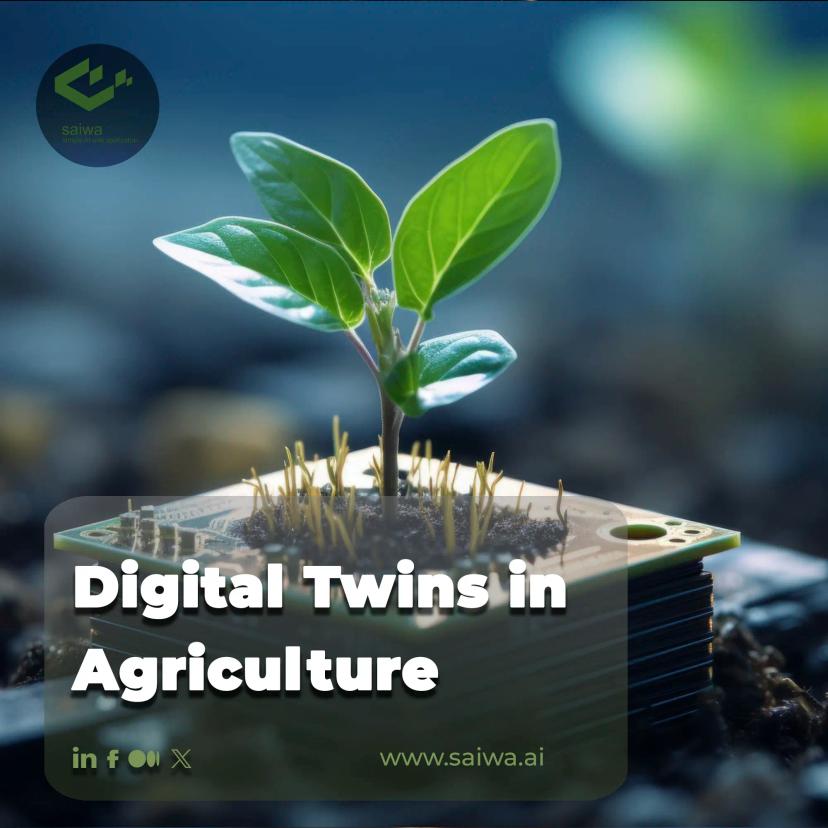
The Impact of Digital Twins in Agriculture |Revolutionizing Farming
The agricultural sector is undergoing a significant transformation driven by technological advancements. Precision agriculture, a data-driven approach to farming that utilizes information technology to optimize crop production and livestock management, is at the forefront of this change. Digital twins, virtual representations of physical entities linked through real-time data exchange, are emerging as a powerful tool within precision agriculture, offering a multitude of benefits for farmers, agricultural researchers, and the entire food production system.
Definition and Key Concepts
A digital twin is a digital representation of a physical entity or process, such as a farm, a crop field, a herd of livestock, or even an individual plant or animal. This digital representation is updated in real time with data collected from a variety of sources, including sensors, satellite imagery, and farm machinery. By simulating the behavior of the physical entity in the virtual world, digital twins enable farmers to gain deeper insights, predict potential challenges, and optimize agricultural practices for improved efficiency, sustainability, and yield.
Importance of Digital Twins in the Era of Industry 4.0
The integration of cyber-physical systems, the Internet of Things (IoT), and big data analytics, collectively known as Industry 4.0, is transforming various industries, including agriculture. Digital twins represent a pivotal technology within the context of Industry 4.0 for agriculture, offering the potential for real-time monitoring, data-driven decision-making, and the optimization of complex agricultural systems.
What are Digital Twins and How Do They Work?
