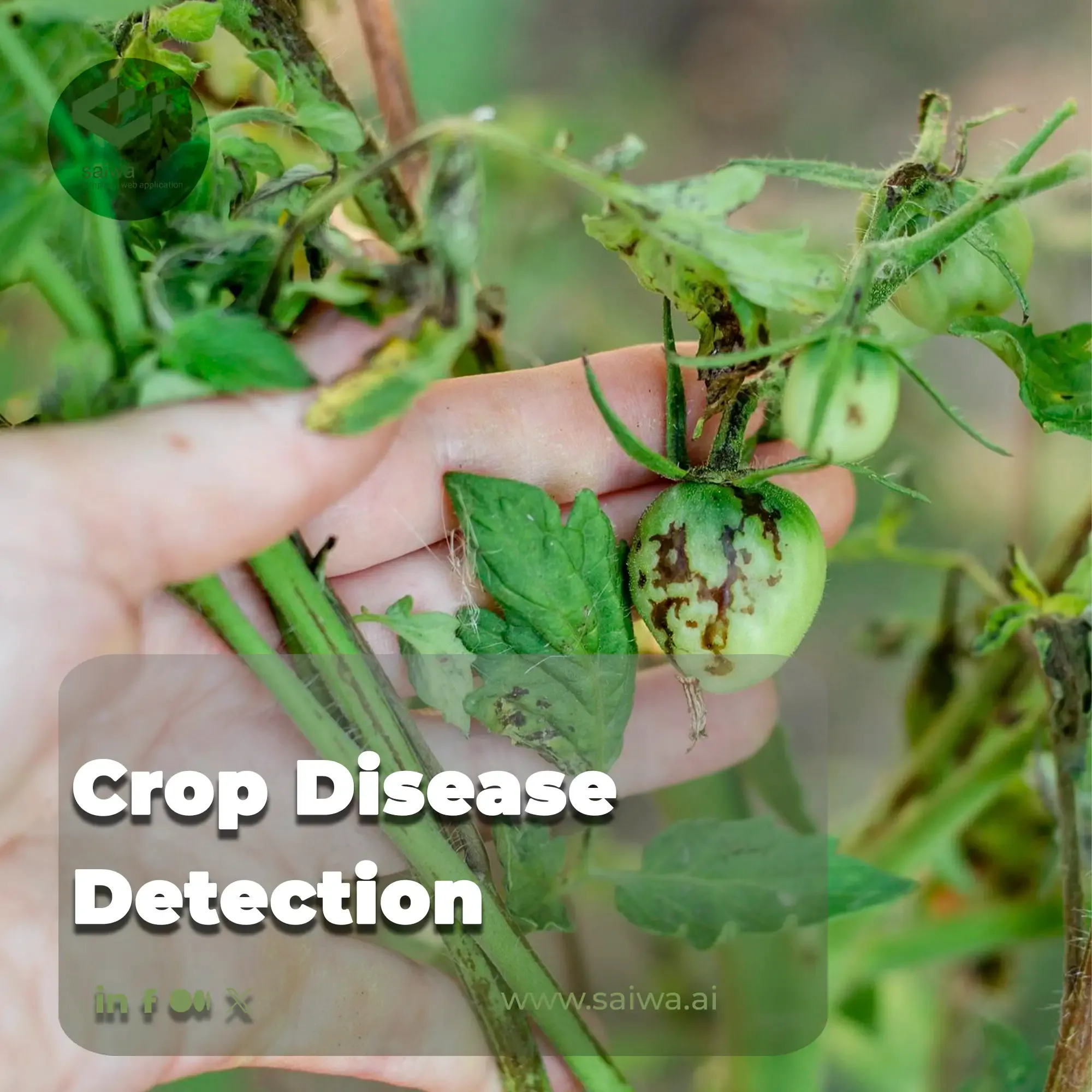
The agricultural sector plays a pivotal role in global food security. However, crop diseases pose a significant threat to crop yields, causing substantial economic losses and impacting food availability. Early and accurate detection of crop diseases is crucial for implementing effective control measures, minimizing yield losses, and ensuring food quality.
