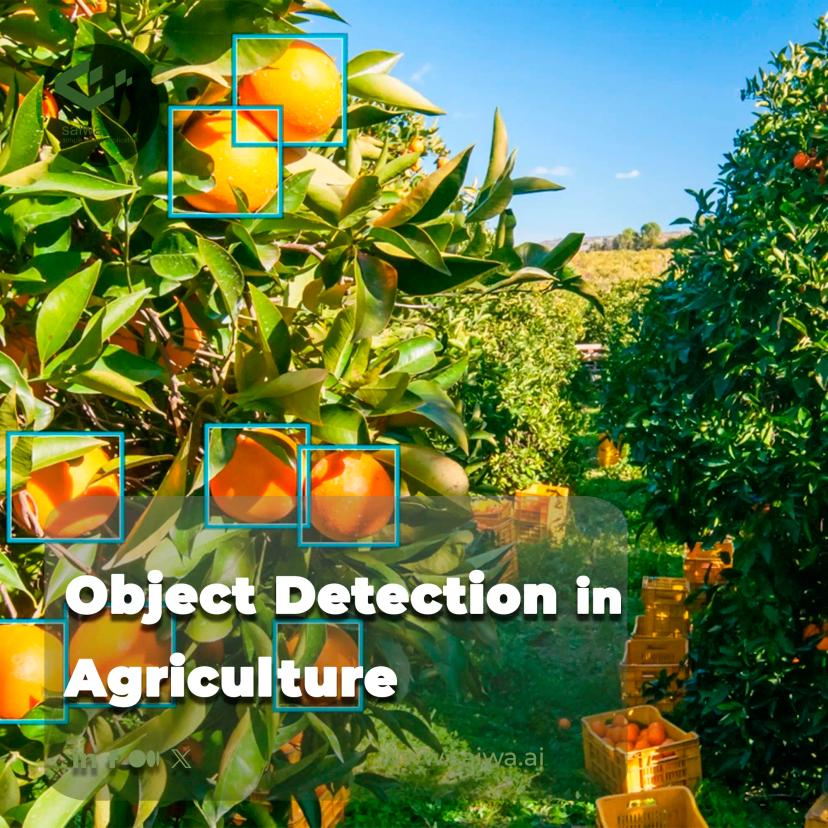
Object Detection in Agriculture
In the ever-evolving landscape of modern agriculture, the integration of cutting-edge technologies has become paramount for ensuring efficient and sustainable practices. Among these innovations, object detection stands as a pivotal tool, revolutionizing the way we approach various aspects of crop management, pest control, and yield optimization. By harnessing the power of advanced computer vision and machine learning algorithms, object detection in agriculture has emerged as a game-changer, enabling farmers and agricultural experts to make informed decisions and implement data-driven strategies.
As the global population continues to grow, the demand for food production increases, necessitating the adoption of innovative solutions that can maximize yields while minimizing resource consumption and environmental impact. Object detection in agriculture offers a powerful solution to address these challenges, providing real-time monitoring, analysis, and decision-making capabilities that were once unimaginable. In this blog post of Saiwa, we will examine the emerging innovations that are pushing the boundaries of what is possible, unlocking new frontiers in sustainable and efficient agricultural practices.
Read Also: Practical Application & Future of AI in Agriculture
Basics of Object Detection
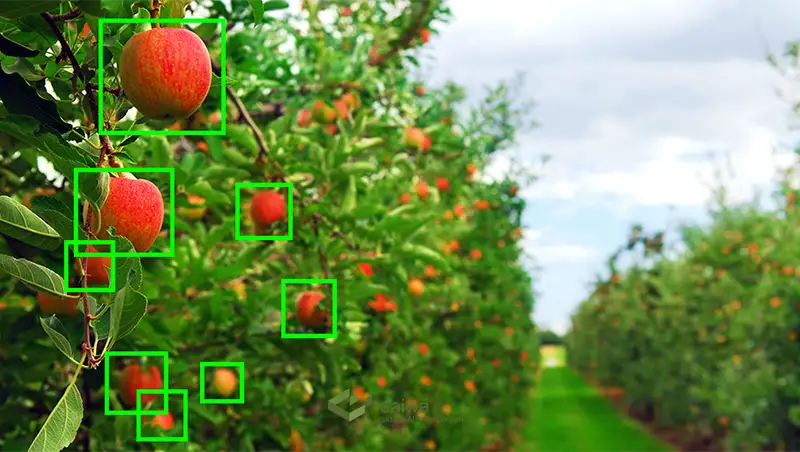
Object detection is a branch of computer vision that focuses on identifying and localizing specific objects within digital images or video streams. In the context of agriculture, this technology is employed to detect and classify various entities, such as crops, pests, weeds, and livestock, among others. By leveraging advanced algorithms and machine learning models, object detection systems can accurately identify and locate these objects, enabling farmers and agricultural experts to make informed decisions and implement targeted interventions.
