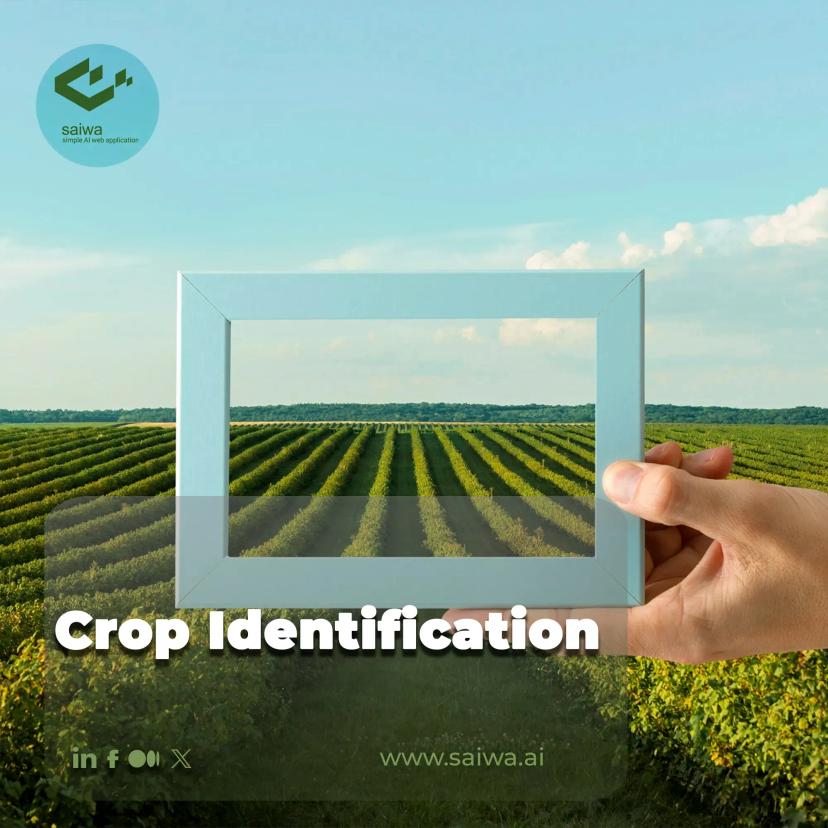
The Power of Crop Identification | Unlocking Agriculture's Potential
The capacity to accurately identify and classify crops is of paramount importance in the context of modern agriculture. Information on the types of crops grown and their spatial distribution across a field is essential for informed decision-making throughout the agricultural cycle. This information is used for a variety of purposes, including planning the planting and allocation of resources, monitoring the growth of crops, and predicting the yield. Historically, the identification of crops has been conducted through manual field surveys, a process that is both time-consuming and labor-intensive, and prone to human error. Nevertheless, the advent of remote sensing technologies and artificial intelligence (AI) has transformed the landscape of crop identification methodologies, paving the way for the development of faster, more accurate, and automated solutions. This article examines the various techniques employed for crop identification, emphasizing their respective advantages and limitations.
This article examines the significance of crop classification in agricultural practices and discusses the innovative approaches that utilize satellite imagery, aerial vehicles (UAVs), and machine learning algorithms. Furthermore, the article examines the applications of crop detection technology in weed identification, yield prediction, and resource management, emphasizing the transformative role of automation in precision agriculture.
How can crops be identified?
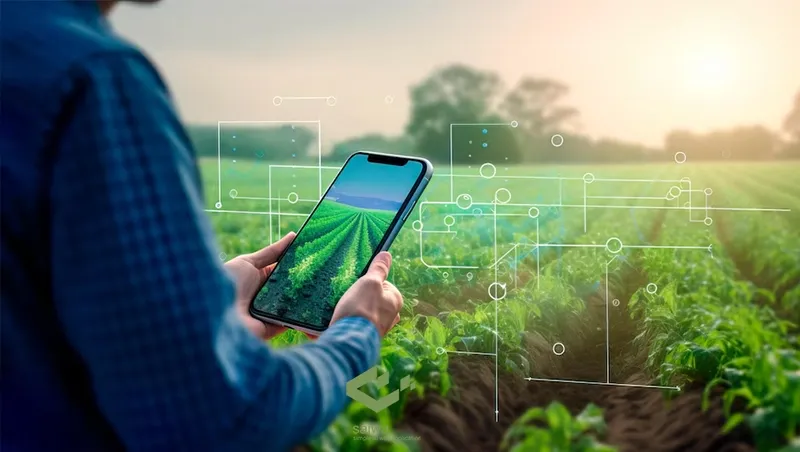
Several methods can be employed for crop identification, each with its own advantages and limitations. Here's an overview of the most common techniques:
Manual Field Surveys
This traditional approach involves visual inspection of crops in the field by trained personnel. While it offers the benefit of directly observing plant characteristics, it is a time-consuming and labor-intensive method, prone to human error and subjectivity. Additionally, manual surveys become impractical for large-scale farms or areas with difficult terrain.
