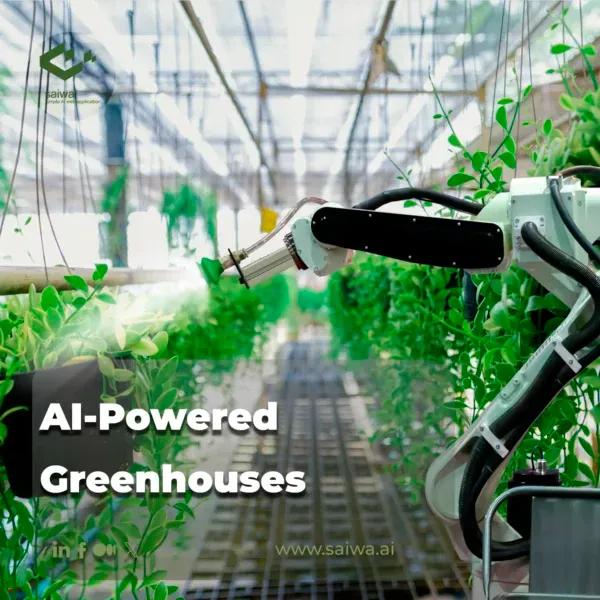
Benefits and Challenges of Ai-Powered Greenhouses
Greenhouses allow growers to optimize conditions for plant growth and maximize yields. As sensor systems, automation technologies, and AI techniques advance, “smart greenhouses” are emerging that leverage data-driven intelligent systems for monitoring, control, and decision support. AI-powered greenhouses promise to transform greenhouse management by increasing efficiency, quality, and sustainability.
Read Also: Leveraging Smart Sensors and Next-Generation Greenhouse IOT Management
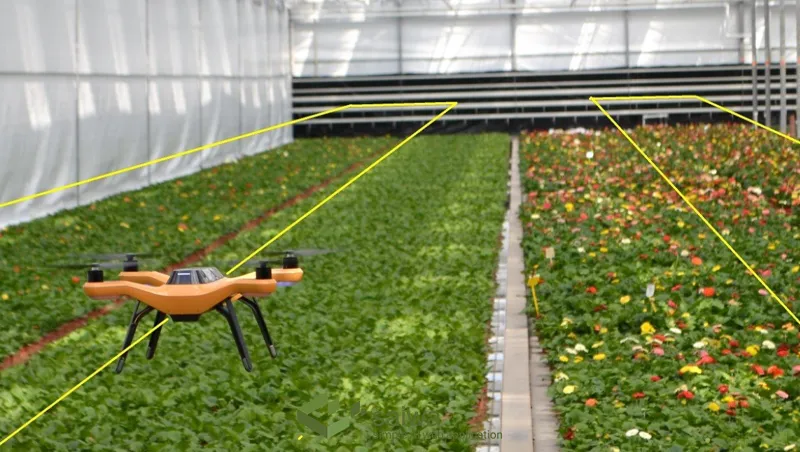
Computer Vision Monitoring and Analytics
Camera networks provide continuous, detailed crop monitoring to track growth and identify problems early. Time-series analysis of image-derived plant morphological metrics such as stem width, leaf size, and coloration provide insight into health status and developmental stages, enabling prediction of yield, harvest timing, and market windows.
Multispectral and hyperspectral cameras detect subtle spectroscopic anomalies that signal the onset of disease and pest infestations before physical manifestations occur. This allows for early, targeted treatment when infections are still localized, shifting to proactive management. However, robust detection requires training over multiple appearance variants within a greenhouse.
With rich temporal visual data, deep learning techniques such as LSTMs can learn plant growth patterns to identify anomalies and make yield predictions. However, model overfitting remains a challenge. Simulation environments that generate synthetic training data help overcome the lack of real labeled images. Overall, computer vision provides rich analytical insights from visual greenhouse data streams.