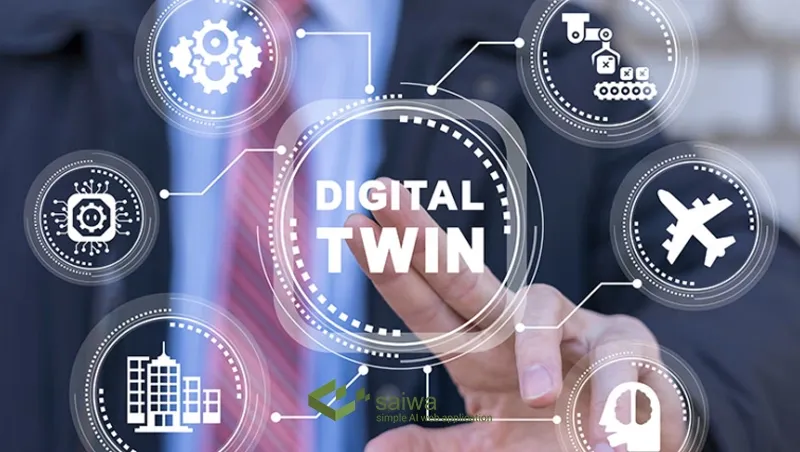
A digital twin in agriculture typically comprises the following components:
Physical Entity (farm, crop, livestock, etc.)
This refers to the real-world system or organism being modeled. Data is collected from this entity through various sensors and monitoring systems.
Virtual Model (computational representation)
This is a digital representation of the physical entity created using software and mathematical models. The virtual model incorporates data on the entity's physical characteristics, environmental conditions, and historical performance.
Data Acquisition and Integration
Sensors are deployed on farms, weather stations, satellites, and agricultural machinery to collect real-time data on various parameters. These include soil moisture, temperature, plant growth stage, animal health indicators, and farm equipment performance. Subsequently, the data is integrated into the digital twin platform for analysis.
Simulation and Analytics
The virtual model is employed in the execution of simulations designed to predict the behavior of the physical entity under a variety of conditions. In order to achieve this objective, advanced analytics techniques are employed with the intention of extracting meaningful insights from the data collected and the simulation results.
User Interfaces and Visualization
Dashboards and visualization tools present data and simulation results in a format that is accessible to users, allowing farmers and agricultural professionals to monitor key performance indicators, identify trends, and make informed decisions.
The Role of Digital Twins in Agriculture
Digital twins offer a multitude of benefits for various aspects of agricultural operations:
Improved Decision Making: By providing real-time data and insights, digital twins empower farmers to make data-driven decisions on irrigation, fertilization, pesticide application, and other critical farm management practices.
Precision Farming: Digital twins enable site-specific management, allowing farmers to tailor inputs and practices to the specific needs of different areas within a field, optimizing resource utilization and crop yield.
Enhanced Efficiency: Digital twins can automate tasks such as irrigation scheduling and resource allocation, leading to increased operational efficiency and reduced labor costs.
Risk Management: By simulating potential scenarios, digital twins can help farmers anticipate and mitigate risks associated with weather events, pests, and diseases.
Improved Sustainability: Digital twins can promote sustainable practices by optimizing water and nutrient use, minimizing environmental impact, and promoting responsible agricultural production.
Digital Twin Architecture and Components
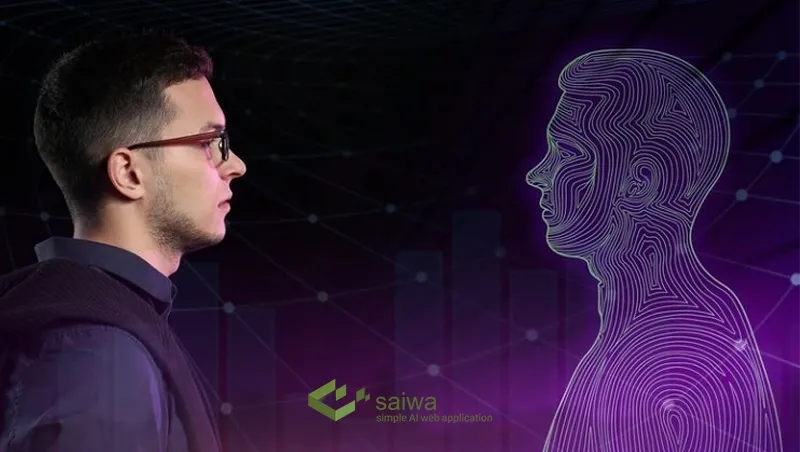
A robust digital twin architecture is crucial for effective implementation in agriculture. Here's a breakdown of the key components:
Physical Entity (farm, crop, livestock, etc.)