Multispectral and Hyperspectral Imaging
Remote sensing techniques utilizing satellites and airborne platforms collect data beyond the visible spectrum. Multispectral imagery captures data in several discrete bands, while hyperspectral imagery acquires data across hundreds of contiguous spectral bands. The unique spectral reflectance properties of different crop types can be exploited to identify and classify them in these images. This method offers wider coverage compared to manual surveys but requires specialized equipment and expertise for data analysis.
Light Detection and Ranging (LiDAR)
LiDAR technology employs laser pulses to measure the distance between the sensor and the ground surface. This data can be used to generate detailed three-dimensional (3D) point clouds of the terrain and vegetation. Analyzing the height and structure of crops obtained through LiDAR can aid in crop identification, particularly for crops with distinct canopy structures.
Machine Learning-based Techniques
The emergence of artificial intelligence (AI) and machine learning algorithms has paved the way for automated crop identification methods. These techniques involve training algorithms on large datasets of labeled images containing various crops. The trained models can then analyze new images and identify the crops present with high accuracy. Machine learning approaches offer automation and scalability but require substantial computational resources and access to high-quality training data.
Read Also: Automated Leaf Disease Detection Using Image Processing and ML
Why is it important to classify and identify crops?
Accurate crop classification provides vital information for farmers and agricultural stakeholders, enabling them to optimize various aspects of farm management. Here are some key benefits of crop identification:
Improved Resource Management: Knowing the types and locations of crops within a field allows for targeted resource allocation. Farmers can optimize fertilizer application, irrigation practices, and pesticide use based on the specific needs of each crop type. This leads to increased efficiency, reduced waste, and improved crop health.
Yield Prediction: Accurate crop identification can be used to develop yield prediction models. By analyzing historical data on crop types, weather patterns, and other factors, farmers can gain insights into potential crop yields. This information helps in planning harvest schedules, managing storage facilities, and negotiating market prices.
Disease and Pest Management: Early detection of crop diseases and pests is crucial for minimizing yield losses. Crop identification techniques can be used to monitor crop health and identify areas with potential infestations. This allows for targeted application of pesticides and other control measures, reducing environmental impact and ensuring food safety.
Crop Insurance and Risk Management: Accurate crop classification data can be valuable for insurance companies in assessing risk and designing crop insurance policies. Additionally, farmers can utilize this information to develop risk management strategies to mitigate potential losses due to weather events or market fluctuations.
Environmental Monitoring: Crop identification data can be used to monitor land-use changes and track agricultural practices over time. This information is valuable for environmental policymakers in developing sustainable agricultural practices and promoting biodiversity.
Read Also : Improving Crop Yield Prediction Using Machine Learning
Crop Detection using the Sairone Platform
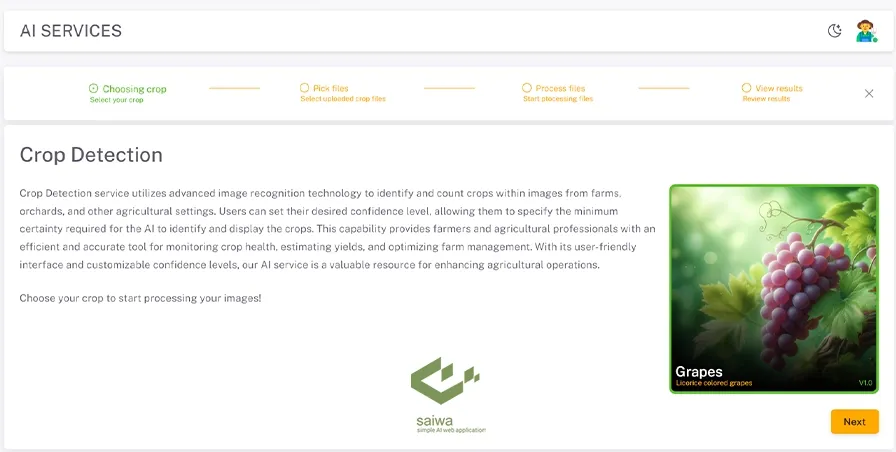
The field of crop identification has undergone a significant transformation as a result of the advent of innovative technologies such as Sairone's Crop Detection service. By employing sophisticated deep learning and machine learning algorithms, Sairone enables users to accurately identify specific crops in images and videos. The application of AI enables users to streamline the crop identification process, thereby facilitating informed decision-making throughout the agricultural cycle.