AI-Powered Greenhouses and Climate Control
Maintaining optimal temperature, humidity, ventilation, lighting, and other environmental parameters is critical to plant health and growth. Physics-based AI models can simulate greenhouse climate dynamics to predict conditions and optimize control interventions. Reinforcement learning agents improve set points to balance a conducive growth environment with energy efficiency.
With multiple interdependent variables, AI-based climate control outperforms traditional rule-based controllers. AI dynamically adjusts setpoints based on predictions informed by weather data, crop needs, and real-time sensor streams. Automated ventilation, shade control, and other actuators enable responsive management of the indoor environment. This maintains ideal growing environments for quality and yield.
Intelligent Hydroponics Management
Monitoring and adaptive control of nutrients and growth media is critical in hydroponic greenhouses. Online sensors continuously track pH, dissolved oxygen, electrical conductivity, and other parameters. Outlier detection and classification algorithms help identify events such as low oxygen levels before they escalate.
Physics-based models predict optimal nutrient inputs tailored to plant types and growth stages based on current root development and climatic conditions. Control systems then dispense the necessary nutrient solutions and minerals. Monitoring systems analyze sensor streams to diagnose problems and improve control logic. This optimizes growth media for health and quality.
Automated aeration, filtration, and dosing maintain ideal conditions, minimizing the risk of environmental shocks from suboptimal media. Cybersecurity is critical, however, as compromised control systems could deliver hazardous chemical doses. Anomaly detection and human override capabilities provide safeguards against malfunction or malicious manipulation.
Robotics and Automation
Robotic arms assist human growers with repetitive and physically intensive tasks such as seeding, transplanting, pruning, and harvesting. Path planning algorithms avoid dense foliage and obstacles for efficient navigation in confined greenhouse layouts. Manipulation capabilities enable gentle, damage-free plant handling.
Automated mobile inspection robots also complement the static camera infrastructure. LiDAR, thermal sensors, and computer vision support the monitoring of plant health, soil conditions, and equipment issues across greenhouse zones. Learning-based navigation avoids disruptions to operations.
Despite high upfront costs, long-term benefits include reduced reliance on scarce skilled labor, improved consistency, and 24/7 task automation. Intuitive programming interfaces could improve accessibility even for small greenhouses. However, user-centered design and training are essential for a smooth adoption.
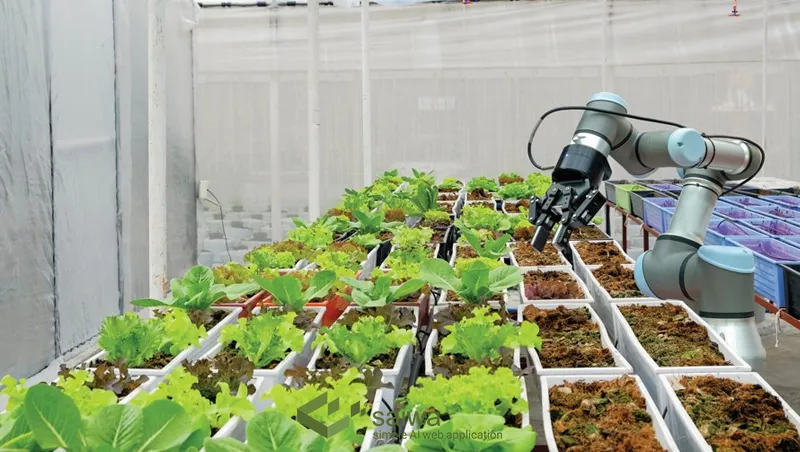
Decision Support Systems
To build trust and adoption, interfaces must provide straightforward visualizations, alerts, and prescriptive recommendations based on model output. This decision support can recommend responses to emerging crop risks, such as disease, that are informed by local factors without requiring fully autonomous action. Ongoing human oversight will remain critical.
