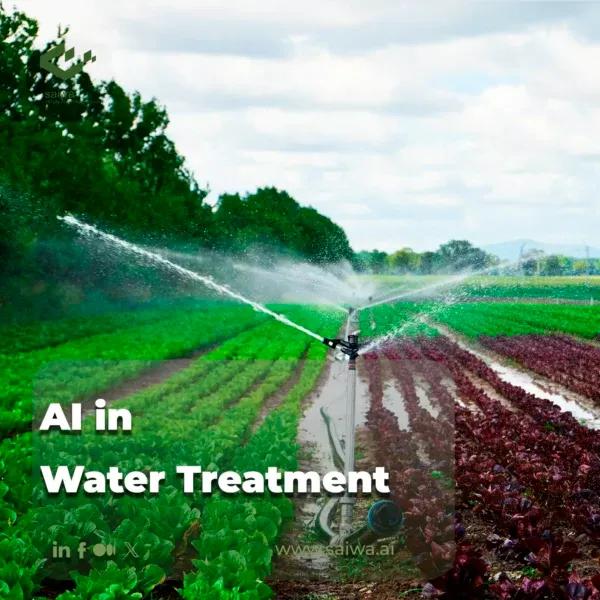
AI in water treatment | Optimizing Irrigation Efficiency
Clean water is vital for agriculture and food production. However, contaminants from natural and human sources can compromise water quality and safety. Additionally, aging infrastructure and unpredictable demand create challenges for reliably supplying water. Artificial intelligence (AI) is emerging as a transformative technology for intelligently managing water treatment and distribution in the agricultural sector.
AI in water treatment techniques like machine learning and computer vision enable real-time monitoring, predictive analytics, and process automation to optimize water usage. When thoughtfully implemented, AI-based water management can improve efficiency, reduce waste, and ensure both adequate water quantity and quality for crop irrigation and livestock.
Read Also: AI in Agriculture | Practical Application & Future of AI in Agriculture
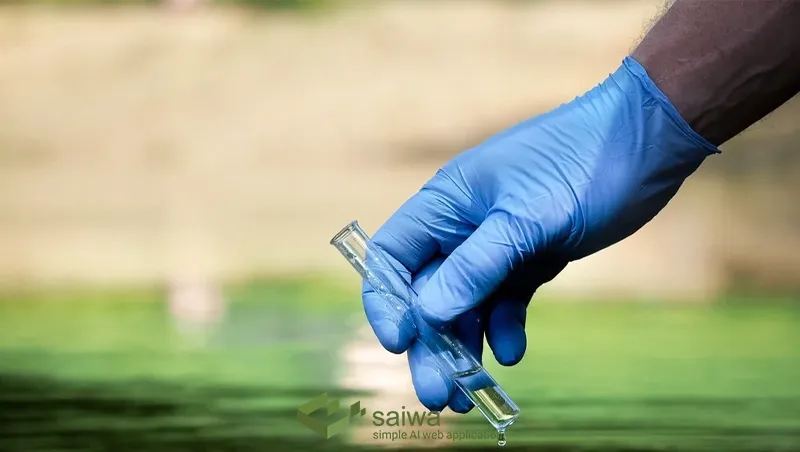
Water Contaminant Monitoring
Water contaminant monitoring is a critical process for ensuring the safety and quality of our water supply. By systematically evaluating and analyzing water samples, it helps identify and address potential contaminants to protect public health and the environment.
Continuous Sensor Surveillance
High-frequency water quality measurements enable the detection of contamination events rapidly. Online turbidity, pH, conductivity, and chlorine sensors provide important baseline indicators. Time series analysis tracks normal variability versus outliers signaling anomalies. Computer vision on microscope images also reveals particulates. Combining multiple sensors and data modalities provides robust monitoring.
Early Warning Systems
Contamination alerts require low latency for urgent response. Machine learning classifies severity based on anomaly size, while physics-based models identify expected propagation. AI-powered monitoring dashboards visually summarize threats and recommended actions for operators. Rapid contaminant detection maximizes available response time before agricultural application.