As mentioned earlier, this is the real-world system being modeled. The types of physical entities can range from individual plants or animals to entire farms or agricultural landscapes.
Virtual Model (computational representation)
The virtual model is a complex software representation of the physical entity. It incorporates data on various aspects, including:
Biophysical characteristics: These include soil properties, plant genetics, animal breeds, and physiological parameters.
Environmental conditions: Weather data, historical climate patterns, and real-time sensor readings on temperature, humidity, and precipitation are integrated.
Management practices: Data on past and planned agricultural practices, such as planting dates, fertilizer application rates, and irrigation schedules, is included.
Data Acquisition and Integration
A variety of data sources contribute to the digital twin:
Remote sensing (satellites, drones, UAVs): These technologies provide valuable data on crop health, soil moisture levels, and field conditions over large areas.
Internet of Things (IoT) sensors: A network of sensors deployed throughout the farm collects real-time data on various parameters, such as soil temperature, humidity, light levels, and nutrient content. Sensors can also be attached to individual plants or animals to monitor vital signs and growth patterns.
Weather and climate data: Historical and real-time weather data from weather stations and forecasting services are integrated to understand the impact of weather patterns on crop growth and animal health.
Soil data: Soil analysis reports, including data on nutrient composition, pH levels, and organic matter content, are incorporated into the model.
Crop growth models: Existing scientific models that simulate plant growth and development under different environmental conditions are used to inform the virtual model.
Farm machinery and equipment data: Data from tractors, harvesters, and other farm equipment can provide insights into field conditions, planting depth, and crop yield.
Modeling and Simulation Techniques: The virtual model leverages various modeling techniques to simulate the behavior of the physical entity:
Physics-based modeling: These models rely on scientific equations and principles to simulate physical processes like crop growth, water flow in soil, and animal metabolism.
Data-driven modeling (machine learning, deep learning): Machine learning algorithms are trained on historical data to identify patterns and relationships. These relationships can then be used to make predictions about future outcomes.
Hybrid modeling approaches: A combination of physics-based and data-driven models can be used to leverage the strengths of both approaches for more comprehensive simulations.
Uncertainty Quantification and Sensitivity Analysis: Real-world data is inherently uncertain, and models have limitations. Uncertainty quantification techniques assess the level of confidence in model predictions. Sensitivity analysis identifies how changes in specific input parameters affect the model's outputs.
High-performance computing and parallel simulations: Complex agricultural systems require high-performance computing resources to run simulations efficiently, particularly for large-scale farms or regional models. Parallel processing techniques can be employed to distribute the computational workload across multiple processors.
Data Sources for Agricultural Digital Twins
Digital twins rely on a rich tapestry of data sources to create a comprehensive virtual representation of a physical farm system. These data sources can be broadly categorized as follows:
Remote sensing (satellites, drones, UAVs)
These technologies provide valuable data on a range of agricultural parameters, including crop health, soil moisture levels, field conditions, and even plant growth patterns over large areas. Satellites offer a high-altitude perspective for monitoring vast agricultural landscapes, while drones and unmanned aerial vehicles (UAVs) can capture high-resolution imagery at lower altitudes, allowing for more detailed analysis of specific fields.
Internet of Things (IoT) sensors
A network of sensors deployed throughout the farm collects real-time data on various parameters that directly impact crop growth, animal health, and overall farm operations. These sensors can be strategically placed in soil, on plants themselves, or integrated into farm machinery. Examples of data collected by IoT sensors include:
Soil temperature, humidity, and nutrient content
Plant growth stage and health indicators
Animal vital signs like body temperature, heart rate, and activity levels
Farm machinery performance metrics like fuel consumption and field coverage
Weather and climate data
Historical and real-time weather data from weather stations and forecasting services are crucial for understanding the impact of weather patterns on crop growth, animal health, and resource management. This data can include:
Precipitation levels
Temperature and humidity fluctuations
Wind speed and direction
Historical weather trends and forecasts
Soil data
Soil analysis reports, which include data on nutrient composition, pH levels, and organic matter content, provide valuable insights into the health and fertility of the soil. This data is employed to inform decisions pertaining to fertilizer application, irrigation practices, and the implementation of comprehensive soil management strategies.