The service offers unparalleled convenience, allowing users to upload files directly to their cloud storage from a local computer or via . The platform's user-friendly interface allows users to access the platform and initiate crop identification with minimal effort. Furthermore, Sairone offers a complimentary trial period, during which users can gain firsthand experience of the service and observe its transformative capabilities.
One of the key differentiators of Sairone is its adaptability. In instances where the desired crop is not among the pre-trained options, users are afforded the flexibility to retrain the crop detection model using their own images. This customization feature ensures that the platform is tailored to the specific needs of each user, providing bespoke solutions for different agricultural applications. Furthermore, Sairone offers seamless integration with associated APIs, enabling users to automate crop identification and streamline their workflow. In any of these applications, Sairone's Crop Detection service represents a significant advance in the field of agricultural technology.
Saiwa's Sairone platform is a pioneering innovation in crop detection, offering a transformative approach to modern agriculture. By harnessing the power of Sairone, farmers can achieve greater efficiency, sustainability, and productivity. Sairone is a game-changer in crop detection, offering a glimpse into the future of agriculture. To learn more, please contact us via the Contact Us page.
Traditional Methods of Crop Identification and Their Limitations
As mentioned earlier, manual field surveys were the traditional method for crop identification. While these surveys offer the advantage of directly observing plant characteristics, they are hindered by several limitations:
Time-consuming and Labor-intensive: Manual surveys require trained personnel to physically visit fields and visually identify crops. This approach becomes impractical for large-scale farms or geographically dispersed fields.
Subjectivity and Human Error: Crop identification accuracy can be subjective and prone to human error due to variations in lighting conditions, plant growth stages, and the individual expertise of the surveyors.
Limited Scalability: Manual surveys cannot be easily scaled to cover vast agricultural regions. Monitoring large areas or tracking changes over time becomes highly challenging with this method.
Weather Dependence: Field surveys can be hampered by unfavorable weather conditions like rain or excessive heat, further limiting data collection capabilities.
The Necessity for Innovative and Efficient Crop Identification Techniques
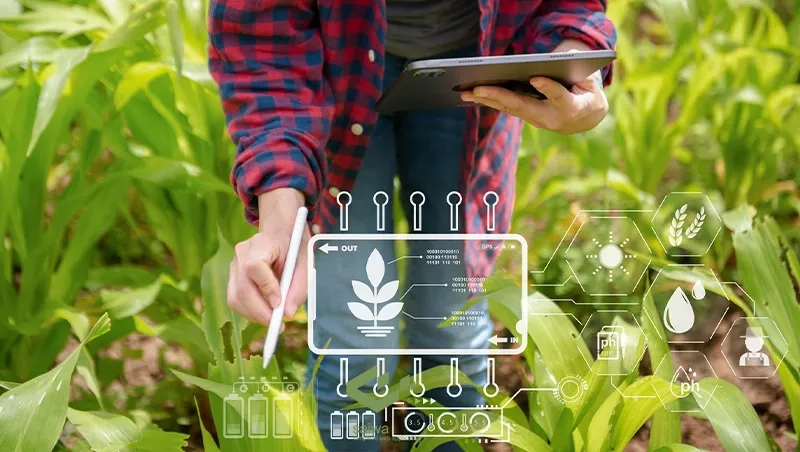
The limitations of traditional methods necessitate the adoption of innovative and efficient crop identification techniques. The increasing demand for food security, coupled with the need for sustainable agricultural practices, has driven the development of advanced technologies for crop classification.
