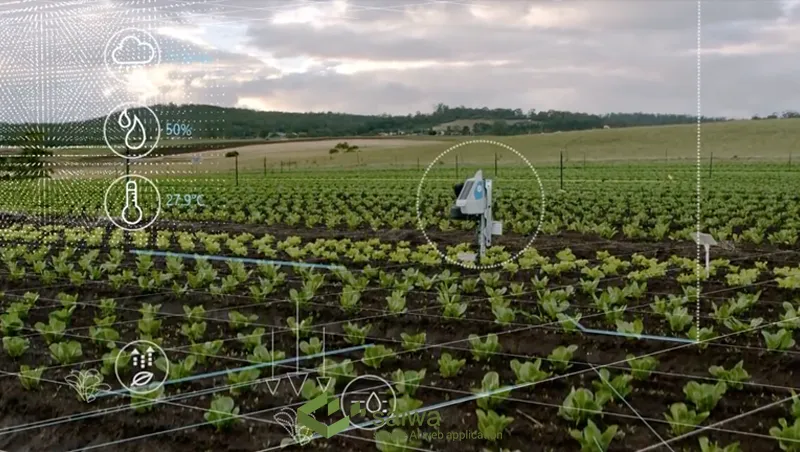
Machine Learning Applications in Agriculture
Several key applications demonstrate smart farming using machine learning:
For crop monitoring, computer vision applied to drone or satellite imagery reveals crop health patterns while sensors analyze soil composition to determine micronutrient deficiencies and moisture needs at a hyper-local level, enabling corrective actions. By analyzing these factors, farmers can optimize irrigation, fertilizer application, and other cultivation practices to maximize productivity while minimizing resource waste. Machine learning algorithms can analyze images or sensor data to detect signs of diseases or pest infestations at an early stage. This enables farmers to take prompt action, preventing widespread damage and minimizing the need for harmful chemical treatments.
Based on weather data, ground sensor data, and crop growth models, machine learning analytics can develop highly customized prescription maps specifying optimal fertilizer blends, seed varieties, and planting densities suited to every square meter to maximize yields in each zone.
By forecasting weather using neural networks, machine learning quantifies cultivation risk, allowing farmers to hedge with insurance or protective equipment. Longer-range climate projections also guide breeding decisions.
Read more: The impact of machine learning in agriculture.
Machine Learning in Smart Farming
These are the types of machine learning algorithms relevant to smart farming:
Supervised learning: algorithms trained on labeled datasets to predict outcomes or classify new data points
Unsupervised learning: algorithms analyze unlabeled data to discover underlying patterns or groupings.
Reinforcement learning: Algorithms learn through a trial-and-error approach, receiving feedback based on their actions.
Data Collection, Analysis, and Real-Time Decision-Making
Machine learning algorithms require quality agricultural data.
Internet of Things (IoT) sensors like soil probes, drones, and wearables constantly gather data on metrics like moisture, pests, animal behavior, and equipment use which feeds models. Edge computing enables real-time analytics.
Cloud platforms analyze aggregated sensor data to uncover trends, predict outcomes, and continually enhance algorithm accuracy. Humans interpret insights for actionability.
Real-time data flows via digital interfaces inform instant operational decisions like selective harvesting based on ripeness predictions, protecting crops using weather alerts, and adjusting irrigation based on water stress.
Benefits of Applying Predictive Analytics in Smart Farming
Predictive analytics in smart farming delivers a wide array of benefits when leveraged in an agricultural context:
- Optimize crop selection, acreage, and planting schedules by modeling predicted yields under various crop, soil, and weather conditions.
- Improve irrigation efficiency by using weather forecasts and predictive crop water demand models to only irrigate as needed.
- Data-driven pest and disease outbreak predictions enable proactive treatments and preventative plans.
- Predict expected market prices and demand for certain crops when planning seasonal activities to maximize profitability.
- Determine optimal harvest times and production quantities by modeling crop maturity progress and predicting peak readiness.
- Lower costs through proactive optimization driven by analytics compared to reactionary responses to issues as they arise.
- Identify highest yielding seed varieties and beneficial growing conditions for specific fields based on predictive crop analytics.
- Predictive maintenance modeling minimizes downtime by optimizing equipment sensor monitoring and service schedules.
These use cases demonstrate the broad value proposition of applying predictive intelligence to guide better farming and agribusiness decisions.
Real-world Applications
As we mentioned in our previous blogs, such as AI in Agriculture, there are various capabilities in smart farming using machine learning:
Automated irrigation and fertilization
Smart farming techniques employ machine learning to analyze soil moisture levels, weather forecasts, and crop data to automate irrigation and fertilization processes. This ensures that plants receive the right amount of water and nutrients at the right time, optimizing resource usage and crop growth.
Soil analysis and nutrient management
Machine learning algorithms can process soil data to identify nutrient deficiencies, pH imbalances, and other soil-related issues. This enables farmers to implement targeted soil amendments and fertilization strategies, promoting healthier crops and reducing ecological impact.