Crop growth models
The incorporation of existing scientific models that simulate plant growth and development under different environmental conditions into the digital twin represents a significant advancement in the field of agricultural engineering. These models may be based on factors such as plant genetics, soil type, and weather data, thereby enabling predictions regarding potential yields and the identification of potential issues.
Farm machinery and equipment data
The data generated by tractors, harvesters, and other farm equipment can provide valuable insights into a number of key areas, including field conditions, planting depth, crop yield, and overall farm operations. For instance, data on fuel consumption by tractors can be employed to identify variations in soil compaction across different areas of a field.
Modeling and Simulation Techniques
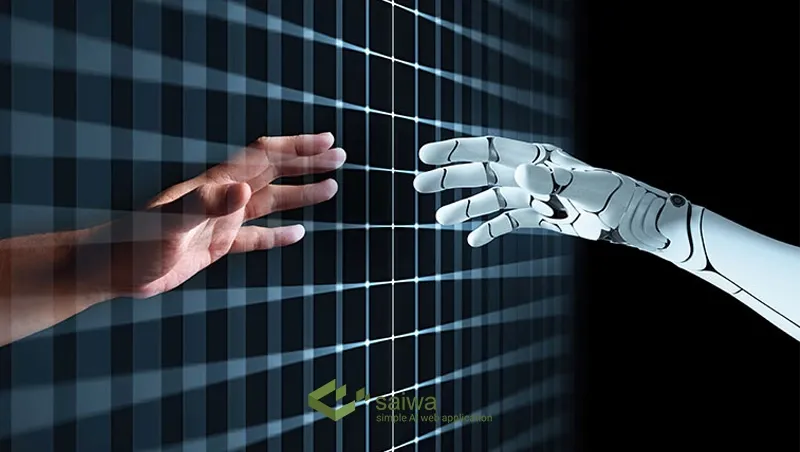
The virtual model within a digital twin leverages various modeling techniques to simulate the behavior of the physical entity:
Physics-based modeling
These models rely on scientific equations and principles to simulate physical processes like crop growth, water flow in soil, nutrient uptake by plants, and animal metabolism. They offer a high degree of accuracy but can be computationally expensive for complex systems.
Data-driven modeling (machine learning, deep learning)
Machine learning algorithms are trained on historical data from various sources with the objective of identifying patterns and relationships. These relationships can then be employed to make predictions about future outcomes, such as crop yield or potential pest outbreaks. Machine learning models are often more expeditious to execute than physics-based models, yet they may necessitate substantial data for training and may be less readily interpretable.
Data-driven modeling, which encompasses machine learning and deep learning, is the process of creating models that learn from and make predictions based on data. Through the application of machine learning and deep learning, Saiwa through its new product, i.e. Sairone, develops sophisticated models that analyze vast amounts of image data for the precise detection of crops or pests, as well as for the authentication of other objects.
The platform employs boundary annotation, bounding-box annotation, and classification annotation to generate comprehensive training datasets, which are essential for the accurate training of models. These annotations permit Saiwa's deep learning algorithms to comprehend intricate patterns and enhance their performance over time. By integrating data-driven modeling, Saiwa provides clients with advanced image analysis and detection solutions, ensuring high accuracy and reliability.
Hybrid modeling approaches
A combination of physics-based and data-driven models can be employed to capitalize on the respective strengths of both approaches. For instance, a physics-based model can serve as the fundamental framework, while a machine learning model can be employed to enhance the simulations through the incorporation of real-world data.
Uncertainty quantification and sensitivity analysis
It is an inherent limitation of real-world data that it is uncertain. Furthermore, models have limitations. Uncertainty quantification techniques assess the level of confidence in model predictions by considering the variability in input data and model assumptions. A sensitivity analysis identifies the manner in which alterations in specific input parameters (e.g., weather patterns, fertilizer application rates) affect the model's outputs. This enables farmers to comprehend the prospective consequences of disparate managerial choices.
High-performance computing and parallel simulations
Complex agricultural systems, particularly for large-scale farms or regional models, require significant computational resources to run simulations efficiently. High-performance computing clusters can provide the necessary processing power, while parallel processing techniques can distribute the computational workload across multiple processors, accelerating simulations.