Here's how these new approaches address the shortcomings of traditional methods:
Increased Efficiency and Scalability: Remote sensing technologies and machine learning algorithms offer a much faster and more scalable approach to crop identification. Large areas can be covered quickly and efficiently using satellite imagery or aerial data.
Reduced Human Error and Improved Accuracy: Machine learning models trained on vast datasets can achieve high accuracy in crop classification, minimizing human error and subjectivity inherent in manual surveys.
Data Availability and Repeatability: Remote sensing data provides continuous monitoring capabilities, allowing farmers to track crop growth, identify potential issues early on, and make informed decisions throughout the growing season.
Environmental Monitoring and Sustainability: Accurate crop identification data can be utilized to promote sustainable agricultural practices. By monitoring land-use patterns and optimizing resource allocation, environmental impact can be minimized, and soil health can be improved.
Read Also : Remote Sensing in Agriculture| Unleashing the Potential
What Are the Crop Types Classifications?
Crop identification can be performed based on various criteria, including:
Botanical Classification: This method categorizes crops according to their family, genus, and species. For example, wheat, barley, and oats belong to the Poaceae (grass) family.
Growth Habit: Crops can be classified based on their growth patterns, such as cereals (grasses with edible seeds), legumes (plants with pods containing seeds), and root crops (plants with edible underground storage organs).
Seasonality: Crops can be categorized as winter crops (planted in fall and harvested in spring/summer) or summer crops (planted in spring and harvested in fall/winter).
How To Classify and Manage Different Types of Crops?
Effective crop management strategies rely heavily on accurate crop identification. Here's how different crop types benefit from classification techniques:
Cereals (Wheat, Barley, Rice): Identifying crops for cereals allows for targeted application of fertilizers and herbicides specific to their needs. Furthermore, monitoring crop health can facilitate early detection of diseases like rust or smut, enabling timely intervention.
Legumes (Soybeans, Peanuts, Peas): Legume crops play a crucial role in nitrogen fixation. Identification of crops helps in optimizing nitrogen fertilizer application, promoting healthy growth while minimizing environmental impact. Additionally, it facilitates monitoring for diseases like soybean rust and powdery mildew.
Oilseed Crops (Canola, Sunflower): Accurate identification of oilseed crops enables tailored management practices. Farmers can optimize irrigation and nutrient management based on the specific requirements of each oilseed type. Additionally, monitoring helps in identifying and controlling insect pests that can significantly affect yield.
Fruits and Vegetables: Crop identification is essential for proper pruning, fertilization, and pest control practices in orchards and vegetable farms. Knowing the specific types of fruits and vegetables allows for targeted resource allocation and optimization of harvest scheduling.
Satellite Technology
Satellite remote sensing has emerged as a powerful tool for large-scale crop identification. Satellites orbit the Earth, capturing data on the reflected and emitted radiation from the Earth's surface. This data can be used to generate multispectral and hyperspectral images that reveal unique spectral signatures of different crop types.
Here's a closer look at how satellite technology is employed for crop identification:
Multispectral Imaging: Multispectral satellites capture data in several discrete bands of the electromagnetic spectrum, typically ranging from visible to near-infrared wavelengths. Different crop types reflect and absorb light at varying wavelengths. By analyzing the spectral reflectance patterns in these bands, algorithms can distinguish between different crops.
Hyperspectral Imaging: Hyperspectral satellites collect data across hundreds of contiguous spectral bands, providing a much more detailed picture of the reflected light. The rich spectral information obtained from hyperspectral imagery allows for more precise crop classification compared to multispectral data.
Challenges and Limitations of Satellite Technology
While satellite remote sensing offers significant advantages for crop identification, it also faces some challenges:
Spatial Resolution: The spatial resolution of satellite imagery refers to the level of detail captured in an image. High-resolution satellite images provide more precise information but come at a higher cost and lower revisit frequency (time between capturing images of the same area). Lower resolution images, while more frequent, may not provide sufficient detail for accurate crop classification, particularly for closely spaced crops or small fields.
Weather Dependence: Cloud cover can significantly hinder satellite data acquisition. Cloudy conditions obscure the Earth's surface, making it impossible for satellites to capture usable imagery for crop identification.