Traditional methods for disease detection, primarily relying on visual inspection by trained personnel, are often time-consuming, subjective, and labor-intensive. Advancements in computer vision, image processing, and machine learning have revolutionized crop disease detection by enabling the development of automated and objective methods.
This article explores the various approaches for crop disease detection, highlighting their principles, advantages, and limitations. We delve into the fundamentals of plant pathology, and the basis of disease classification, and discuss different sensor-based and image-based techniques employed for early disease detection.
Furthermore, the article examines the challenges and considerations associated with implementing these technologies in real-world agricultural settings. Finally, we explore the crop disease detection applications in precision agriculture, disease management strategies, and emerging trends shaping the future of this field.
Basis of Crop Disease Detection
Overview of plant pathology and disease classification
Plant pathology is the scientific discipline concerned with the study of plant diseases, their causal agents (pathogens), and the host plant response to infection. Understanding the fundamental principles of plant pathology is essential for developing effective disease detection and control strategies. Plant diseases can be broadly classified based on the type of pathogen involved:
Fungal diseases: Caused by fungi, which are eukaryotic organisms that live on or within plants and obtain nutrients from the host. Examples include powdery mildew, rust, and blight.
Bacterial diseases: Caused by bacteria, single-celled prokaryotic organisms that can rapidly multiply within plant tissues. Examples include fire blight and bacterial wilt.
Viral diseases: Caused by viruses, which are microscopic infectious agents that replicate inside host plant cells. Examples include cucumber mosaic virus and tomato spotted wilt virus.
Nematode diseases: Caused by roundworms (nematodes) that feed on plant roots, disrupting nutrient uptake and hindering growth.
Abiotic disorders: Although not caused by living pathogens, these disorders can mimic disease symptoms and are triggered by environmental factors like nutrient deficiencies, herbicide damage, or extreme weather conditions.
Read More: Plant Disease Identification | Leveraging Technology for Early Detection and Effective Management
Common crop diseases and their symptoms
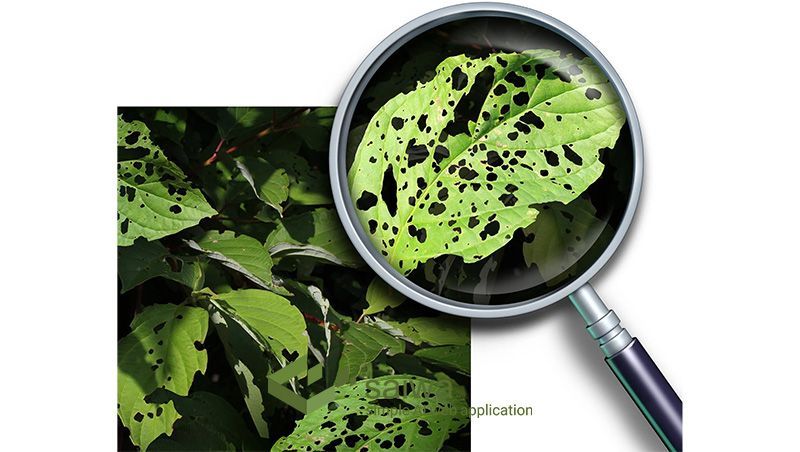
Each crop is susceptible to a specific range of diseases. Here are some prevalent crop diseases and their characteristic symptoms:
Wheat: Rust (yellow, brown, or black pustules on leaves and stems), powdery mildew (white powdery coating on leaves), and Septoria leaf blotch (brown or tan spots with dark margins on leaves).
Corn: Northern corn leaf blight (large, elongated lesions on leaves), Gray leaf spot (oval-shaped lesions with gray centers and brown margins on leaves), and Maize dwarf mosaic virus (stunting, yellowing, and malformation of leaves).
Soybeans: Soybean rust (orange or brown pustules on the undersides of leaves), bacterial blight (water-soaked lesions on stems and leaves), and Soybean mosaic virus (mottling, stunting, and distortion of leaves).
Fruits and Vegetables: Tomato late blight (water-soaked lesions on leaves, stems, and fruit), powdery mildew of grapes (white powdery coating on leaves and fruit), and bacterial spot of pepper (small, water-soaked lesions on leaves and fruit).