By integrating these diverse data sources and employing various modeling techniques, digital twins create a comprehensive and dynamic virtual representation of a physical agricultural system. This allows farmers to gain deeper insights, predict potential challenges, and optimize agricultural practices for improved efficiency, sustainability, and yield.
Applications of Digital Twins in Agriculture
Digital twins in agriculture offer a wide range of applications across various agricultural practices:
Precision farming and site-specific management
By analyzing data on soil conditions, crop health, and historical yields, digital twins can guide farmers in applying fertilizer, irrigation, and pesticides only where and when needed, optimizing resource use and minimizing environmental impact.
Crop yield prediction and optimization
Simulations can predict potential crop yields under different scenarios, allowing farmers to adjust management practices to optimize yield and minimize losses.
Pest and disease monitoring and management
Real-time data from sensors can help detect early signs of pest infestations or disease outbreaks. Digital twins in agriculture can then be used to simulate the spread of pests or diseases and recommend targeted control measures.
Water and nutrient management
Digital twins in agriculture can optimize irrigation schedules based on real-time soil moisture data and weather forecasts, ensuring efficient water use and minimizing water waste. Nutrient management can also be optimized by analyzing soil nutrient levels and plant needs.
Livestock monitoring and management
Sensors attached to animals can transmit data on health indicators like body temperature, heart rate, and activity levels. Digital twins in agriculture can then be used to monitor animal health, detect potential illnesses early, and optimize feeding and breeding practices.
Supply chain optimization and traceability
Digital twins in agriculture can track agricultural products from farm to fork, ensuring transparency and traceability throughout the supply chain. This information can be used to improve logistics, identify potential contamination risks, and build consumer trust in food safety.
Read Also: Practical Application & Future of AI in Agriculture
Digital Twin Integration and Interoperability
For seamless integration and data exchange between different digital twin platforms and farm management systems, several factors need to be addressed:
Standardization and open data formats
Standardized data formats and protocols are crucial for ensuring compatibility between different digital twin platforms and farm management systems. This allows for seamless data exchange and integration.
Interoperability with farm management systems
Digital twins need to integrate with existing farm management software used for tasks like record keeping, financial management, and resource allocation. This ensures a holistic view of farm operations and avoids data silos.
Cloud-based platforms and edge computing
Cloud computing provides the scalability and storage capacity required for managing large amounts of data generated by digital twins in agriculture. Edge computing can be used to process time-sensitive data locally on devices or gateways before sending it to the cloud for further analysis.
Cybersecurity and data privacy considerations
Security measures are essential to protect sensitive farm data collected by digital twins in agriculture. Data privacy regulations need to be adhered to ensure:
Data security: Implement robust cybersecurity measures to safeguard data from unauthorized access, breaches, or cyberattacks. This includes encryption of data at rest and in transit, strong passwords, and regular security audits.
Data privacy: Comply with relevant data privacy regulations such as GDPR (General Data Protection Regulation) and CCPA (California Consumer Privacy Act). This may involve obtaining consent from farmers for data collection, providing transparency on how data is used, and offering farmers control over their data.
Data ownership and access control: Clearly define data ownership rights. Farmers should have control over their data and be able to access, modify, or delete their data as per regulations. Implement access control mechanisms to restrict access to authorized personnel only.
Data anonymization and pseudonymization: Consider anonymizing or pseudonymizing data whenever possible to minimize privacy risks, especially when sharing data with third-party service providers or for research purposes.
Transparency and trust: Maintain transparency with farmers about how their data is collected, used, and shared. Building trust is essential for encouraging farmer adoption of digital twins in agriculture.
Conclusion
Digital twins are transforming the agricultural landscape by providing a powerful tool for data-driven decision-making, precision farming, and sustainable agricultural practices. By overcoming challenges related to data management, model development, and user adoption, digital twins have the potential to transform agriculture, contributing to increased food production, improved resource efficiency, and a more sustainable food system. As the technology continues to evolve and integrate with emerging advancements such as quantum computing and edge AI, the future of digital twins in agriculture promises to be even more innovative and data-driven, with the potential to feed the world's growing population in a more sustainable manner.