Read Also: Automated Agriculture Irrigation Systems| a Closer Look
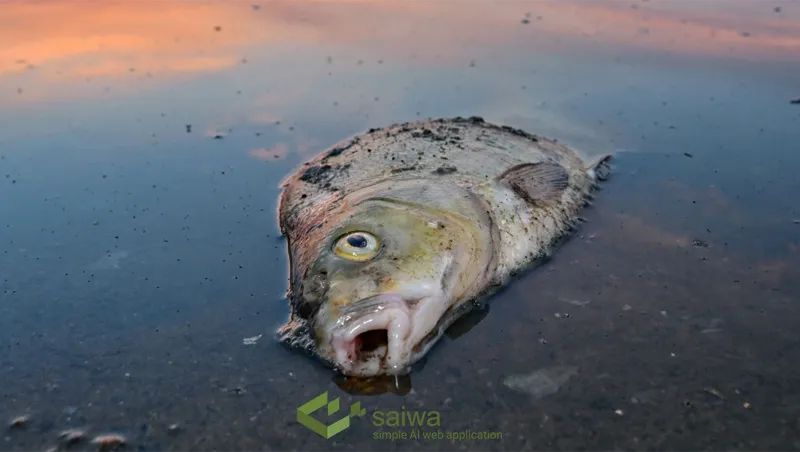
Ongoing Model Improvements
Training data limitations are a challenge for reliable AI monitoring. Online learning continuously updates models with new sensor measurements to improve baseline water profiles. Classifiers also expand to new contaminant types by incorporating expert knowledge and transferring learned features. Regular validation and explainability analysis are critical to building confidence.
Predictive Maintenance
Predictive maintenance is an innovative approach that uses data and advanced analytics to predict equipment failures before they occur. By proactively identifying problems, organizations can reduce downtime, extend asset life, and optimize maintenance schedules, ultimately saving time and resources.
Multimodal Sensor Fusion
AI predictive maintenance integrates diverse sensor data such as vibration, acoustics, temperature, pressure, power consumption, and flow rate. Combining time series streams provides a multi-dimensional view of equipment health. Anomalies in any modality can indicate developing failures. Correlating across measurements improves the accuracy of predicting failure.
Physics-Based Failure Modeling
Expert knowledge relates sensor observations to component deterioration processes such as fatigue, corrosion, and wear. These physical models guide feature design and anomaly interpretation. Hybrid AI, combining data-driven detection with physics-based diagnostics, provides fully explainable predictions for operator confidence.
Prescriptive Maintenance Planning
Ai in water treatment model outputs should link detected anomalies to actionable maintenance prescriptions. Severity scores determine repair urgency while optimization balances lead time, resource constraints, and failure risks. Predictions must integrate smoothly into existing maintenance workflows. AI is a decision aid, not a full replacement for human judgment.
Leakage Detection
Leaking pipes result in wasted water, infrastructure damage, and contamination risks. AI algorithms help detect leaks by analyzing flow patterns and pressure sensors. Anomalous flows pinpoint the location, while machine learning classifies leak severity to prioritize critical repairs. For large utility networks, AI optimization identifies optimal leak detection routes. Rapid response to AI-detected leaks reduces losses and safety hazards.
Water Quality Modeling
Water Quality Modeling is a sophisticated approach that employs mathematical and computational techniques to simulate and predict the quality of water in various environmental systems. Analyzing the complex interplay of physical, chemical, and biological factors, provides essential insights into the health of aquatic ecosystems and the safety of water sources.
Complex Treatment Dynamics
Water treatment processes like coagulation, ozonation, adsorption, and filtration involve complex physical and chemical interactions. AI modeling incorporates domain expertise into multilayer differential equation representations capturing nonlinear dynamics. This maps process inputs to outputs under diverse operating conditions.