Early detection of these diseases is crucial for implementing control measures like fungicides, bactericides, or resistant crop varieties, minimizing potential yield losses.
Factors influencing disease development and spread
A complex interplay of factors influences the development and spread of crop diseases:
Susceptibility of the host plant: Certain plant varieties may be more susceptible to specific diseases due to genetic factors or physiological weaknesses.
Presence of the pathogen: The pathogen must be present in the environment to initiate an infection. Wind, rain, insects, or contaminated equipment can spread spores, bacteria, or viruses.
Favorable environmental conditions: Environmental factors like temperature, humidity, and rainfall can significantly influence disease development and spread. For example, high humidity and warm temperatures often favor fungal growth.
Understanding these factors allows for the development of preventative measures and the implementation of integrated pest management (IPM) strategies to minimize disease risks.
Image-based Crop Disease Detection
Image-based crop disease detection utilizes digital images or videos captured from various sources, such as ground-based sensors, UAVs, or satellites, to identify and diagnose diseases in crops. This approach offers several advantages over traditional methods:
Objectivity and Automation: Image-based detection eliminates the subjectivity associated with visual inspection, providing objective and automated disease identification.
Early Detection: Diseases can be detected at early stages when symptoms may be subtle or invisible to the naked eye, allowing for timely intervention and minimizing potential yield losses.
Scalability and Efficiency: Image-based techniques can be applied to large areas efficiently, covering vast agricultural fields in a shorter time than manual scouting.
Data Acquisition and Monitoring: Images can be captured regularly, enabling continuous monitoring of crop identification health and disease progression over time.
Read Also: Plant Disease Detection Using Image Processing |Transforming Agriculture
Principles of computer vision and image processing
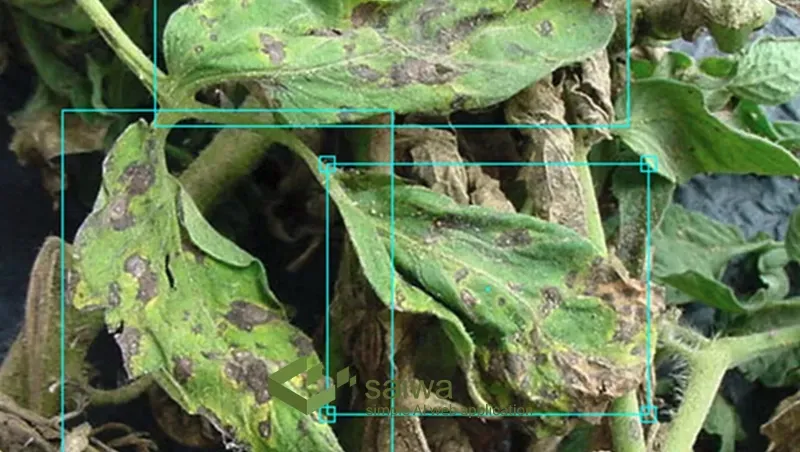
Image-based crop disease detection relies on computer vision and image processing techniques. Computer vision involves extracting meaningful information from digital images and videos. Image processing in agriculture is used to manipulate and enhance image data for further analysis. Here's a breakdown of the key steps involved:
Image Acquisition: Images of crops are captured using various platforms like ground-based sensors mounted on tractors or handheld devices, unmanned aerial vehicles (UAVs) equipped with high-resolution cameras, or satellite imagery.
Preprocessing: The acquired images may undergo preprocessing steps to enhance their quality and prepare them for further analysis. This may involve noise reduction, contrast enhancement, color correction, or background removal.
Image Segmentation: This process involves segmenting the image to separate the regions of interest (ROIs) containing the crop from the background and other objects. Segmentation techniques like thresholding, edge detection, or region-growing algorithms can be employed.
Feature Extraction: Once the ROIs are identified, relevant features are extracted from the image to characterize the potential disease presence. These features may include color features (e.g., color intensity, hue, and saturation), texture features (e.g., smoothness, roughness, and regularity), and spatial features (e.g., shape, size, and distribution of lesions).