Object detection in agriculture goes beyond mere recognition; it also provides valuable insights into the characteristics and attributes of the detected objects. This includes information such as the size, location, and quantity of crops, the presence and severity of pest infestations, and the distribution of weeds across a field. By providing this comprehensive data, object detection empowers farmers to optimize their resources, minimize crop losses, and maximize yields.
Key Components
Object detection systems in agriculture typically comprise several key components that work in tandem to deliver accurate and reliable results. These components include:
Image or Video Acquisition: High-quality images or video streams are captured using specialized cameras, drones, or satellite imagery. These visual inputs serve as the foundation for the object detection process.
Data Preprocessing: The acquired visual data undergoes preprocessing steps, such as noise reduction, image enhancement, and normalization, to ensure optimal performance of the object detection algorithms.
Object Detection Algorithms: At the core of the system are the object detection algorithms, which are responsible for identifying and localizing the objects of interest within the visual data. These algorithms leverage machine learning techniques, such as deep learning and convolutional neural networks, to achieve highly accurate results.
Training Data: Object detection systems rely on extensive training data, which consists of labeled images or videos containing the objects of interest. This training data is used to teach the algorithms to recognize and classify the desired objects accurately.
Post-processing and Analysis: Once the objects have been detected, post-processing techniques are applied to refine the results and extract relevant information, such as object counts, locations, and attributes. This data is then analyzed to provide actionable insights and recommendations for agricultural practices.
User Interface: User-friendly interfaces, such as web applications or mobile apps, are designed to present object detection results and insights in an accessible and intuitive manner, enabling farmers and agricultural experts to make informed decisions efficiently.
Algorithms and Techniques in Object Detection
Object detection in agriculture leverages a wide range of algorithms and techniques to achieve accurate and reliable results. These algorithms are constantly evolving, driven by advancements in machine learning, computer vision, and computational power. Some of the prominent algorithms and techniques employed in object detection for agricultural applications include:
Convolutional Neural Networks (CNNs)
CNNs are a type of deep learning architecture that has proven exceptionally effective in object detection tasks. These algorithms are designed to automatically learn and extract features from visual data, enabling accurate object recognition and localization. Popular CNN architectures used in agriculture include YOLO (You Only Look Once), Faster R-CNN, and Mask R-CNN.
Region-Based Convolutional Neural Networks (R-CNNs)
R-CNNs are a class of algorithms that combine region proposal methods with CNNs for object detection. These algorithms first identify regions of interest within an image and then apply CNN-based classification to identify the objects within those regions. Examples include Fast R-CNN and Faster R-CNN.
Semantic Segmentation
Semantic segmentation algorithms go beyond simple object detection by classifying and labeling every pixel in an image, assigning it to a specific object or category. This technique is particularly useful in agriculture for tasks such as crop monitoring, weed detection, and soil analysis.
Instance Segmentation
Instance segmentation is an extension of semantic segmentation, where individual instances of objects are identified and segmented separately. This technique is valuable for applications such as counting and tracking individual plants, fruits, or livestock.
Transfer Learning
Transfer learning is a technique that leverages pre-trained models on large datasets and fine-tunes them for specific tasks or domains. This approach has proven effective in agriculture, as it allows researchers and developers to take advantage of existing models trained on general object detection tasks and adapt them to the agricultural domain with relatively small amounts of labeled data.
Data Augmentation
Data augmentation techniques are employed to artificially expand the training data by applying transformations, such as rotations, flips, and color adjustments, to the existing labeled images. This approach helps to improve the robustness and generalization capabilities of the object detection models, particularly in scenarios where large amounts of labeled data are not readily available.
Ensemble Methods
Ensemble methods combine multiple object detection algorithms or models to improve overall performance and accuracy. By leveraging the strengths of different approaches, ensemble methods can overcome individual model limitations and provide more reliable and consistent results.