Operational Recommendation Systems
Ai in water treatment models enables predictive simulations to evaluate control interventions without perturbing real infrastructure. The models quantify expected effects on water quality metrics under hypothetical scenarios. This allows AI agents to recommend operational adjustments for achieving safety and compliance goals while minimizing cost and energy.
Control Automation and Optimization
Autonomous AI-driven treatment controllers tune pumps, valves, aerator speeds, etc. responsively based on streaming sensor data and quality predictions. Physics-informed constraints ensure safe operating ranges. Multi-objective optimization adjusts multiple water quality parameters simultaneously for overall plant efficiency.
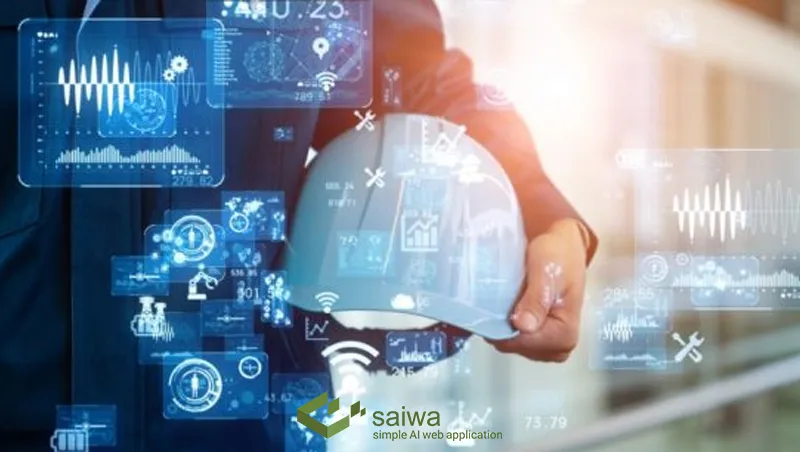
Demand Forecasting
Unpredicted spikes or drops in agricultural water demand lead to deficiencies and wastage. AI forecasting algorithms incorporate weather, crop, and seasonal trends to reliably estimate needs across timescales. This allows tuning storage, supply, and distribution accordingly. AI-assisted planning balances agricultural requirements with responsible water stewardship.
Treatment Process Control
Treatment process control is the backbone of ensuring the efficiency and effectiveness of various treatment processes in water and wastewater treatment facilities. By constantly monitoring and adjusting variables, it ensures that contaminants are removed and water quality meets stringent standards, promoting clean and safe water for communities.
Monitoring and Diagnostics
AI controllers continuously analyze sensor measurements on treatment subsystems to detect issues and anomalies. Domain-specific feature engineering provides interpretable system state representations for diagnostic assessments. Classifiers identify faults and abnormalities from historical patterns. This monitoring enables responsive maintenance.
Automatic Control Interventions
When a problem is detected, AI systems prescribe and execute corrective actions, such as adjusting coagulant dosage, aeration flow rates, or backwashing filters. This automation provides precise quality control without constant human oversight. However, AI should remain within configured safety limits and subject to occasional human review.
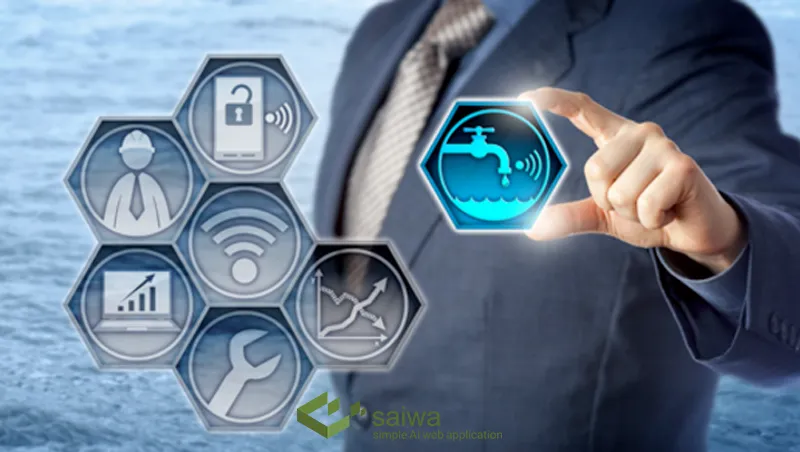
Adaptive Optimization
Beyond reactive control, AI optimization dynamically adjusts plant operations in anticipation of changing conditions. AI balances multiple objectives, such as maximizing quality and reliability while minimizing cost and energy consumption. Continuous sensor data updates the optimization as system conditions evolve. This enables efficient proactive adaptation rather than reactive operation.