Disease Classification: Extracted features are fed into machine learning or deep learning models trained to classify healthy and diseased plant tissues. These models learn to identify patterns and relationships between features and disease presence based on labeled training datasets containing images of healthy and diseased crops.
Read Also : An Overview Applications of Computer Vision in Agriculture
Machine learning and deep learning approaches for disease classification
Machine learning algorithms like support vector machines (SVMs), decision trees, and random forests can be used for disease classification. These algorithms learn from labeled training data to establish relationships between extracted features and disease presence. Deep learning approaches, specifically convolutional neural networks (CNNs), have emerged as powerful tools for image-based disease detection. CNNs are artificial neural networks with a layered architecture specifically designed for image recognition tasks. They learn to automatically extract relevant features from images through a series of convolutional layers and can achieve high accuracy in disease classification, especially when trained on large datasets.
Read Also : The Impact of Machine Learning in Agriculture
Crop Disease Detection using Sairone
Several AI-based platforms, such as Sairone, employ image-based disease detection for precision agriculture or Automated farming . The Sairone platform typically provides users with a web interface or mobile application for uploading images of crops or specifying the area of interest in a field.
The Sairon Crop Disease Detection platform employs pre-trained machine learning models or deep learning algorithms to analyze the uploaded images or satellite data of the designated area. The user then receives a disease classification report or map that highlights potential disease presence and affected areas. The Sairon platform offers user-friendly interfaces that can be valuable tools for farmers seeking a convenient and objective method for crop disease detection.
Read Also: Practical Application & Future of AI in Agriculture
Sensor-based Crop Disease Detection
While image-based techniques offer valuable insights, they may be limited by factors like weather conditions or lighting variations. Sensor-based crop disease detection utilizes various sensors to detect physiological changes in plants indicative of disease presence. These sensors can be deployed in fields to provide continuous monitoring and early detection of potential problems.
Overview of sensors and sensor networks
A wide range of sensors can be employed for crop disease detection, each offering unique advantages and limitations. Some commonly used sensors include:
Optical sensors: These sensors measure the reflectance properties of plant tissues in various spectral bands, including visible, near-infrared, and hyperspectral regions. Changes in spectral reflectance due to disease can be indicative of specific diseases or stress conditions.
Electrochemical sensors: These sensors measure electrical properties of plant tissues, such as pH, conductivity, and ion concentration. Variations in these parameters can indicate the presence of pathogens or disrupted physiological processes.
Thermal sensors: These sensors measure the temperature of plant tissues. Certain diseases can cause localized changes in plant temperature, which can be detected by thermal sensors.
Sensor networks can be deployed in fields, consisting of multiple sensors strategically placed to collect data from various locations. This data can be transmitted wirelessly to a central processing unit for analysis and disease detection.
Optical Sensors for Disease Detection
Optical sensors play a prominent role in sensor-based crop disease detection due to their ability to measure the spectral reflectance properties of plant tissues. Healthy and diseased plants exhibit distinct spectral signatures, allowing for the identification of potential disease presence. Here's a deeper dive into how optical sensors are utilized for this purpose:
Spectral Reflectance and Disease Detection: Plants interact with light across the electromagnetic spectrum. Healthy plant tissues absorb specific wavelengths of light for photosynthesis, while reflecting others. Disease infection disrupts these physiological processes, altering the spectral reflectance profile of the plant. Optical sensors can measure this reflected light across various wavelengths, providing valuable information for disease detection.
Multispectral Imaging: Multispectral sensors capture images in multiple discrete spectral bands, typically focusing on wavelengths relevant to plant health assessment. These sensors can be ground-based, mounted on tractors or handheld devices, or airborne, integrated into UAVs or satellites. By analyzing the variations in reflectance across different bands, multispectral imaging can detect subtle changes indicative of specific diseases.
Hyperspectral Imaging: Hyperspectral sensors capture data across hundreds of contiguous narrow spectral bands, providing a much more detailed picture of the spectral reflectance profile compared to multispectral sensors. This high spectral resolution allows for more precise disease identification and differentiation between various disease types or stress conditions. However, hyperspectral imaging systems tend to be more expensive and complex compared to multispectral sensors.