Data Processing Requirements: Analyzing satellite data for crop classification requires specialized software and expertise. Extracting meaningful information from the complex datasets necessitates computational resources and knowledge of remote sensing techniques.
Aerial Vehicles (UAVs) and drone technology
Aerial vehicles (UAVs), also commonly known as drones, offer a complementary approach to satellite remote sensing for crop identification. UAVs are unmanned aerial vehicles equipped with various sensors, including high-resolution cameras, multispectral sensors, and LiDAR systems. These sensors can be flown at lower altitudes compared to satellites, capturing data with higher spatial resolution.
Here's how UAVs contribute to crop identification:
High-Resolution Data Acquisition: UAVs can capture high-resolution imagery of agricultural fields, providing detailed information about crop health, growth stage, and potential problems. This detailed data allows for more accurate crop classification compared to lower-resolution satellite imagery.
Flexibility and Maneuverability: UAVs offer greater flexibility in data collection compared to satellites. They can be deployed on-demand to capture data over specific areas of interest and can navigate around obstacles like trees or buildings.
Multi-sensor Capability: Modern UAVs can be equipped with various sensors, including multispectral cameras and LiDAR systems. Combining data from these sensors can provide a more comprehensive picture of the crop canopy, facilitating more precise crop classification
Limitations of UAV-based Crop Identification
Despite their advantages, UAVs also have limitations to consider:
Limited Coverage Area: The operational range of UAVs is typically smaller compared to satellites. They are better suited for detailed data collection over specific fields rather than large-scale regional monitoring.
Weather Dependence: Similar to satellite data acquisition, UAV flights can be hampered by adverse weather conditions like strong winds or heavy rain.
Regulatory Restrictions: The operation of UAVs is often subject to regulations regarding airspace usage and privacy concerns. Obtaining necessary permits and adhering to regulations can add complexity to UAV-based crop identification projects.
Read More: AI-Based Drone Operation| AI in Drones use cases
The Significance of Technology and Automation in Agriculture
The integration of advanced technologies like satellite remote sensing, UAVs, and machine learning algorithms has revolutionized crop identification methods. These automated techniques offer several advantages over traditional manual surveys:
Increased Efficiency and Scalability: Remote sensing and machine learning enable rapid and efficient crop identification over large areas. Vast agricultural landscapes can be monitored with minimal human intervention, providing valuable data for informed decision-making.
Improved Accuracy and Objectivity: Machine learning models trained on vast datasets can achieve high accuracy in crop classification, minimizing human error and subjectivity inherent in manual surveys.
Data-driven Decision Making: The continuous flow of data from remote sensing and UAV platforms allows farmers to make data-driven decisions throughout the growing season. This data can be used to optimize resource allocation, identify potential problems early on, and improve overall farm management practices.
Precision Agriculture: The integration of crop identification technologies with other precision agriculture tools like variable rate technology and automated irrigation systems paves the way for more sustainable and efficient agricultural practices. Resources like fertilizers and water can be applied precisely where and when needed, minimizing waste and environmental impact.
Light Detection and Ranging (LiDAR) mapping using drones
LiDAR (Light Detection and Ranging) is a remote sensing technology that utilizes laser pulses to measure the distance between the sensor and the target object. In the context of agriculture, LiDAR systems mounted on UAVs can be used to generate detailed 3D point clouds of the terrain and vegetation. These point clouds provide valuable information about the height and structure of crops, which can be beneficial for crop identification, particularly for crops with distinct canopy structures.
Here's how LiDAR contributes to crop identification:
3D Crop Canopy Structure Analysis: LiDAR data allows for the analysis of the 3D structure of the crop canopy. This information can be used to distinguish between different crop types, especially those with varying plant heights or growth patterns. For example, LiDAR can help differentiate between corn and soybeans based on their distinct canopy structures.
Read Also: Practical Application & Future of AI in Agriculture
Conclusion
The application of remote sensing, machine learning, and computer vision to crop identification has led to a revolution in agricultural practices. The capacity to accurately classify and localize crops across extensive areas confers a multitude of advantages, including optimized resource management, enhanced yield prediction, and the early identification of crop stress. As these technologies continue to evolve and become more affordable, their adoption will further enhance precision agriculture practices, leading to increased efficiency, sustainability, and food security in a globalized world.