Overview of the issues in water management globally
Managing water resources effectively is crucial as we face an array of worldwide challenges. Innovative approaches are essential to manage water sustainably. The promise of AI for water management is to tackle these problems head-on.
As the globe sees more people, more cities, and more industries, the strain on water supplies increases. This puts a burden on water managers to come up with smart, strategic solutions. AI for water management is key here, helping to balance water distribution, predict when and where water is needed, and cut down on waste.
The situation with water management is further complicated by the erratic climate, leading to droughts and floods. AI for water management offers a vital tool for predicting and mitigating these disasters. By deploying AI and predictive analytics, we can get ahead of water shortages or excesses and act proactively.
Old and deteriorating water systems also make things difficult, causing leaks and high maintenance costs. AI can reshape this by allowing for the ongoing tracking of water networks. Equipped with AI, smart sensors, and IoT devices enhance data gathering and pinpoint leaks sooner, boosting infrastructure strength.
Another pressing concern is water quality, endangered by pollutants. Here AI steps in to supervise and maintain water purity, ensuring it meets health standards. Using machine learning, AI systems process huge amounts of data to spot potential contamination and prevent it.
In the realm of agriculture, the challenge lies in juggling water conservation with the water needs for irrigation. Precision farming, enhanced by AI, elevates crop output while dialing back on water usage. This eco-conscious method aligns with the bigger goal of careful water management.
AI’s application in water management is revolutionary, offering a whole system to ramp up water efficiency, minimize risks, and encourage sustainable habits the world over. This blend of AI and water strategy isn’t just a tech evolution; it’s a leap toward a more robust and enduring water future.
Read Also : AI for Hydroponic Farming
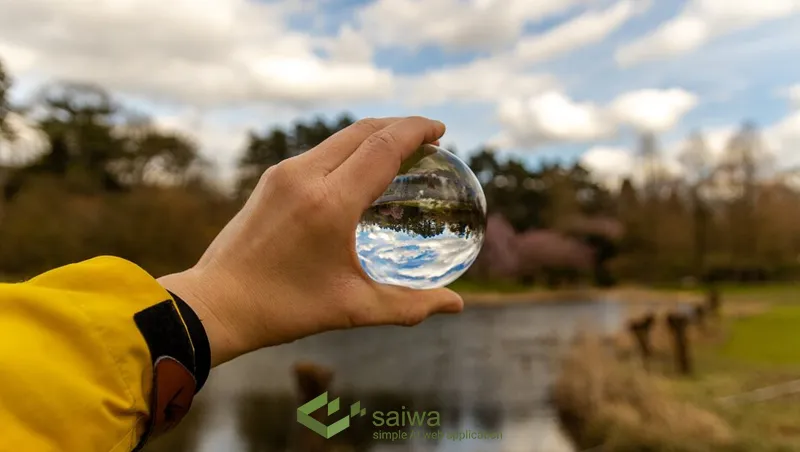
Conclusion
AI solutions in AI in water treatment field are well-suited for addressing pressing challenges in agricultural water management ranging from quality assurance to predictive maintenance. To build trust and adopt AI responsibly, water utilities must implement robust cybersecurity, preserve human oversight, and communicate transparently with stakeholders. If embraced prudently, AI-enabled intelligent water systems can help ensure safe and sustainable water usage for agricultural needs.
Read Also: AI in Agriculture | Practical Application & Future of AI in Agriculture