Fluorescence Spectroscopy: Certain plant pigments, such as chlorophyll, exhibit fluorescence when excited by specific wavelengths of light. Disease infection can alter the fluorescence properties of these pigments. Fluorescence spectroscopy measures the emitted fluorescence intensity at different wavelengths, providing additional insights into plant health and potential disease presence.
Data Fusion and Multimodal Disease Detection
The integration of data from multiple sensors can enhance the accuracy and robustness of crop disease detection. Data fusion techniques combine information from optical sensors (e.g., multispectral or hyperspectral) with data from other sensor types like electrochemical or thermal sensors. This combined data analysis can provide a more comprehensive picture of plant health and improve crop disease detection capabilities.
For example, combining multispectral data with thermal data can be beneficial for differentiating between fungal diseases and water stress, as both can cause similar visible symptoms. By analyzing both spectral reflectance patterns and temperature variations, the system can achieve a more accurate diagnosis.
Crop-Specific Disease Detection Techniques
The necessity of adapting disease detection techniques to the specific characteristics of crops is vital to ensure the precision and efficacy of the results obtained. Different crops face unique challenges, and customized approaches can significantly improve the outcomes of any crop disease detection project.
Understanding Crop Pathology: Each crop has a unique set of vulnerabilities. For example, wheat is prone to rust diseases, while citrus crops are often affected by greening. A crop disease detection project should begin with a thorough understanding of the target crop’s common diseases.
Leveraging Targeted Imaging Tools: Advanced imaging technologies such as multispectral and hyperspectral cameras can detect diseases specific to particular crops. For instance, these tools are effective in identifying early signs of blight in potatoes or mildew in grapevines.
Developing Crop-Specific Algorithms: Artificial intelligence models trained on disease images of a specific crop yield better detection accuracy. A crop disease detection project that focuses on rice will require algorithms tailored to identify sheath blight or bacterial leaf blight.
Field Testing for Region-Specific Variants: Diseases can vary by region. Including localized disease variants in a crop disease detection project ensures that the system is comprehensive and adaptable.
Tailoring methods to crop-specific needs not only improves detection rates but also reduces unnecessary interventions, saving time and resources. As agriculture evolves, crop-specific approaches will remain at the forefront of disease management innovation.
Challenges and Considerations
While sensor-based and image-based techniques offer significant advantages for crop disease detection, several challenges need to be addressed:
Environmental factors and field conditions
Variations in lighting conditions, weather (e.g., rain, wind), and soil moisture content can affect sensor readings and image quality. Algorithms need to be robust enough to account for these variations and minimize false positives or negatives.
Disease complexity and variability
Plant diseases can exhibit diverse symptoms depending on the specific pathogen, plant variety, and environmental conditions. Developing detection models that can generalize well to various disease manifestations requires large and diverse training datasets.
Data quality and preprocessing requirements
Sensor data and images may require extensive preprocessing to remove noise, correct for lighting variations, and ensure data quality for accurate analysis. Robust algorithms and efficient data processing methods are crucial for reliable crop disease detection.
Real-time performance and computational constraints
For real-time crop disease detection and field applications, algorithms need to be computationally efficient and provide timely results. This may necessitate balancing accuracy with processing speed, particularly for resource-constrained devices used in remote agricultural settings.
Applications and Case Studies
Crop disease detection technologies are revolutionizing precision agriculture practices by enabling:
Precision spraying and targeted disease control
Early and accurate disease detection allows for targeted application of fungicides or other control measures only to affected areas, minimizing waste and environmental impact.
Improved disease forecasting and risk assessment
Sensor data and historical information can be used to develop disease forecasting models, allowing farmers to take preventative measures and minimize potential outbreaks.
Enhanced crop monitoring and yield optimization
Continuous monitoring of crop health with sensor networks facilitates timely intervention and management practices that optimize yield potential.