These algorithms and techniques are constantly evolving, driven by ongoing research and development efforts in the field of computer vision and machine learning. As new breakthroughs emerge, the capabilities of object detection in agriculture will continue to expand, unlocking new possibilities for optimized crop management, pest control, and yield maximization.
Read Also: An Over View Applications of Computer Vision in Agriculture
Applications of Object Detection in Agriculture
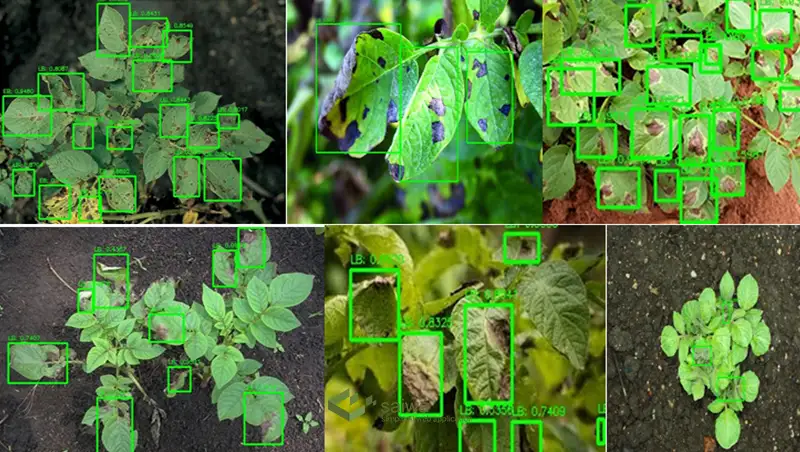
Object detection in agriculture has a wide range of applications, each playing a crucial role in optimizing various aspects of crop management, pest control, and yield optimization. These applications span multiple domains within the agricultural sector, demonstrating the versatility and far-reaching impact of this technology. Some of the key applications include:
Crop Monitoring and Yield Estimation
Object detection systems can accurately identify and count individual crops, enabling precise monitoring of crop growth and development. This information is invaluable for yield estimation, allowing farmers to make informed decisions regarding resource allocation, harvest planning, and marketing strategies.
Pest and Disease Detection
Early detection of pests and diseases is critical for minimizing crop losses and implementing effective control measures. Object detection algorithms can identify and localize various pests, such as insects, fungi, and pathogens, enabling timely interventions and targeted treatments.
Weed Identification and Management
Weeds can significantly impact crop yields by competing for nutrients, water, and sunlight. Object detection systems can accurately identify and map the distribution of weeds within fields, facilitating targeted herbicide application or mechanical removal, and reducing the need for widespread chemical treatments.
Emerging Innovations
The field of object detection in agriculture is rapidly evolving, driven by advancements in machine learning, computer vision, and related technologies. Researchers and innovators are continuously pushing the boundaries, exploring novel approaches, and integrating complementary technologies to enhance the capabilities and applications of object detection in agricultural settings. Some of the emerging innovations in this domain include:
Hyperspectral and Multispectral Imaging
Traditional object detection relies on visible light imagery, but the integration of hyperspectral and multispectral imaging techniques can provide additional valuable information. These techniques capture data across a wide range of the electromagnetic spectrum, including visible, infrared, and ultraviolet wavelengths. This additional spectral information can reveal insights into plant health, nutrient deficiencies, and soil characteristics, enabling more comprehensive analysis and decision-making.
Aerial and Satellite-Based Object Detection
The use of aerial and satellite-based platforms for object detection offers a broader perspective and larger coverage area compared to ground-based systems. AI Drones equipped with advanced cameras and sensors can capture high-resolution imagery and data over vast agricultural fields, while satellite imagery can provide valuable insights at a regional or global scale. These technologies enable large-scale monitoring, mapping, and analysis of crop conditions, pest infestations, and environmental factors.
Conclusion
Object detection in agriculture has emerged as a transformative technology, revolutionizing the way we approach crop management, pest control, and yield optimization. By leveraging advanced computer vision and machine learning algorithms, this technology empowers farmers and agricultural experts with unprecedented insights and decision-making capabilities. In conclusion, object detection in agriculture represents a transformative force, poised to reshape the way we approach food production and agricultural practices. By leveraging the power of advanced algorithms and emerging innovations, we can unlock new frontiers in sustainable and efficient agriculture, contributing to a more secure and resilient global food system for generations to come.