Participatory design that incorporates grower feedback will maximize usability and relevance. Growers will be able to annotate images, validate predictions, and customize decision-support content. This grounds AI in user needs and context. Compliance logging also enables oversight for safety. AI aims to augment human growers, not replace them, by surfacing non-obvious insights from data.
Benefits of AI-Powered Greenhouses
AI-Powered Greenhouses can achieve higher yields and crop quality by optimizing growing conditions based on continuous monitoring data and predictive analytics. Computer vision enables early detection of disease before it spreads, allowing localized suppression and loss prevention. Physics-based models precisely tune temperature, humidity, nutrients, and other factors to the needs of each crop, maximizing plant health. Automation also reduces resource use and waste through targeted, precision application of lighting, water, and chemicals, rather than across the entire greenhouse.
In addition, robotics and intelligent systems automate routine physical tasks such as seeding, harvesting, and monitoring. This reduces reliance on scarce skilled labor, improves consistency, and enables 24/7 operation without fatigue. AI also provides greater resilience to risks such as equipment failure or changing weather by quickly detecting anomalies and prescribing corrective actions. Overall, AI-Powered Greenhouses increase productivity, efficiency, and sustainability.
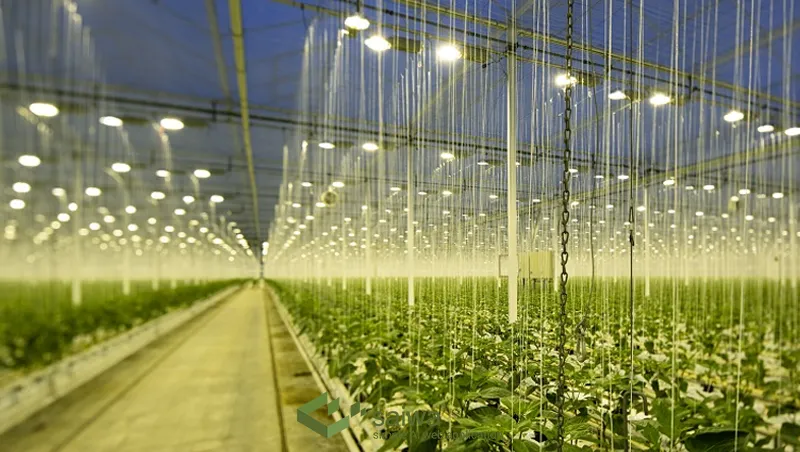
Challenges of Adoption
However, significant barriers can limit adoption. Initial infrastructure investments in sensors, data pipelines, and analytics platforms raise costs, especially for small farms with limited capital. The complexity of data-driven systems also requires specialized skills. Obtaining the large, high-quality, labeled datasets needed to train robust AI models remains challenging. Without participatory development, systems risk being impractical or incompatible with existing practices and user needs.
Cultural resistance to new technologies persists in traditionally hands-on fields such as agriculture. Cybersecurity vulnerabilities in networked systems could allow catastrophic manipulation if compromised. There is also the risk of over-automation without maintaining farmer oversight and responsibility. To overcome these multiple challenges, costs must be reduced, capabilities must be aligned with user workflows, and trust in AI must be cultivated through transparent development and governance.
The Evolving Role of Growers
The role of the grower will evolve with the integration of AI. Growers will spend less time on manual tasks and more time on higher-level oversight, strategic analysis, and customer interactions. They will monitor and collaborate with AI systems, rather than directly perform routine operations. This evolution will require skills development and change management. But thoughtfully implemented, AI aims to augment growers' expertise and free up their time for more impactful contributions. Human context remains essential for greenhouses to balance automation with natural complexity.
Conclusion
AI is transforming greenhouse management across the entire crop lifecycle-from seeding to harvest. Intelligent systems are optimizing previously manual routines while uncovering hidden insights from data. However, collaborative development and governance frameworks are essential for the smooth integration of AI capabilities into greenhouse operations. When managed holistically, AI-Powered Greenhouses can increase productivity and sustainability to help meet the growing demand for food.