Early Detection of Fungal Diseases in Grapes
A vineyard implemented a sensor-based disease detection system utilizing multispectral imaging and data analysis algorithms. The system continuously monitored grapevines throughout the growing season, detecting early signs of fungal diseases like powdery mildew and botrytis bunch rot. By enabling early intervention with fungicide application, the system significantly reduced crop losses and improved grape quality.
Cost Benefit Analysis of Different Crop Disease Detection Methods
When selecting crop disease detection methods, it is crucial to compare the costs against the benefits to ensure the most efficient approach for farmers. Traditional methods, such as visual inspections, are cost-effective but often limited by labor intensity, subjectivity, and slower response times. On the other side, modern techniques like image-based and sensor-based crop disease detection offer faster and more accurate results but come with higher upfront costs for equipment, training, and maintenance.
Cost Considerations
Image-based crop disease detection, which uses drones, satellites, or ground-based sensors, often requires significant initial investment. These costs include purchasing high-resolution cameras, drones, and software for image processing. Similarly, sensor-based methods may involve deploying networks of optical, thermal, or electrochemical sensors, which can be expensive but provide continuous monitoring. Additionally, there are costs associated with data processing and analysis, particularly when advanced machine learning algorithms are employed.
Benefits and Returns
Despite higher costs, modern crop disease detection or even leaf Disease Detection Using Image processing methods can significantly reduce losses by enabling early and precise interventions. Automated systems improve detection accuracy, reduce labor costs, and allow for targeted applications of pesticides or other treatments, minimizing waste and environmental impact. These benefits can lead to higher crop yields and better overall farm management. Selecting the most appropriate crop disease detection method involves understanding these trade-offs and the potential for long-term returns.
Economic Impact Analysis of Agriculture Detection Technologies
The integration of advanced agriculture detection technologies is transforming agricultural practices and driving notable economic transformations. Here’s an analysis of their impact:
Optimized Resource Utilization: Precision agriculture detection technologies help farmers allocate water, fertilizers, and pesticides more effectively. This results in cost savings, the minimization of environmental harm, and an increase in the profitability of farming operations.
Increased Productivity: With real-time data from agriculture detection systems, farmers can manage their crops more effectively, leading to increased yields and improved produce quality. Higher productivity levels support the ability to offer competitive pricing and ensure a consistent market supply.
Access to New Markets: The ability to produce higher-quality crops using agriculture detection opens doors to premium and export markets. This diversification of revenue streams enhances farmers' financial resilience and global trade participation.
Cost of Technology: Although implementing crop disease detection technologies requires a significant upfront investment, the long-term savings and increased profitability often far outweigh these costs, ensuring sustained financial benefits for farmers.
Job Creation and Skill Development: The rise of agriculture detection technologies has resulted in the creation of employment opportunities in the fields of technology development, installation, and training. This contributes to economic development, particularly in rural areas, through the fostering of a skilled workforce and the enhancement of livelihoods.
Reduced Environmental Costs: Crop disease detection enhances the efficient of resource utilization and reduces waste, lowering the overall environmental cost of farming. This approach not only offers benefits for ecosystems but also aligns with the global demand for sustainable agricultural methods.
Explainable AI (XAI) in Crop Disease Detection
Explainable AI (XAI) is rapidly becoming essential in crop disease detection process. While AI models can achieve impressive accuracy, understanding why they make certain predictions is important for building trust and ensuring effective deployment. XAI addresses this need by providing insights into the decision-making processes of these complex systems.
Building Trust in Crop Disease Detection: Farmers and agricultural professionals need to trust the diagnoses provided by AI tools. XAI helps build this trust by revealing the factors that contribute to a diagnosis. Instead of a "black box" approach, XAI makes the reasoning transparent.
Improving Diagnostic Accuracy: XAI can help identify potential flaws or biases in crop disease detection models. By understanding which features the model focuses on, researchers can refine the training data and improve the accuracy and robustness of the system. For example, if the model is misclassifying a disease due to a specific image artifact, XAI can highlight this issue.
Facilitating Early Intervention: In crop disease detection, early intervention is critical. XAI can help pinpoint the specific symptoms or patterns that indicate the early stages of a disease, allowing for timely treatment and preventing widespread outbreaks. This granular level of insight is invaluable.