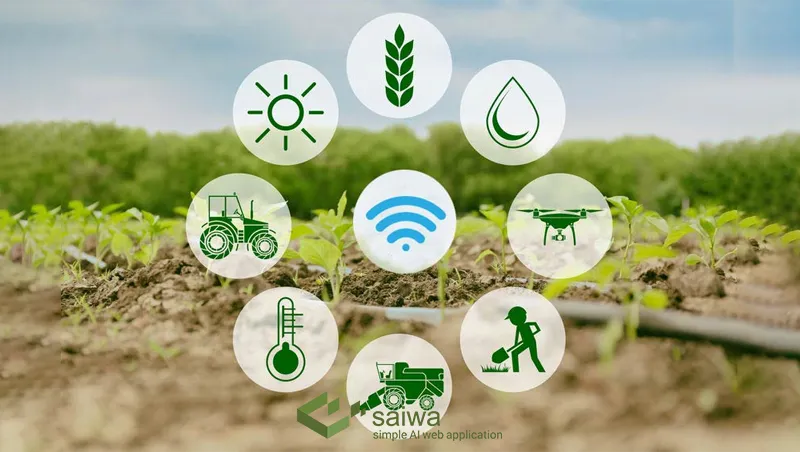
Indoor cultivation and controlled environments
Vertical farming involves growing crops in vertically stacked layers, often in indoor environments. Machine learning algorithms play a key role in monitoring and controlling essential variables such as light intensity, temperature, humidity, and nutrient delivery. This precise control enables year-round cultivation, increased crop yield, and reduced resource consumption.
Optimization of light, temperature, and humidity
Machine learning algorithms can analyze real-time data from sensors to adjust lighting conditions, temperature, and humidity levels in vertical farm setups. By maintaining optimal growing conditions, farmers can achieve faster growth rates, better crop quality, and higher yields.
Benefits of Smart Farming Using Machine Learning
Several key benefits arise from applying machine learning to smart farming:
First and foremost, predictive analytics and precision management enabled by machine learning aim to boost yields and crop quality by determining optimal site-specific actions like planting densities, irrigation amounts, fertilizer inputs, and harvesting times for each location based on data. Optimized resource allocation prevents losses.
Machine learning also facilitates more efficient use of key agricultural resources like water, fertilizers, pesticides, and energy through precisely targeted application only where, when, and in the amounts needed based on data insights. This reduces unnecessary waste while sustaining productivity.
For pest and disease management, machine learning allows earlier detection based on predictive models, sometimes even before visible symptoms arise. This enables preventative treatment to avoid losses rather than reactive efforts after widespread outbreaks. Data-driven integrated pest management reduces pesticide overuse as well.
Read Also : AI in Food Technology | Revolutionizing the Way We Eat
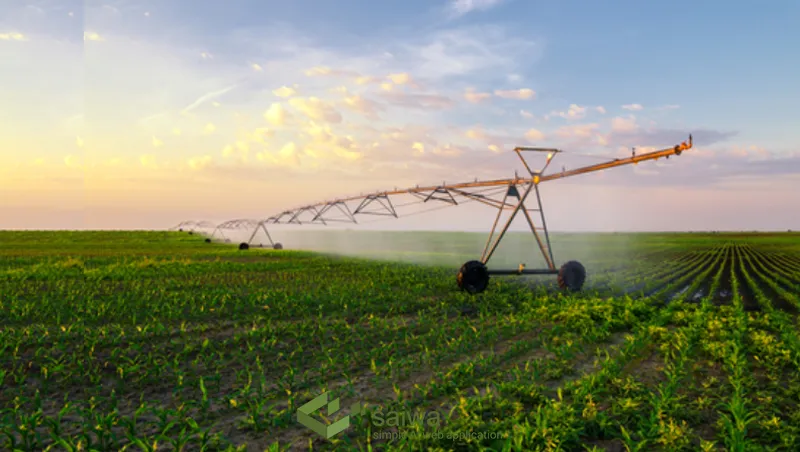
Data Sources to Feed Predictive Analytics in Smart Farming Models
A variety of data sources feed into predictive analytics in smart farming systems for the agricultural industry:
- Historical and real-time weather data provides temperature, precipitation, soil moisture data to forecast crop cycles.
- Soil sensors monitor nitrogen levels, salinity, moisture, and other attributes to model crop needs.
- Satellite imagery detects vegetation patterns, helping identify crop stress and growth variability.
- Detailed crop yield records allow modeling correlations between yields and field conditions over time.
- Equipment sensors tracking runtime, fuel usage, engine load can optimize future machinery usage.
- Local market and commodity pricing data enables crop planning tailored for profitability.
- Government agriculture statistics contain useful benchmarks for comparison.
The more relevant data from across operations tapped as inputs, the greater the predictive insights generated.
Challenges and Limitations:
However, issues like the following must be addressed in smart farming using machine learning:
Data privacy and security concerns
The collection and integration of large amounts of sensitive farm data raise concerns about data privacy and security. Proper data encryption, access controls, and compliance with privacy regulations are necessary to address these challenges.
Initial setup and integration challenges
Setting up a smart farming system can be complex and requires adequate infrastructure, such as IoT devices, sensors, and reliable connectivity. Additionally, integrating diverse technologies and ensuring compatibility between different systems can present initial challenges. Technical constraints like low connectivity in remote areas, power for devices, and latency should not exclude smallholder farmers from machine learning potential; hence, human-centric development is key.
Read Also: Greenhouse IOT
Cost implications for small-scale farmers
Implementing smart farming practices can involve significant upfront costs, such as purchasing IoT devices and implementing sensor networks. Small-scale farmers with limited resources may face financial barriers to adopting these technologies.
Conclusion
Machine learning holds tremendous potential to accelerate a new era of data-driven, digitally enhanced precision agriculture if purposefully applied. However, inclusively addressing accessibility, ethics, and responsible technology integration remains paramount. If developed collaboratively with farmers, smart farming using machine learning could become a cornerstone for sustainably nourishing the world.