Empowering Agricultural Professionals: XAI empowers agricultural professionals with a deeper understanding of crop diseases. By visualizing the features that the AI model uses for diagnosis, experts can gain new insights into disease progression and develop more effective management strategies. This knowledge transfer is a key benefit of XAI in crop disease detection.
Transfer Learning for Crop Disease Detection
Transfer learning (TL) has emerged as a powerful and essential technique in crop disease detection, offering a practical solution to several challenges faced in agricultural settings. It leverages pre-existing knowledge gained from other tasks to improve the efficiency and accuracy of crop disease identification, particularly when dealing with the constraints of real-world farming.
Data Scarcity Solution: Transfer learning overcomes the common problem of limited labeled data for training. By using models pre-trained on large datasets, the need for massive, crop-specific datasets is significantly reduced.
Faster Training: Instead of starting from scratch with random initialization, TL begins with weights already learned from a related task. This greatly speeds up the training process and improves performance, particularly when identifying subtle disease symptoms.
Computational Efficiency: TL models generally require fewer computational resources than traditional approaches and are better at combating overfitting.
Feature Extraction: Pre-trained Convolutional Neural Networks (CNNs) are powerful feature extractors. Various architectures can be utilized effectively.
Fine-Tuning: Pre-trained models are fine-tuned on the specific crop disease detection dataset. This involves adjusting the weights of some layers, while earlier layers are often kept frozen to preserve general feature recognition.
Ensemble Models: Combining multiple pre-trained models in an ensemble can further enhance performance and robustness.
Classifier Replacement: A common strategy is to replace the final classification layers of pre-trained CNNs with a different classifier, like logistic regression, for improved customization.
Smart Agriculture Application: TL directly aids timely and accurate plant pest and disease detection, a crucial element of smart and sustainable agriculture. It offers a faster, more cost-effective alternative to traditional methods. The overall aim is to boost automated systems for improved crop disease detection.
Trends and Emerging Technologies
The field of crop disease detection is constantly evolving, with several emerging trends shaping the future:
Integration with artificial intelligence (AI) and machine learning: Advancements in AI and machine learning are leading to the development of more sophisticated algorithms for disease detection. Deep learning techniques trained on vast datasets are improving the
accuracy and robustness of crop disease detection models: These models can learn complex relationships between sensor data, image features, and disease presence, leading to more reliable identification of diverse crop diseases.
Advancements in sensor technology: New sensor technologies are emerging, offering enhanced capabilities for crop disease detection. Hyperspectral sensors with improved resolution and miniaturized sensor designs suitable for integration into UAVs hold significant promise. Additionally, advancements in biosensors that detect specific disease markers or volatile organic compounds emitted by stressed plants are being explored.
Integration with Internet of Things (IoT) and cloud computing: The integration of sensor networks with IoT platforms allows for real-time data collection, transmission, and analysis. Cloud computing provides the necessary infrastructure for storing vast amounts of sensor data and running complex crop disease detection algorithms. This facilitates remote monitoring of crop health and timely decision-making for farmers.
Development of autonomous agricultural robots: The integration of crop disease detection technologies with autonomous robots is a promising future direction. Robots equipped with sensors and AI-powered disease identification capabilities can autonomously patrol fields, detect disease outbreaks, and even apply targeted treatments, minimizing human intervention and labor costs.
Focus on sustainable and organic agriculture: As the demand for sustainable and organic agricultural practices increases, crop disease detection technologies play a crucial role. By enabling early detection and targeted interventions, these technologies can help reduce reliance on chemical pesticides and promote more environmentally friendly crop production methods.
Conclusion
Crop disease detection technologies offer a transformative approach to ensuring food security and sustainability in agriculture. Image-based and sensor-based techniques, coupled with advancements in machine learning and AI, empower farmers with the tools to detect diseases early, optimize resource allocation, and minimize yield losses. As these technologies continue to evolve and become more cost-effective, their widespread adoption in agricultural practices holds immense potential for enhancing crop health, improving food quality, and promoting sustainable agricultural production methods that safeguard our future food security.